Visualizations for universal deep-feature representations: survey and taxonomy
KNOWLEDGE AND INFORMATION SYSTEMS(2024)
摘要
In data science and content-based retrieval, we find many domain-specific techniques that employ a data processing pipeline with two fundamental steps. First, data entities are represented by some visualizations, while in the second step, the visualizations are used with a machine learning model to extract deep features. Deep convolutional neural networks (DCNN) became the standard and reliable choice. The purpose of using DCNN is either a specific classification task or just a deep feature representation of visual data for additional processing (e.g., similarity search). Whereas the deep feature extraction is a domain-agnostic step in the pipeline (inference of an arbitrary visual input), the visualization design itself is domain-dependent and ad hoc for every use case. In this paper, we survey and analyze many instances of data visualizations used with deep learning models (mostly DCNN) for domain-specific tasks. Based on the analysis, we synthesize a taxonomy that provides a systematic overview of visualization techniques suitable for usage with the models. The aim of the taxonomy is to enable the future generalization of the visualization design process to become completely domain-agnostic, leading to the automation of the entire feature extraction pipeline. As the ultimate goal, such an automated pipeline could lead to universal deep feature data representations for content-based retrieval.
更多查看译文
关键词
Taxonomy,Visualization,Deep features,DCNN,Data processing pipeline,Similarity search,Data representation,Content-based retrieval
AI 理解论文
溯源树
样例
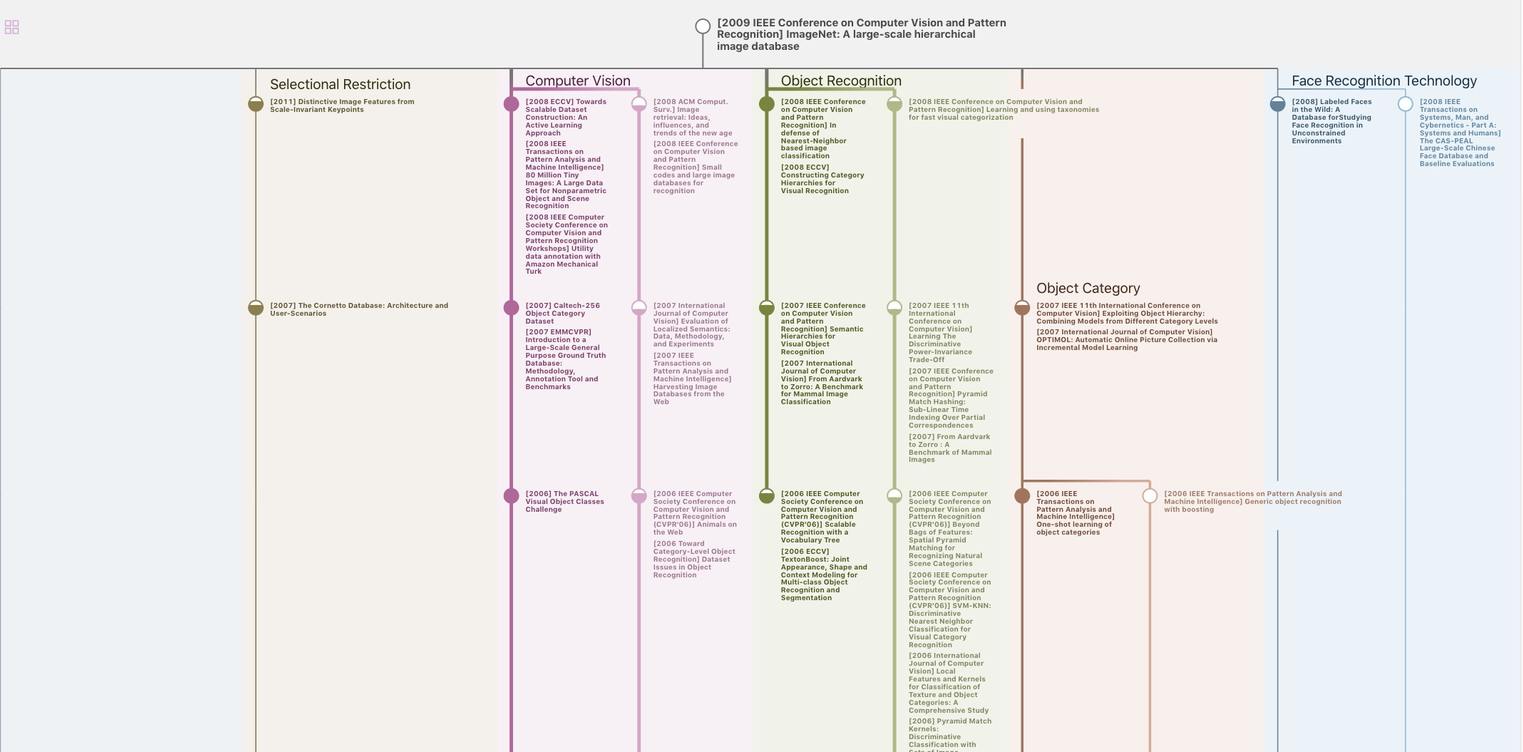
生成溯源树,研究论文发展脉络
Chat Paper
正在生成论文摘要