TOPr: Enhanced Static Code Pruning for Fast and Precise Directed Fuzzing
CoRR(2023)
摘要
Directed fuzzing is a dynamic testing technique that focuses exploration on specific, pre targeted program locations. Like other types of fuzzers, directed fuzzers are most effective when maximizing testing speed and precision. To this end, recent directed fuzzers have begun leveraging path pruning: preventing the wasteful testing of program paths deemed irrelevant to reaching a desired target location. Yet, despite code pruning's substantial speedup, current approaches are imprecise failing to capture indirect control flow requiring additional dynamic analyses that diminish directed fuzzers' speeds. Thus, without code pruning that is both fast and precise, directed fuzzers' effectiveness will continue to remain limited. This paper aims to tackle the challenge of upholding both speed and precision in pruning-based directed fuzzing. We show that existing pruning approaches fail to recover common case indirect control flow; and identify opportunities to enhance them with lightweight heuristics namely, function signature matching enabling them to maximize precision without the burden of dynamic analysis. We implement our enhanced pruning as a prototype, TOPr (Target Oriented Pruning), and evaluate it against the leading pruning based and pruning agnostic directed fuzzers SieveFuzz and AFLGo. We show that TOPr's enhanced pruning outperforms these fuzzers in (1) speed (achieving 222% and 73% higher test case throughput, respectively); (2) reachability (achieving 149% and 9% more target relevant coverage, respectively); and (3) bug discovery time (triggering bugs faster 85% and 8%, respectively). Furthermore, TOPr's balance of speed and precision enables it to find 24 new bugs in 5 open source applications, with 18 confirmed by developers, 12 bugs labelled as "Priority - 1. High", and 12 bugs fixed, underscoring the effectiveness of our framework.
更多查看译文
AI 理解论文
溯源树
样例
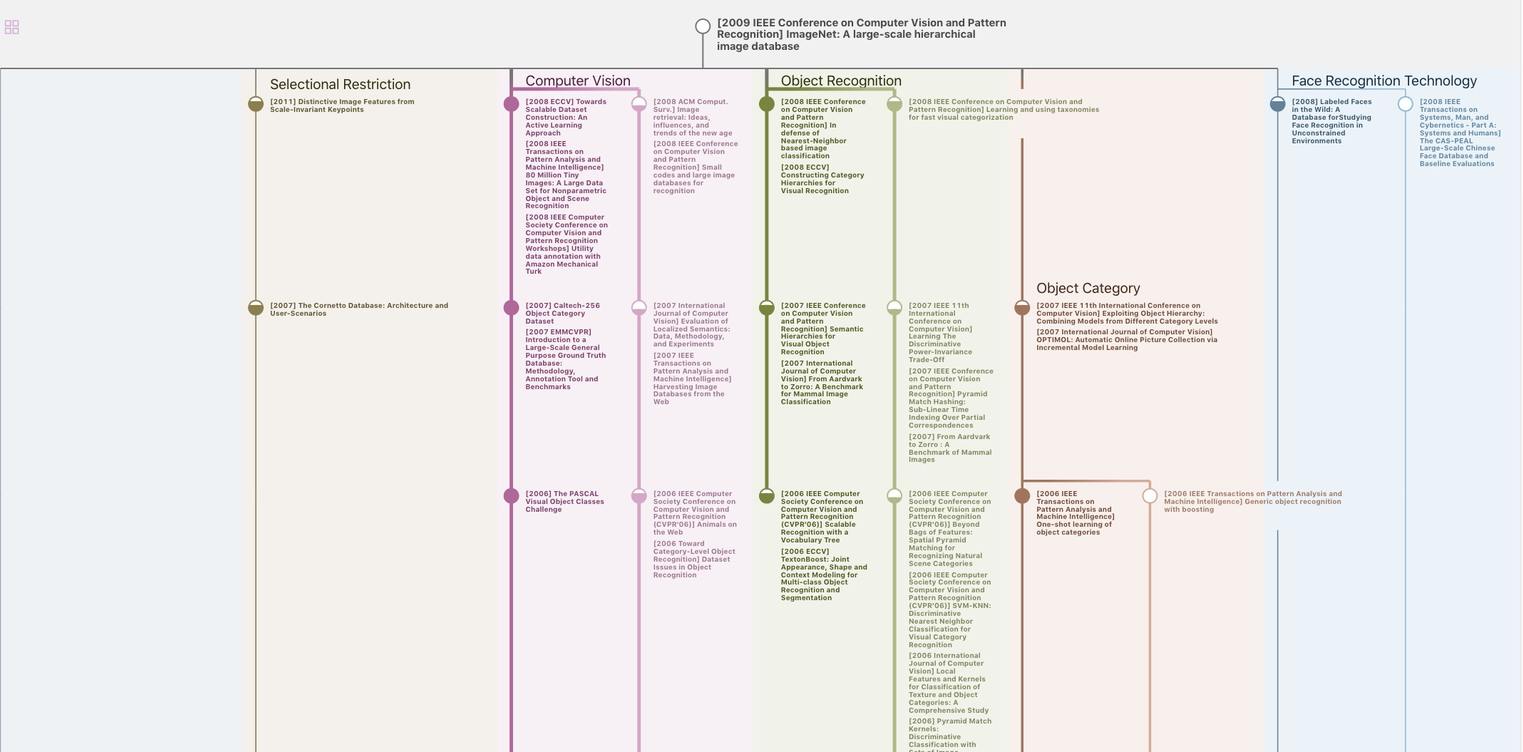
生成溯源树,研究论文发展脉络
Chat Paper
正在生成论文摘要