PromptST: Prompt-Enhanced Spatio-Temporal Multi-Attribute Prediction
PROCEEDINGS OF THE 32ND ACM INTERNATIONAL CONFERENCE ON INFORMATION AND KNOWLEDGE MANAGEMENT, CIKM 2023(2023)
摘要
In the era of information explosion, spatio-temporal data mining serves as a critical part of urban management. Considering the various fields demanding attention, e.g., traffic state, human activity, and social event, predicting multiple spatio-temporal attributes simultaneously can alleviate regulatory pressure and foster smart city construction. However, current research can not handle the spatio-temporal multi-attribute prediction well due to the complex relationships between diverse attributes. The key challenge lies in howto address the common spatio-temporal patterns while tackling their distinctions. In this paper, we propose an effective solution for spatio-temporal multi-attribute prediction, PromptST. We devise a spatio-temporal transformer and a parameter-sharing training scheme to address the common knowledge among different spatio-temporal attributes. Then, we elaborate a spatio-temporal prompt tuning strategy to fit the specific attributes in a lightweight manner. Through the pretrain and prompt tuning phases, our PromptST is able to enhance the specific spatio-temoral characteristic capture by prompting the backbone model to fit the specific target attribute while maintaining the learned common knowledge. Extensive experiments on real-world datasets verify that our PromptST attains state-of-the-art performance. Furthermore, we also prove PromptST owns good transferability on unseen spatio-temporal attributes, which brings promising application potential in urban computing. The implementation code is available to ease reproducibility.
更多查看译文
关键词
smart city,spatio-temporal prediction,multi-attribute prediction,prompt learning
AI 理解论文
溯源树
样例
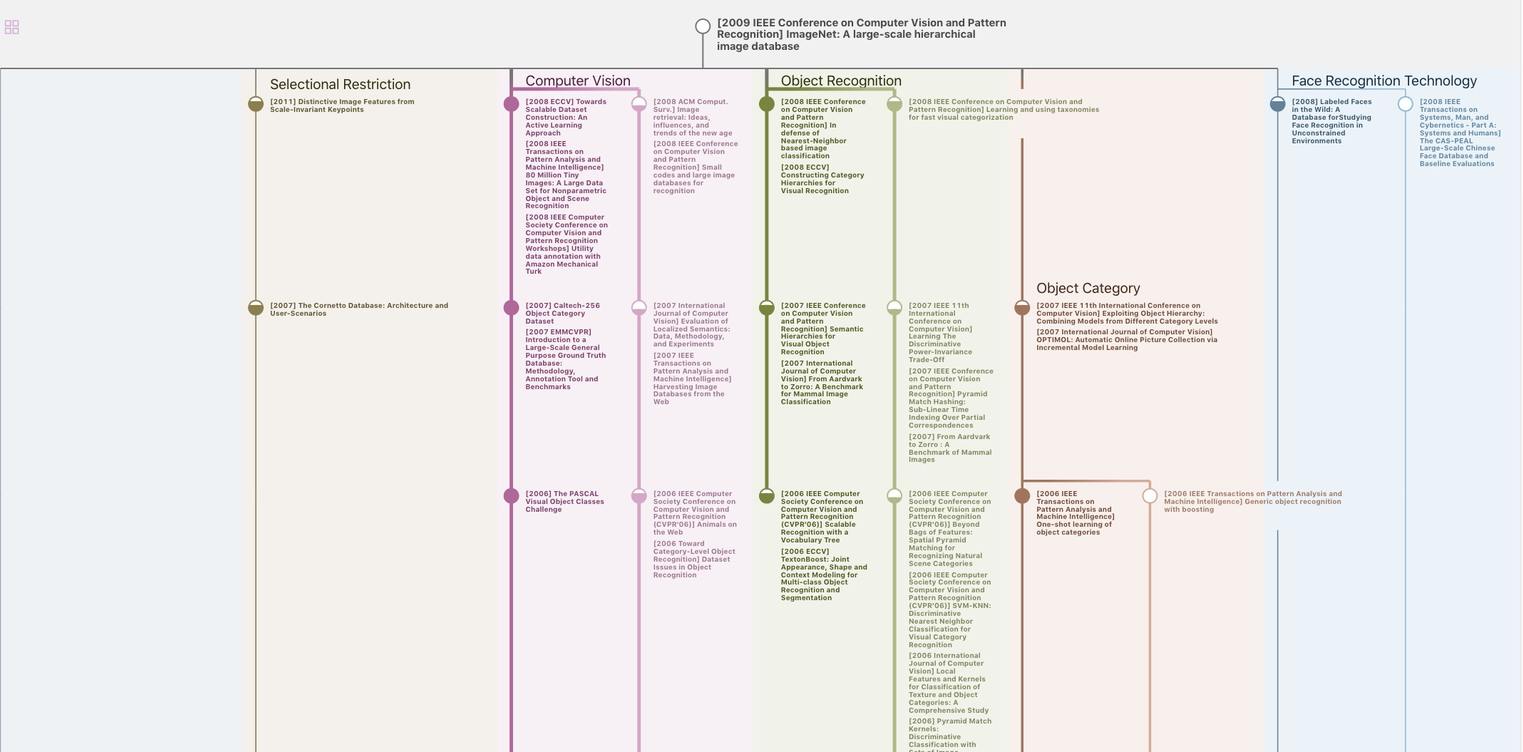
生成溯源树,研究论文发展脉络
Chat Paper
正在生成论文摘要