Recovering from Privacy-Preserving Masking with Large Language Models
ICASSP 2024 - 2024 IEEE International Conference on Acoustics, Speech and Signal Processing (ICASSP)(2023)
Abstract
Model adaptation is crucial to handle the discrepancy between proxy training data and actual users data received. To effectively perform adaptation, textual data of users is typically stored on servers or their local devices, where downstream natural language processing (NLP) models can be directly trained using such in-domain data. However, this might raise privacy and security concerns due to the extra risks of exposing user information to adversaries. Replacing identifying information in textual data with a generic marker has been recently explored. In this work, we leverage large language models (LLMs) to suggest substitutes of masked tokens and have their effectiveness evaluated on downstream language modeling tasks. Specifically, we propose multiple pre-trained and fine-tuned LLM-based approaches and perform empirical studies on various datasets for the comparison of these methods. Experimental results show that models trained on the obfuscation corpora are able to achieve comparable performance with the ones trained on the original data without privacy-preserving token masking.
MoreTranslated text
Key words
Privacy-preserving machine learning,language modeling,large language models,automatic speech recognition
AI Read Science
Must-Reading Tree
Example
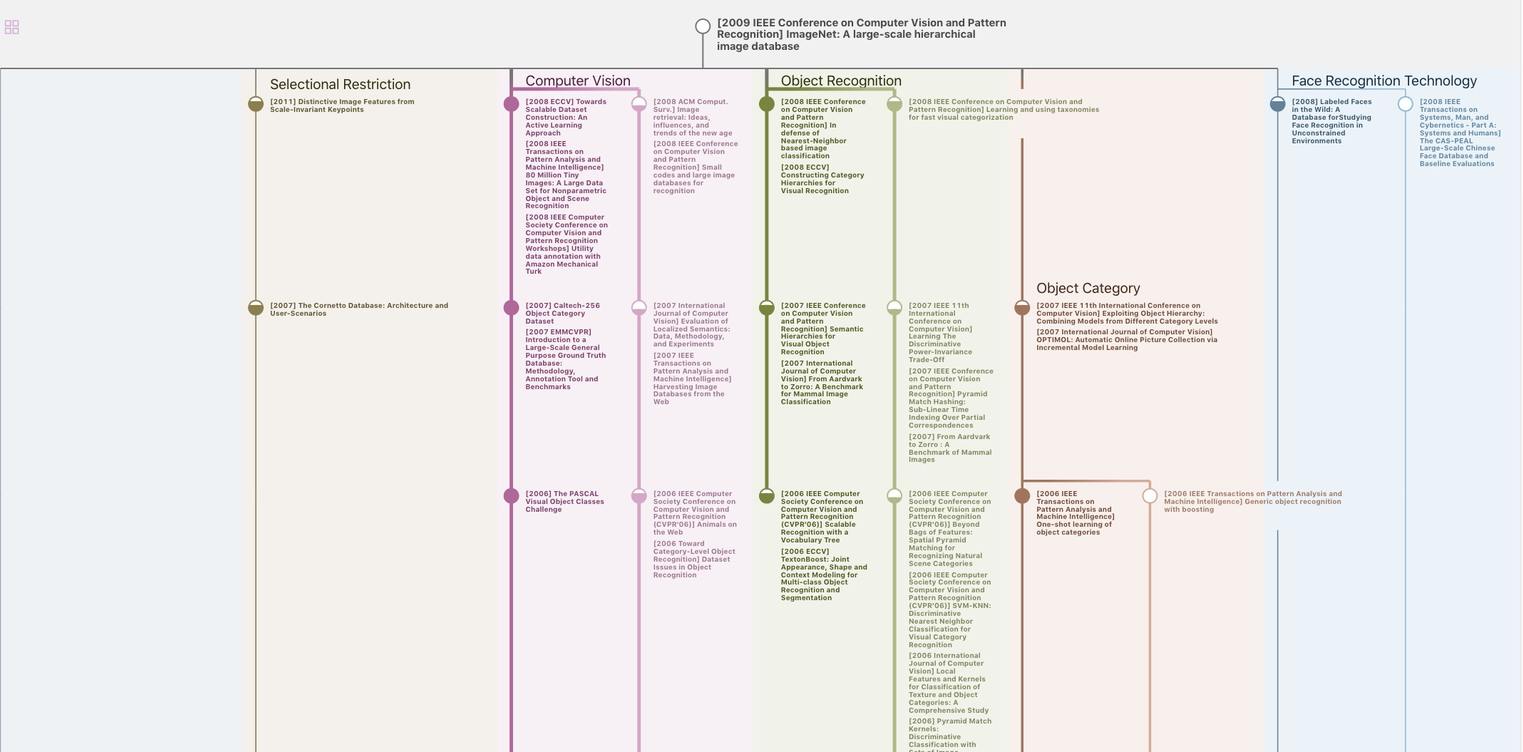
Generate MRT to find the research sequence of this paper
Chat Paper
Summary is being generated by the instructions you defined