A Benchmark for Text Expansion: Datasets, Metrics, and Baselines
CoRR(2023)
摘要
This work presents a new task of Text Expansion (TE), which aims to insert fine-grained modifiers into proper locations of the plain text to concretize or vivify human writings. Different from existing insertion-based writing assistance tasks, TE requires the model to be more flexible in both locating and generation, and also more cautious in keeping basic semantics. We leverage four complementary approaches to construct a dataset with 12 million automatically generated instances and 2K human-annotated references for both English and Chinese. To facilitate automatic evaluation, we design various metrics from multiple perspectives. In particular, we propose Info-Gain to effectively measure the informativeness of expansions, which is an important quality dimension in TE. On top of a pre-trained text-infilling model, we build both pipelined and joint Locate&Infill models, which demonstrate the superiority over the Text2Text baselines, especially in expansion informativeness. Experiments verify the feasibility of the TE task and point out potential directions for future research toward better automatic text expansion.
更多查看译文
关键词
text expansion,baselines,benchmark,datasets
AI 理解论文
溯源树
样例
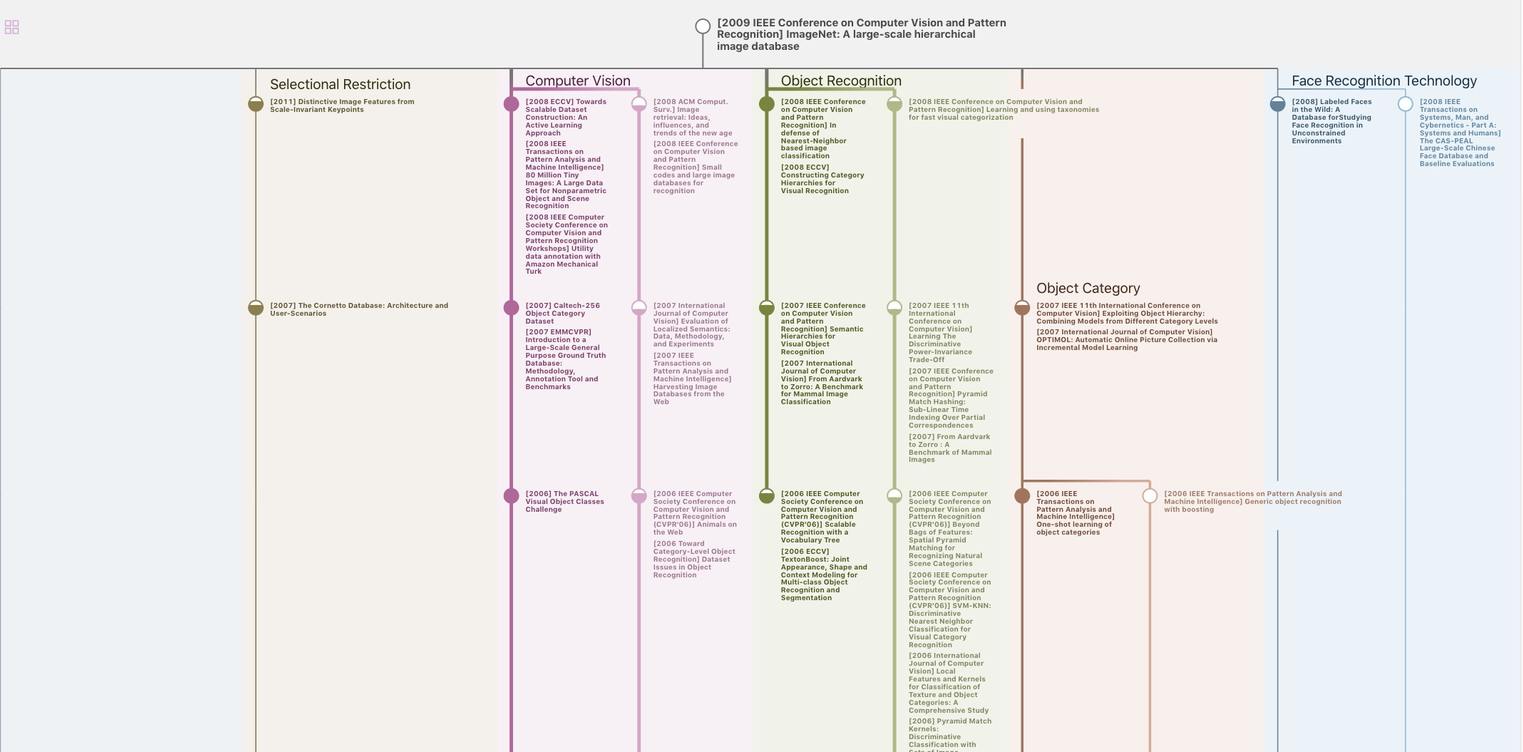
生成溯源树,研究论文发展脉络
Chat Paper
正在生成论文摘要