Malicious Cyber Activity Detection Using Zigzag Persistence
CoRR(2023)
摘要
In this study we synthesize zigzag persistence from topological data analysis with autoencoder-based approaches to detect malicious cyber activity and derive analytic insights. Cybersecurity aims to safeguard computers, networks, and servers from various forms of malicious attacks, including network damage, data theft, and activity monitoring. Here we focus on the detection of malicious activity using log data. To do this we consider the dynamics of the data by exploring the changing topology of a hypergraph representation gaining insights into the underlying activity. Hypergraphs provide a natural representation of cyber log data by capturing complex interactions between processes. To study the changing topology we use zigzag persistence which captures how topological features persist at multiple dimensions over time. We observe that the resulting barcodes represent malicious activity differently than benign activity. To automate this detection we implement an autoencoder trained on a vectorization of the resulting zigzag persistence barcodes. Our experimental results demonstrate the effectiveness of the autoencoder in detecting malicious activity in comparison to standard summary statistics. Overall, this study highlights the potential of zigzag persistence and its combination with temporal hypergraphs for analyzing cybersecurity log data and detecting malicious behavior.
更多查看译文
关键词
malicious cyber activity detection,persistence
AI 理解论文
溯源树
样例
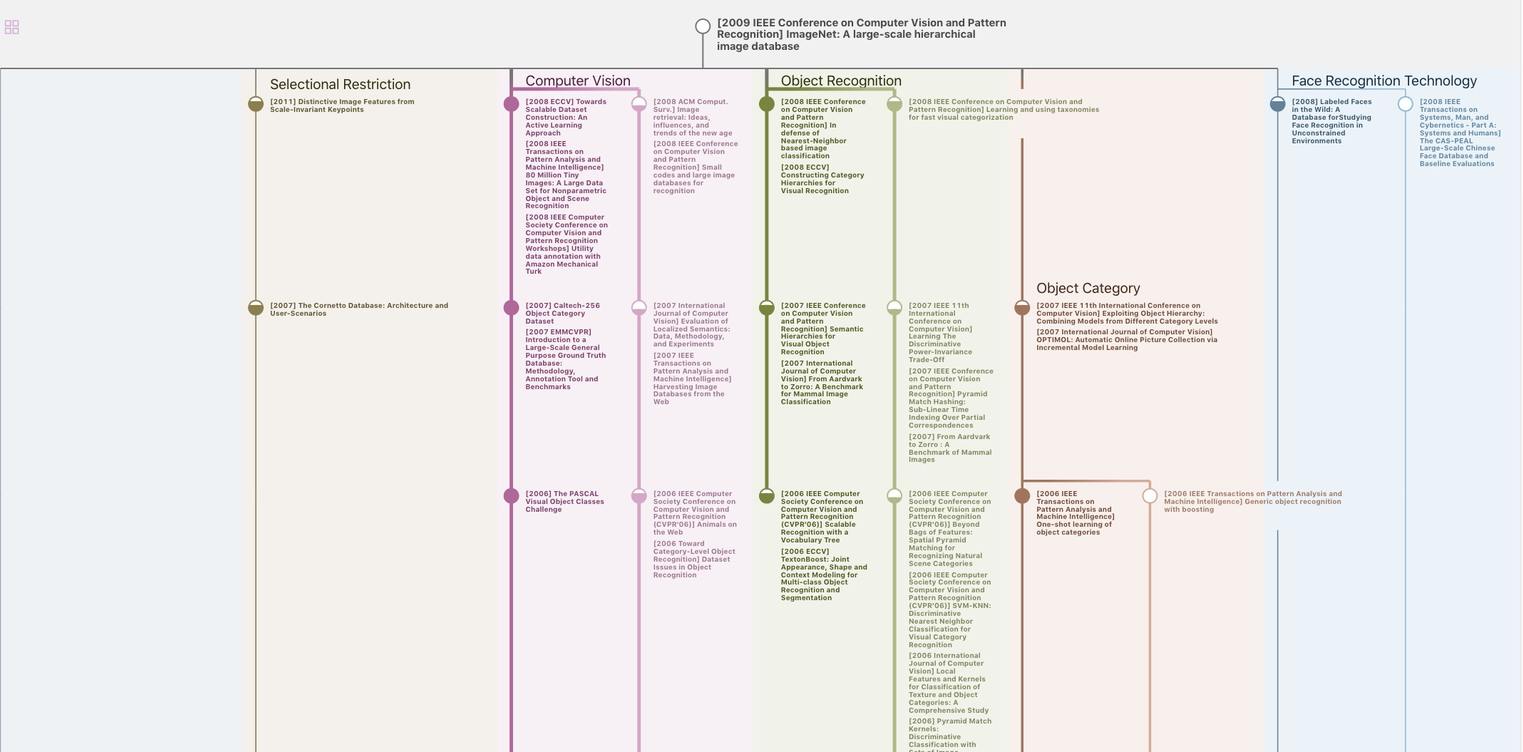
生成溯源树,研究论文发展脉络
Chat Paper
正在生成论文摘要