Multi-turn dialogue comprehension from a topic-aware perspective
Neurocomputing(2024)
摘要
Dialogue Machine Reading Comprehension requires language models to effectively decouple and model multi-turn dialogue passages. As a dialogue development goes after the intentions of participants, its topic may not remain constant throughout the whole passage. Hence, it is non-trivial to detect and leverage the topic shift in dialogue modeling. Topic modeling, although has been widely studied in plain text, deserves far more utilization in dialogue reading comprehension. This paper proposes to model multi-turn dialogues from a topic-aware perspective. This paper starts with a dialogue segmentation algorithm to split a dialogue passage into topic-concentrated fragments in an unsupervised way. Then these fragments are used as topic-aware language processing units in further dialogue comprehension. On one hand, the split segments indict specific topics rather than mixed intentions, thus showing convenience on in-domain topic detection and location. For this task, this paper designs a clustering system with a self-training auto-encoder, and two constructed datasets are built for evaluation. On the other hand, the split segments are an appropriate element of multi-turn dialogue response selection. For this purpose, this paper further presents a novel model, Topic-Aware Dual-Attention Matching (TADAM) Network, which takes topic segments as processing elements and matches response candidates with a dual cross-attention. Empirical studies on three public benchmarks show great improvements over baselines. Our work continues the previous studies on document topic, and brings the dialogue modeling to a novel topic-aware perspective with exhaustive experiments and analyses.
更多查看译文
关键词
Multi-turn dialogue modeling,Topic-aware,Segmentation,Clustering,Response selection
AI 理解论文
溯源树
样例
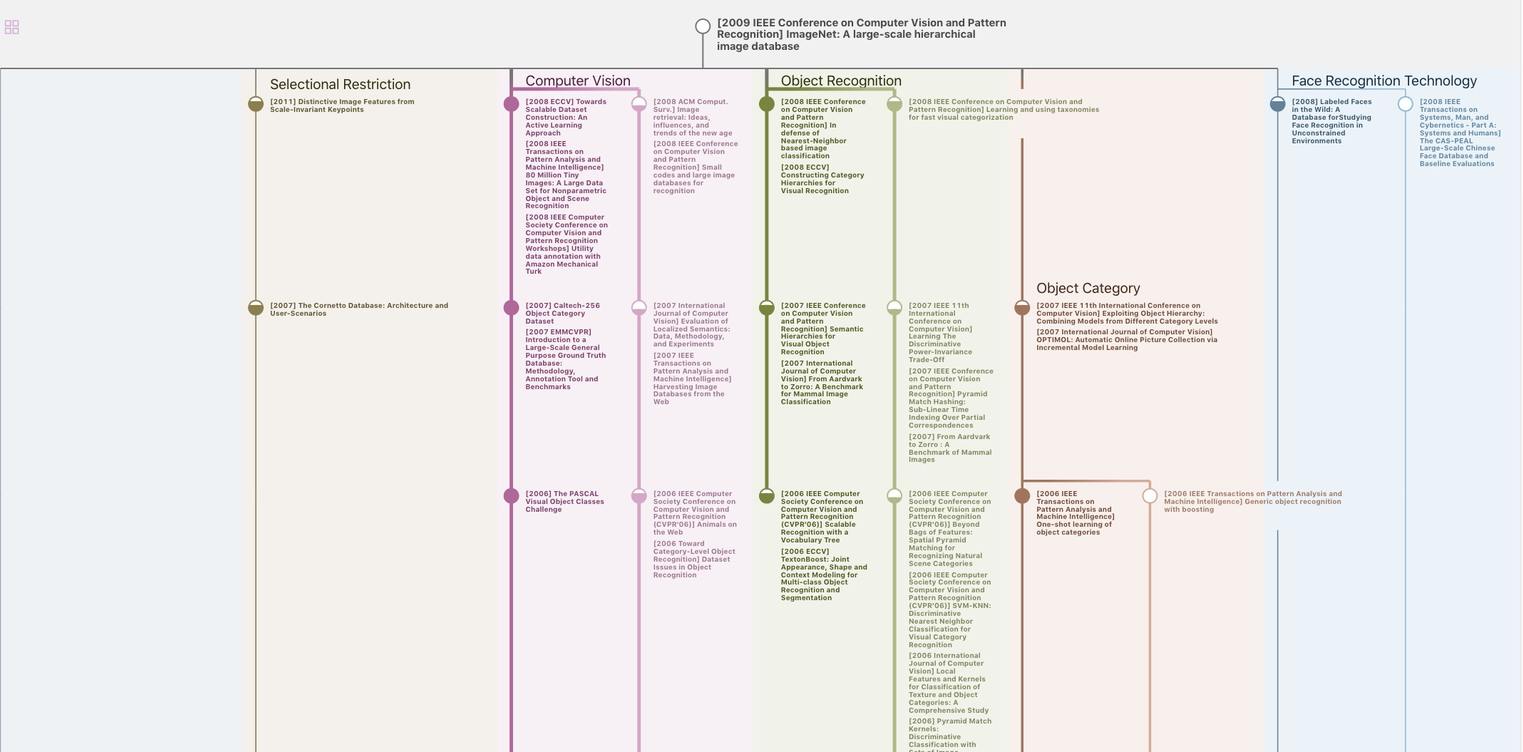
生成溯源树,研究论文发展脉络
Chat Paper
正在生成论文摘要