SafeShift: Safety-Informed Distribution Shifts for Robust Trajectory Prediction in Autonomous Driving
2024 IEEE Intelligent Vehicles Symposium (IV)(2024)
Abstract
As autonomous driving technology matures, safety and robustness of its keycomponents, including trajectory prediction, is vital. Though real-worlddatasets, such as Waymo Open Motion, provide realistic recorded scenarios formodel development, they often lack truly safety-critical situations. Ratherthan utilizing unrealistic simulation or dangerous real-world testing, weinstead propose a framework to characterize such datasets and find hiddensafety-relevant scenarios within. Our approach expands the spectrum ofsafety-relevance, allowing us to study trajectory prediction models under asafety-informed, distribution shift setting. We contribute a generalizedscenario characterization method, a novel scoring scheme to find subtly-avoidedrisky scenarios, and an evaluation of trajectory prediction models in thissetting. We further contribute a remediation strategy, achieving a 10reduction in prediction collision rates. To facilitate future research, werelease our code to the public: github.com/cmubig/SafeShift
MoreTranslated text
Key words
robust trajectory,safeshift,distribution shifts,prediction,safety-informed
AI Read Science
Must-Reading Tree
Example
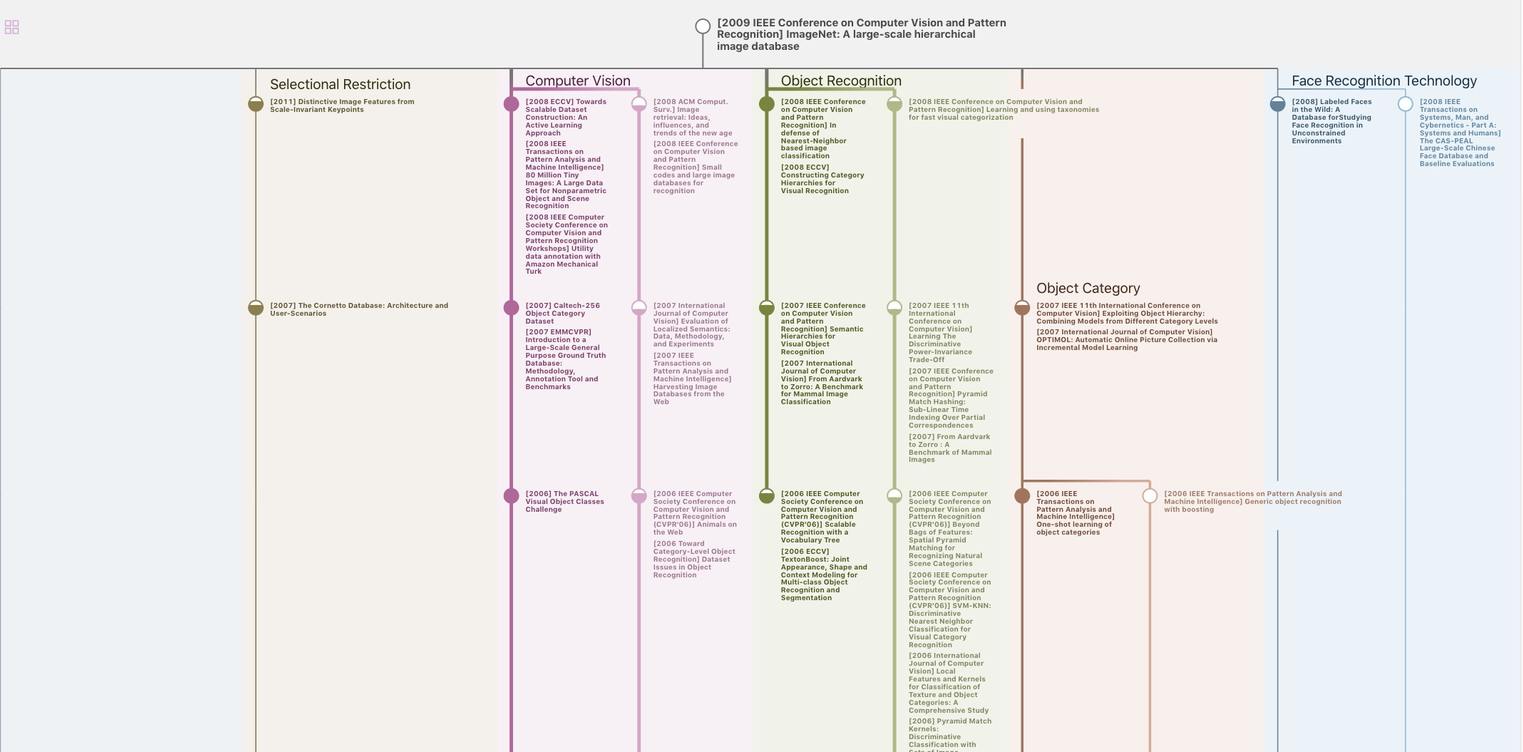
Generate MRT to find the research sequence of this paper
Chat Paper
Summary is being generated by the instructions you defined