Using Reinforcement Learning to Simplify Mealtime Insulin Dosing for People with Type 1 Diabetes: In-Silico Experiments
CoRR(2023)
摘要
People with type 1 diabetes (T1D) struggle to calculate the optimal insulin dose at mealtime, especially when under multiple daily injections (MDI) therapy. Effectively, they will not always perform rigorous and precise calculations; but occasionally, they might rely on intuition and previous experience. Reinforcement learning (RL) has shown outstanding results in outperforming humans on tasks requiring intuition and learning from experience. In this work, we propose an RL agent that recommends the optimal meal-accompanying insulin dose corresponding to a qualitative meal (QM) strategy that does not require precise carbohydrate counting (CC) (e.g., a usual meal at noon.). The agent is trained using the soft actor-critic approach and comprises long short-term memory (LSTM) neurons. For training, eighty virtual subjects (VS) of the FDA-accepted UVA/Padova T1D adult population were simulated using MDI therapy and QM strategy. For validation, the remaining twenty VS were examined in 26-week scenarios, including intra- and inter-day variabilities in glucose. In-silico results showed that the proposed RL approach outperforms a baseline run-to-run approach and can replace the standard CC approach. Specifically, after 26 weeks, the time-in-range (70 - 180mg/dL) and time-in-hypoglycemia (< 70mg/dL) were 73.1 ± 11.6% and 2.0 ± 1.8% using the RL-optimized QM strategy compared to 70.6± 14.8% and 1.5± 1.5% using CC. Such an approach can simplify diabetes treatment resulting in improved quality of life and glycemic outcomes.
更多查看译文
关键词
mealtime insulin dosing,reinforcement learning,diabetes,in-silico
AI 理解论文
溯源树
样例
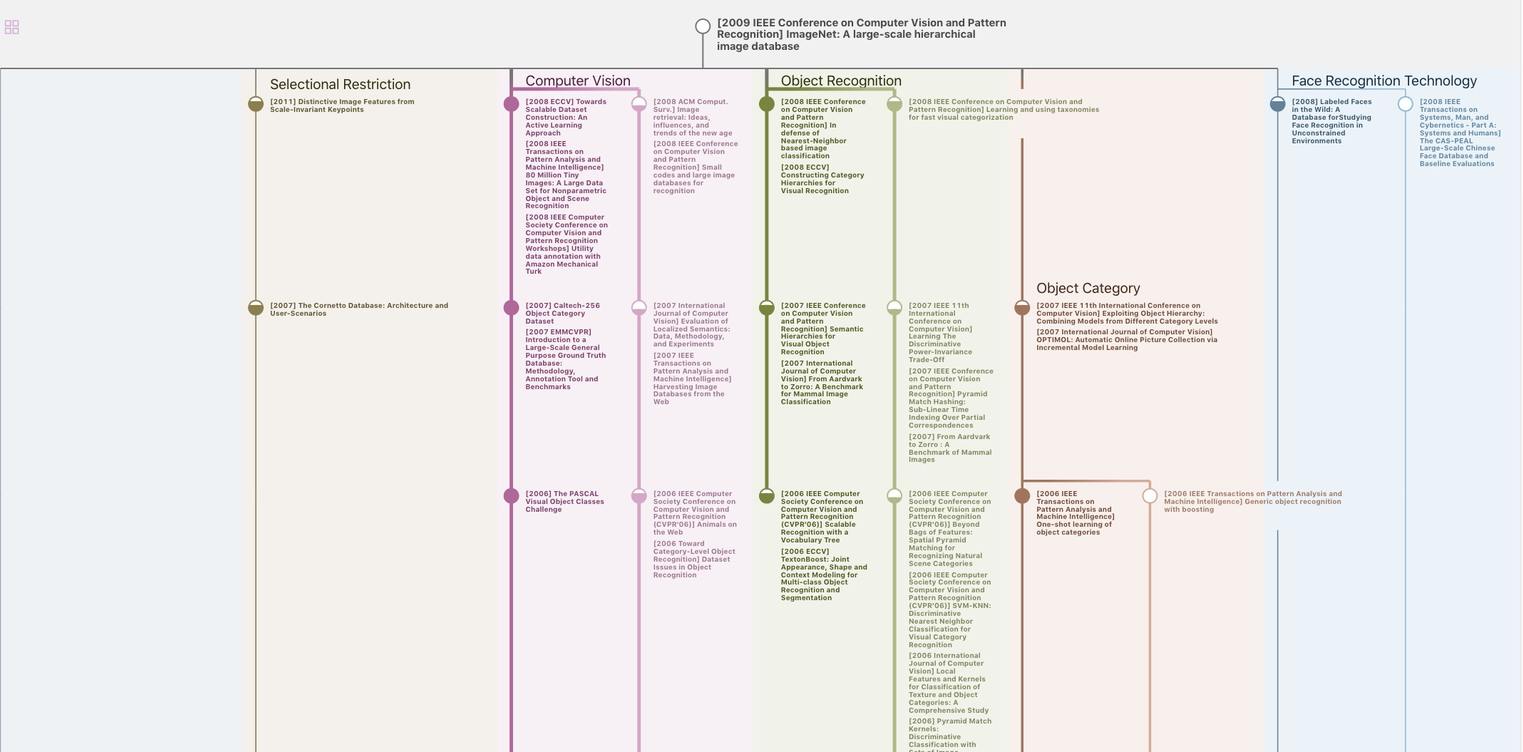
生成溯源树,研究论文发展脉络
Chat Paper
正在生成论文摘要