Label-free SERS detection of prostate cancer based on multi-layer perceptron surrogate model method
SPECTROCHIMICA ACTA PART A-MOLECULAR AND BIOMOLECULAR SPECTROSCOPY(2024)
Abstract
Prior surface-enhanced Raman spectroscopy (SERS) research has shown that pre-processing is necessary before analysis. Pre-processing also typically serves the dual purposes of removing the auto-fluorescence background and minimizing data volatility. This method allows for a more accurate comparison of spectral traits and relative SERS peak strength. However, because there are so many different kinds of samples, it can take a long time, and there is no assurance that the approach chosen will work well with a particular kind of sample. Therefore, this study employed a deep learning technique called multi-layer perceptron (MLP) to simplify the pre-processing of blood plasma SERS samples in patients with prostate cancer (PC), as well as to enhance the sensitivity and specificity of diagnosis using SERS technology. First of all, significant variations in peak intensity can be observed in the difference spectra, facilitating differentiation between PC and normal groups. Second, the data analysis was carried out in three different stages (raw data, defluorescenced data, and normalized data) using principal component analysis and linear discriminant analysis (PCA-LDA), as well as PCA-multi-layer perceptron (PCAMLP). Finally, when SERS data was analyzed using PCA-LDA, there were significant differences in classification accuracy across each stage (The classification accuracy of three different stages were 76.90%, 85.60%, 95.20%, respectively). However, when PCA-MLP was utilized for SERS data analysis, the classification accuracy remained consistently high and stable (The classification accuracy of three different stages were 92.00%, 92.40%, 96.70%,
MoreTranslated text
Key words
SERS,Prostate cancer,Blood plasma,Deep learning,Pre-processing
AI Read Science
Must-Reading Tree
Example
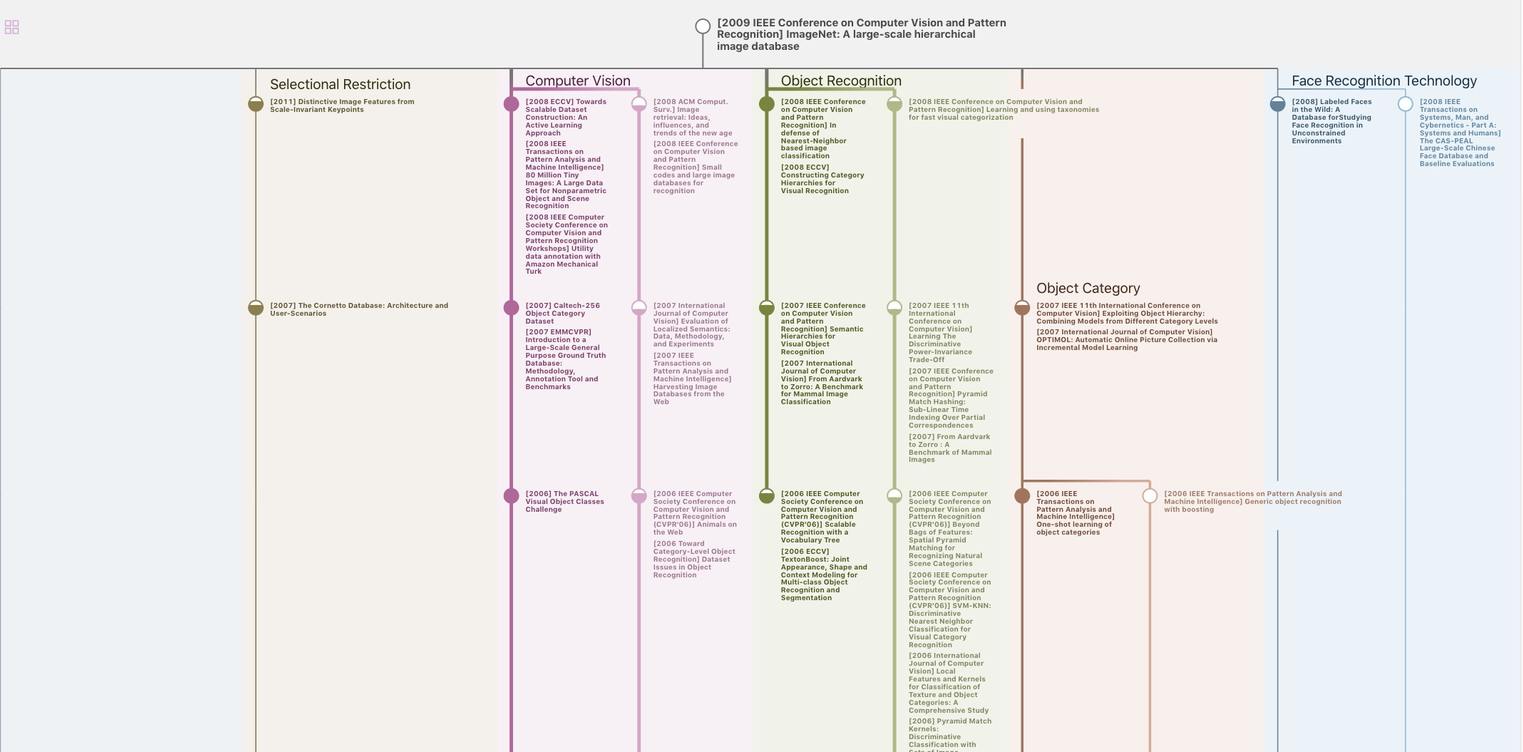
Generate MRT to find the research sequence of this paper
Chat Paper
Summary is being generated by the instructions you defined