Stealthy Physical Masked Face Recognition Attack via Adversarial Style Optimization
IEEE TRANSACTIONS ON MULTIMEDIA(2024)
摘要
Deep neural networks (DNNs) have achieved state-of-the-art performance on face recognition (FR) tasks in the last decade. In real scenarios, the deployment of DNNs requires taking various face accessories into consideration, like glasses, hats, and masks. In the COVID-19 pandemic era, wearing face masks is one of the most effective ways to defend against the novel coronavirus. However, DNNs are known to be vulnerable to adversarial examples with a small but elaborated perturbation. Thus, a facial mask with adversarial perturbations may pose a great threat to the widely used deep learning-based FR models. In this paper, we consider a challenging adversarial setting: targeted attack against FR models. We propose a new stealthy physical masked FR attack via adversarial style optimization. Specifically, we train an adversarial style mask generator that hides adversarial perturbations inside style masks. Moreover, to ameliorate the phenomenon of sub-optimization with one fixed style, we propose to discover the optimal style given a target through style optimization in a continuous relaxation manner. We simultaneously optimize the generator and the style selection for generating strong and stealthy adversarial style masks. We evaluated the effectiveness and transferability of our proposed method via extensive white-box and black-box digital experiments. Furthermore, we also conducted physical attack experiments against local FR models and online platforms.
更多查看译文
关键词
Face recognition,Perturbation methods,Generators,Computational modeling,COVID-19,Task analysis,Optimization,Adversarial attack,computer vision,deep learning,face recognition,physical setting
AI 理解论文
溯源树
样例
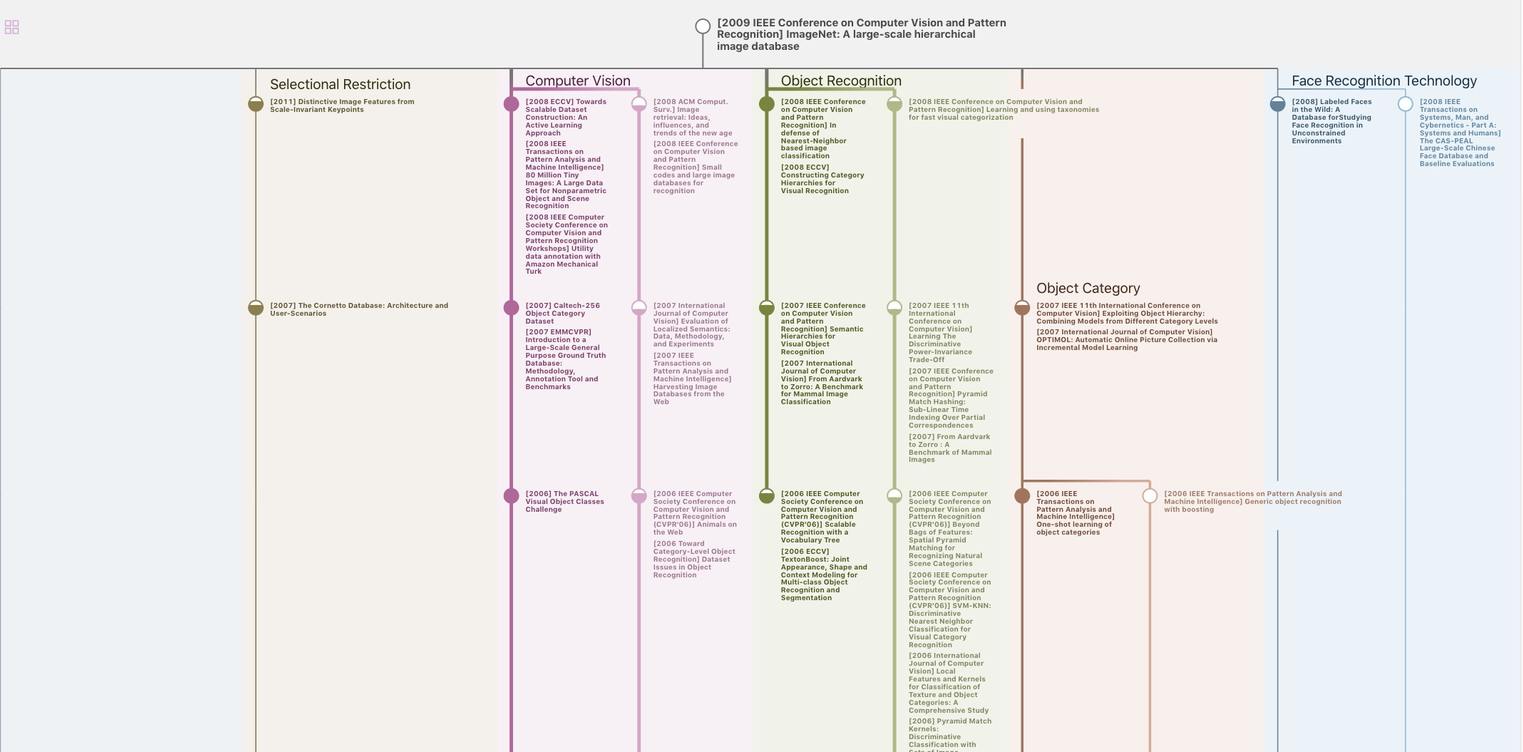
生成溯源树,研究论文发展脉络
Chat Paper
正在生成论文摘要