Perceiving the Ukraine-Russia Conflict: Topic Modeling and Clustering on Twitter Data
2023 IEEE Ninth International Conference on Big Data Computing Service and Applications (BigDataService)(2023)
摘要
This study aims to gain insight into public perception of the ongoing Russia-Ukraine conflict by analyzing tweets collected since March 2022. Text-mining techniques, including Latent Dirichlet Allocation (LDA), Latent Semantic Analysis (LSA), and Non-Negative Matrix Factorization (NMF), are used to analyze high-frequency words in tweets and identify patterns. Additionally, clustering techniques such as K-means and Hierarchical-Density-Based Spatial Clustering of Applications with Noise (HDBSCAN) are applied to dense tweet embeddings to create semantic topical groupings, which we compare to traditional topic modeling approaches using a coherence metric. We demonstrate the effectiveness of the proposed methodology by identifying the commonly used terms, the meaningful topics, and the most discussed topic among tweets related to the Russia-Ukraine conflict to help gain a deeper understanding of public discourse on the conflict.
更多查看译文
关键词
Semantic Analysis,Topic Modeling,Latent Dirichlet Allocation,Latent Semantic Analysis,Non-Negative Matrix Factorization,Clustering Algorithm,K-Means Clustering,HDBSCAN
AI 理解论文
溯源树
样例
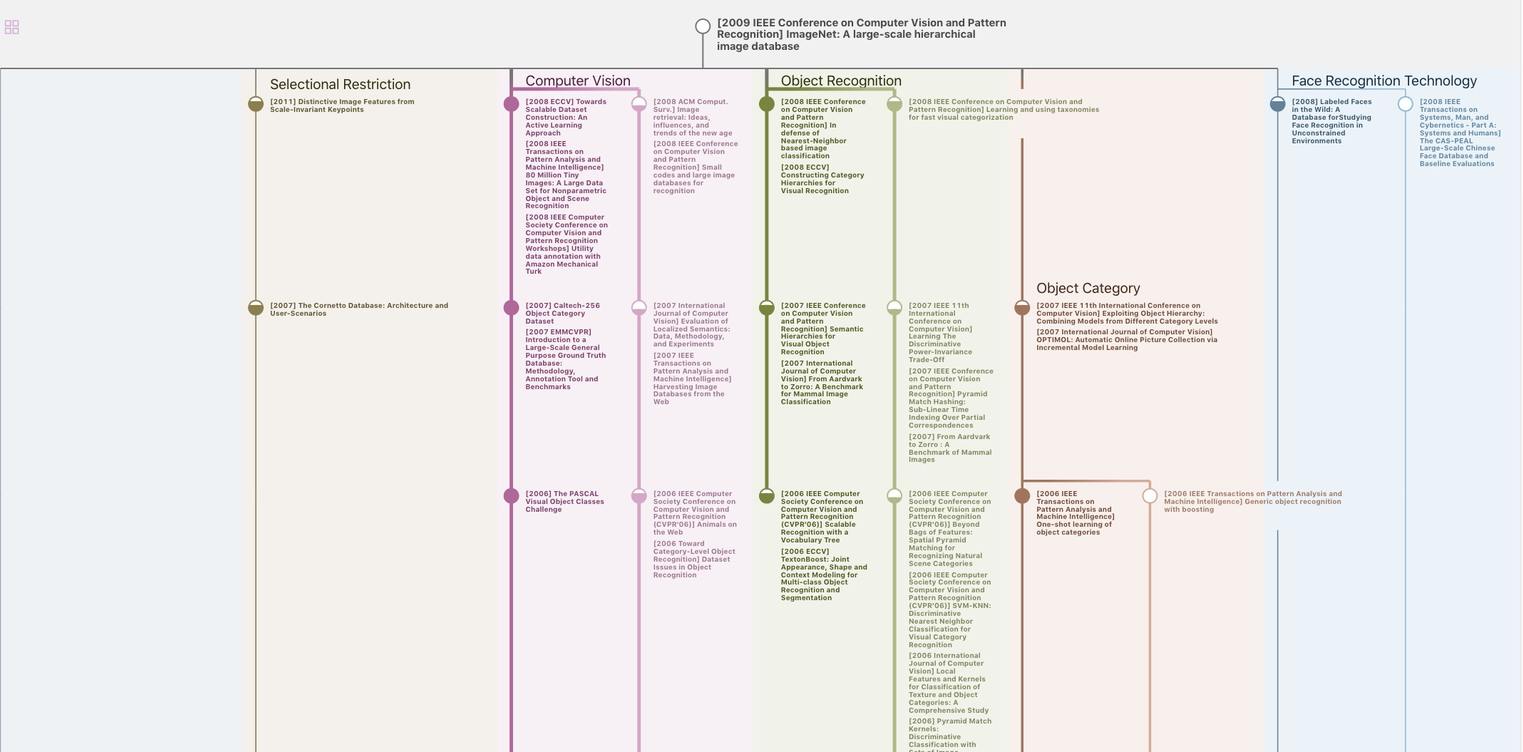
生成溯源树,研究论文发展脉络
Chat Paper
正在生成论文摘要