Estimating feature importance in circuit network using machine learning
MULTIMEDIA TOOLS AND APPLICATIONS(2023)
摘要
Identifying the feature of the circuit network is a crucial step to understanding the behavior of the Very Large Scale Integration (VLSI). Unfortunately, the growing complexity of the VLSI design makes enormous computations for estimating the property of the network. We propose a machine learning framework to overcome the intractable estimation of feature importance in the circuit network. We extract complex network features at the placement stage and compute circuit wire length at the routing stage, then study their correlation using machine learning and estimate the importance of complex network features by the learned correlation. The experimental result on TAU 2017 Benchmark shows the high efficiency of the framework that the prediction accuracy achieves an average of 96.722%. The estimated importance of complex network features is in order of the number of nodes, the average degree, the average edge weight, the average betweenness, the average strength, and the average weighted clustering coefficient. The result is convincing and consistent with the previous work, demonstrating the reliability of our method.
更多查看译文
关键词
Machine learning,Complex network,Centrality,Physical design,Estimation
AI 理解论文
溯源树
样例
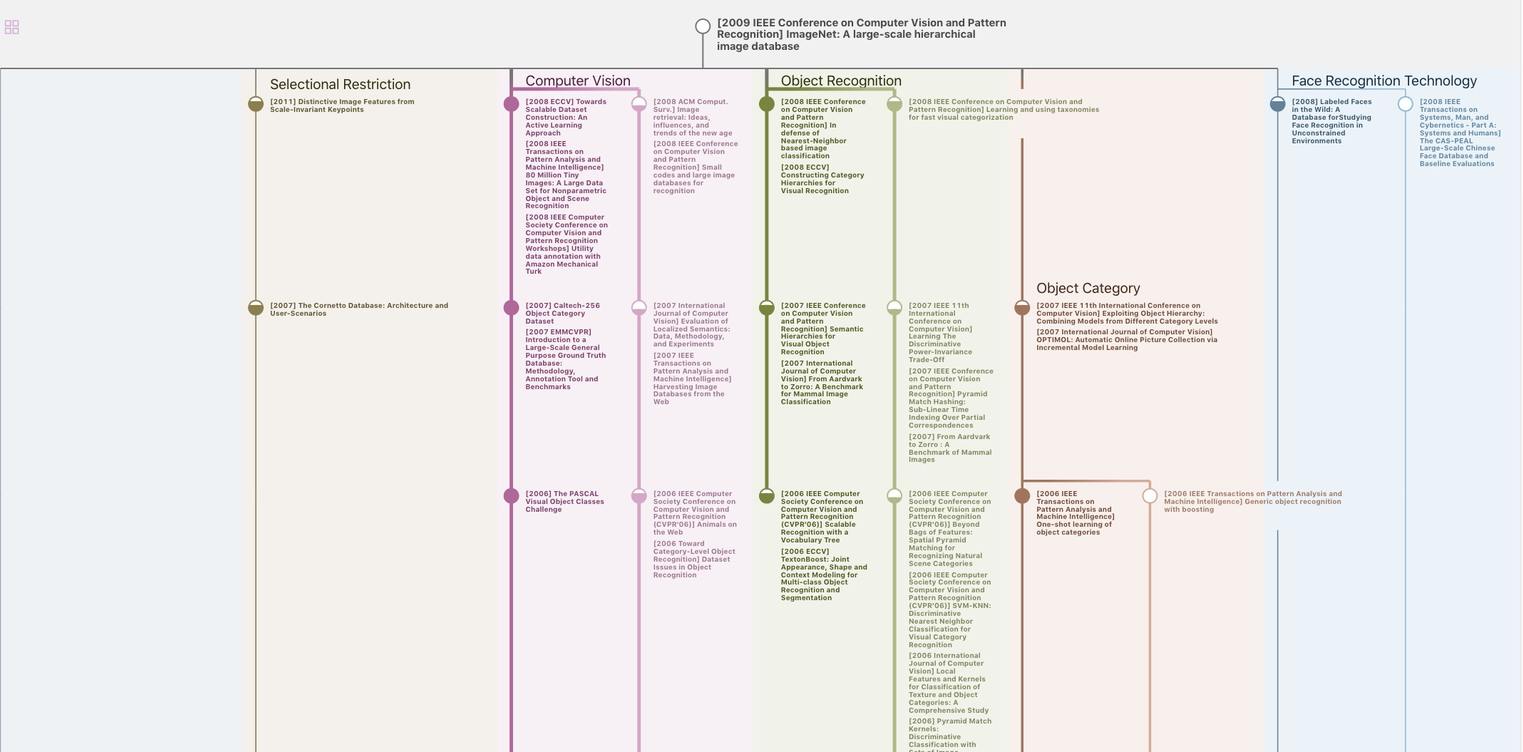
生成溯源树,研究论文发展脉络
Chat Paper
正在生成论文摘要