A new method of modeling the multi-stage decision-making process of CRT using machine learning with uncertainty quantification.
ArXiv(2024)
摘要
Aims:Current machine learning-based (ML) models usually attempt to utilize all available patient data to predict patient outcomes while ignoring the associated cost and time for data acquisition. The purpose of this study is to create a multi-stage machine learning model to predict cardiac resynchronization therapy (CRT) response for heart failure (HF) patients. This model exploits uncertainty quantification to recommend additional collection of single-photon emission computed tomography myocardial perfusion imaging (SPECT MPI) variables if baseline clinical variables and features from electrocardiogram (ECG) are not sufficient.
Methods:218 patients who underwent rest-gated SPECT MPI were enrolled in this study. CRT response was defined as an increase in left ventricular ejection fraction (LVEF) > 5% at a 6±1 month follow-up. A multi-stage ML model was created by combining two ensemble models: Ensemble 1 was trained with clinical variables and ECG; Ensemble 2 included Ensemble 1 plus SPECT MPI features. Uncertainty quantification from Ensemble 1 allowed for multi-stage decision-making to determine if the acquisition of SPECT data for a patient is necessary. The performance of the multi-stage model was compared with that of Ensemble models 1 and 2.
Results:The response rate for CRT was 55.5% (n = 121) with overall male gender 61.0% (n = 133), an average age of 62.0±11.8, and LVEF of 27.7±11.0. The multi-stage model performed similarly to Ensemble 2 (which utilized the additional SPECT data) with AUC of 0.75 vs. 0.77, accuracy of 0.71 vs. 0.69, sensitivity of 0.70 vs. 0.72, and specificity 0.72 vs. 0.65, respectively. However, the multi-stage model only required SPECT MPI data for 52.7% of the patients across all folds.
Conclusions:By using rule-based logic stemming from uncertainty quantification, the multi-stage model was able to reduce the need for additional SPECT MPI data acquisition without sacrificing performance.
更多查看译文
AI 理解论文
溯源树
样例
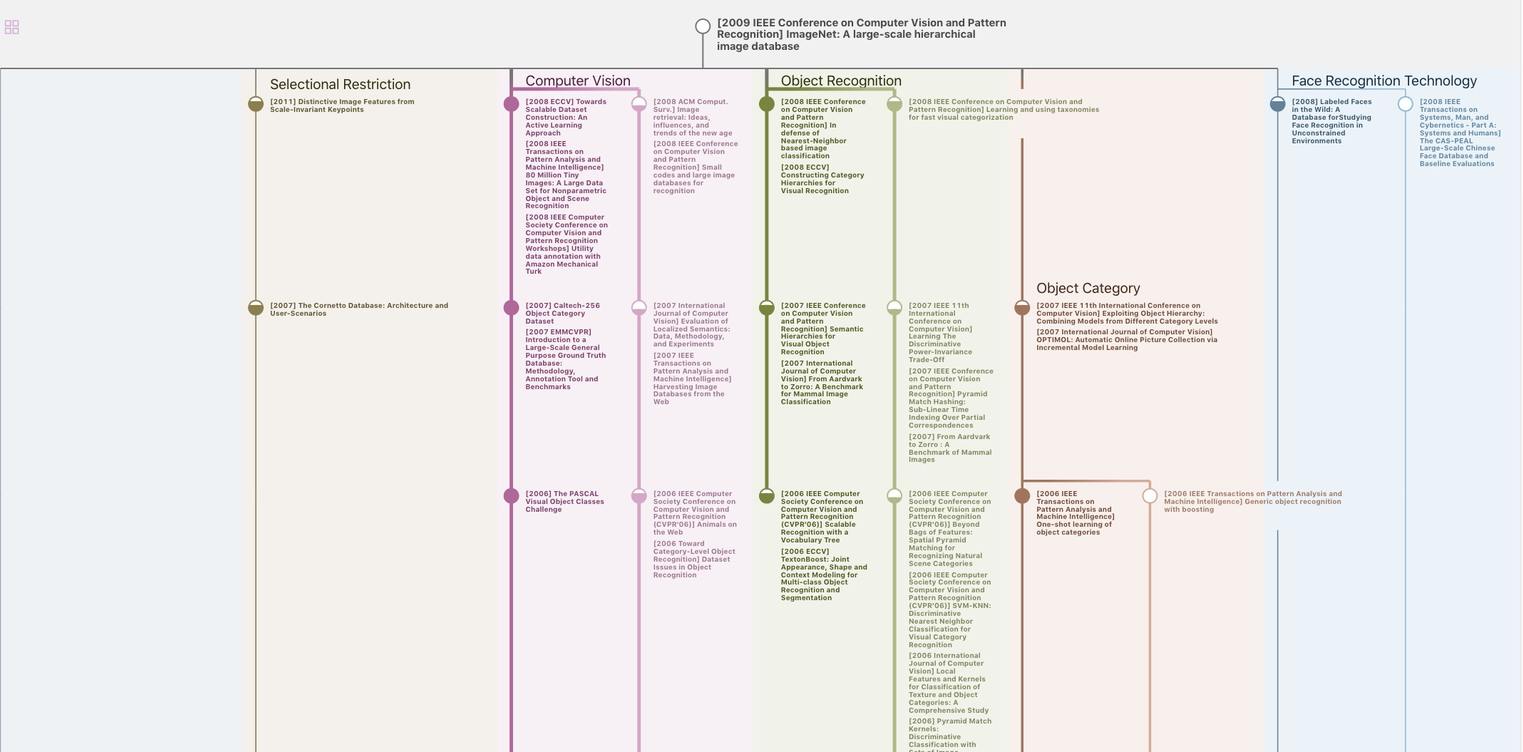
生成溯源树,研究论文发展脉络
Chat Paper
正在生成论文摘要