Total Variation Distance Estimation Is as Easy as Probabilistic Inference
CoRR(2023)
摘要
In this paper, we establish a novel connection between total variation (TV) distance estimation and probabilistic inference. In particular, we present an efficient, structure-preserving reduction from relative approximation of TV distance to probabilistic inference over directed graphical models. This reduction leads to a fully polynomial randomized approximation scheme (FPRAS) for estimating TV distances between distributions over any class of Bayes nets for which there is an efficient probabilistic inference algorithm. In particular, it leads to an FPRAS for estimating TV distances between distributions that are defined by Bayes nets of bounded treewidth. Prior to this work, such approximation schemes only existed for estimating TV distances between product distributions. Our approach employs a new notion of $partial$ couplings of high-dimensional distributions, which might be of independent interest.
更多查看译文
关键词
probabilistic
AI 理解论文
溯源树
样例
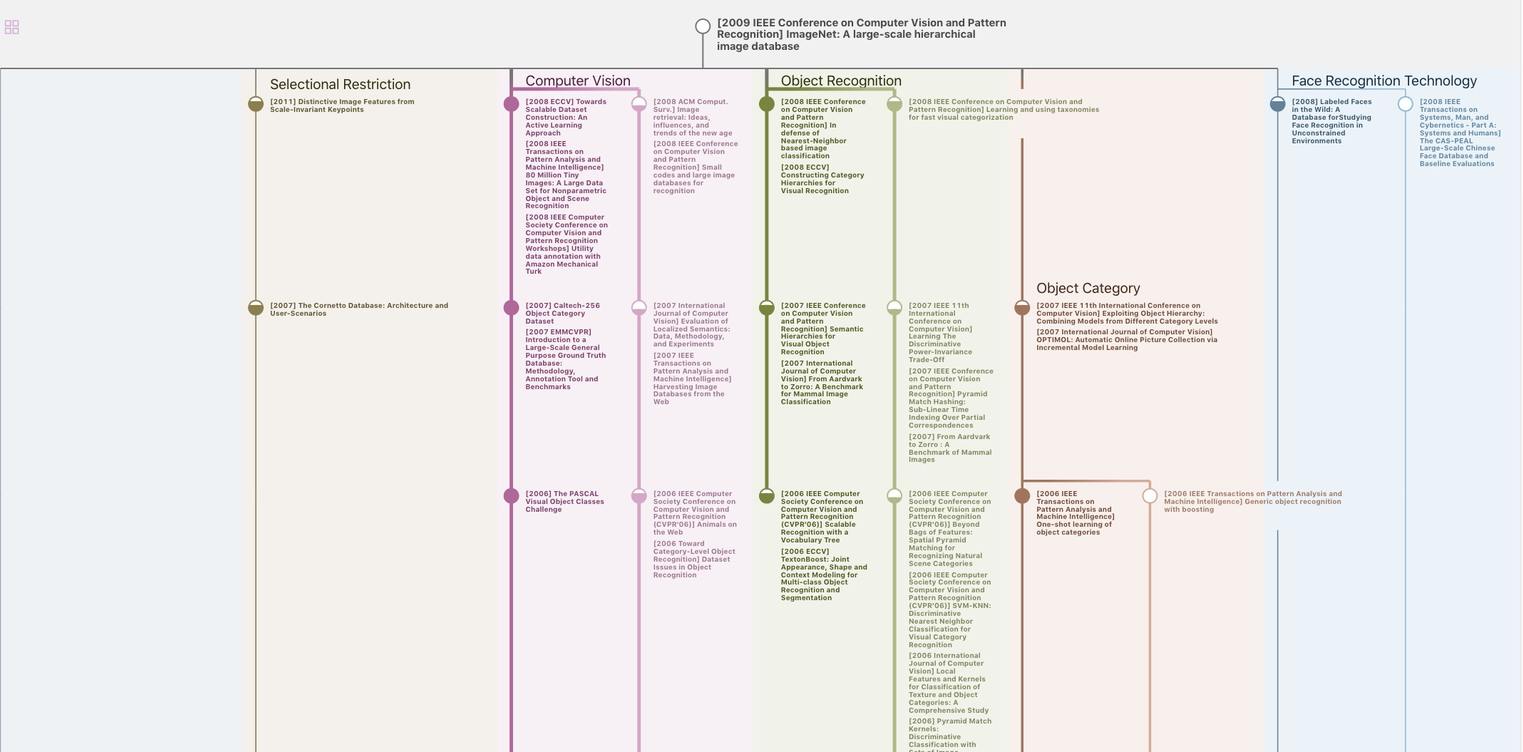
生成溯源树,研究论文发展脉络
Chat Paper
正在生成论文摘要