Mining Patents with Large Language Models Demonstrates Congruence of Functional Labels and Chemical Structures
CoRR(2023)
摘要
Predicting chemical function from structure is a major goal of the chemical sciences, from the discovery and repurposing of novel drugs to the creation of new materials. Recently, new machine learning algorithms are opening up the possibility of general predictive models spanning many different chemical functions. Here, we consider the challenge of applying large language models to chemical patents in order to consolidate and leverage the information about chemical functionality captured by these resources. Chemical patents contain vast knowledge on chemical function, but their usefulness as a dataset has historically been neglected due to the impracticality of extracting high-quality functional labels. Using a scalable ChatGPT-assisted patent summarization and word-embedding label cleaning pipeline, we derive a Chemical Function (CheF) dataset, containing 100K molecules and their patent-derived functional labels. The functional labels were validated to be of high quality, allowing us to detect a strong relationship between functional label and chemical structural spaces. Further, we find that the co-occurrence graph of the functional labels contains a robust semantic structure, which allowed us in turn to examine functional relatedness among the compounds. We then trained a model on the CheF dataset, allowing us to assign new functional labels to compounds. Using this model, we were able to retrodict approved Hepatitis C antivirals, uncover an antiviral mechanism undisclosed in the patent, and identify plausible serotonin-related drugs. The CheF dataset and associated model offers a promising new approach to predict chemical functionality.
更多查看译文
关键词
patents,functional labels,large language models,chemical structures
AI 理解论文
溯源树
样例
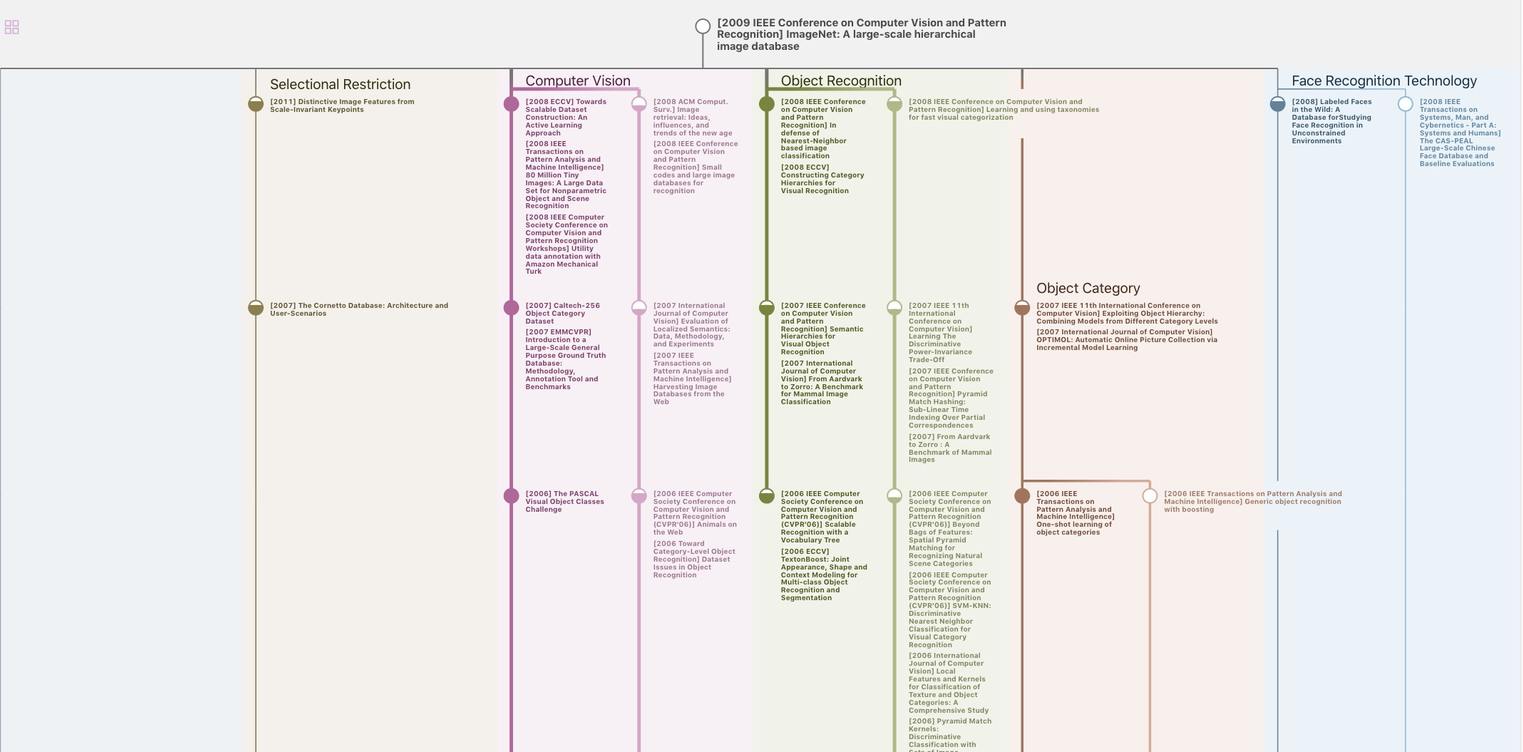
生成溯源树,研究论文发展脉络
Chat Paper
正在生成论文摘要