Location as Supervision for Weakly Supervised Multi-Channel Source Separation of Machine Sounds
2023 IEEE Workshop on Applications of Signal Processing to Audio and Acoustics (WASPAA)(2023)
Abstract
In this work, we are interested in learning a model to separate sources that cannot be recorded in isolation, such as parts of a machine that must run simultaneously in order for the machine to function. We assume the presence of a microphone array and knowledge of the source locations (potentially obtained from schematics or an auxiliary sensor such as a camera). Our method uses the source locations as weak labels for learning to separate the sources, since we cannot obtain the isolated source signals typically used as training targets. We propose a loss function that requires the directional features computed from the separated sources to match the true direction of arrival for each source, and also include a reconstruction loss to ensure all frequencies are taken into account by at least one of the separated sources output by our model. We benchmark the performance of our algorithm using synthetic mixtures created using machine sounds from the DCASE 2021 Task 2 dataset in challenging reverberant conditions. While reaching lower objective scores than a model with access to isolated source signals for training, our proposed weakly-supervised model obtains promising results and applies to industrial scenarios where collecting isolated source signals is prohibitively expensive or impossible.
MoreTranslated text
Key words
Multichannel source separation, weak supervision, directional features, machine sound
AI Read Science
Must-Reading Tree
Example
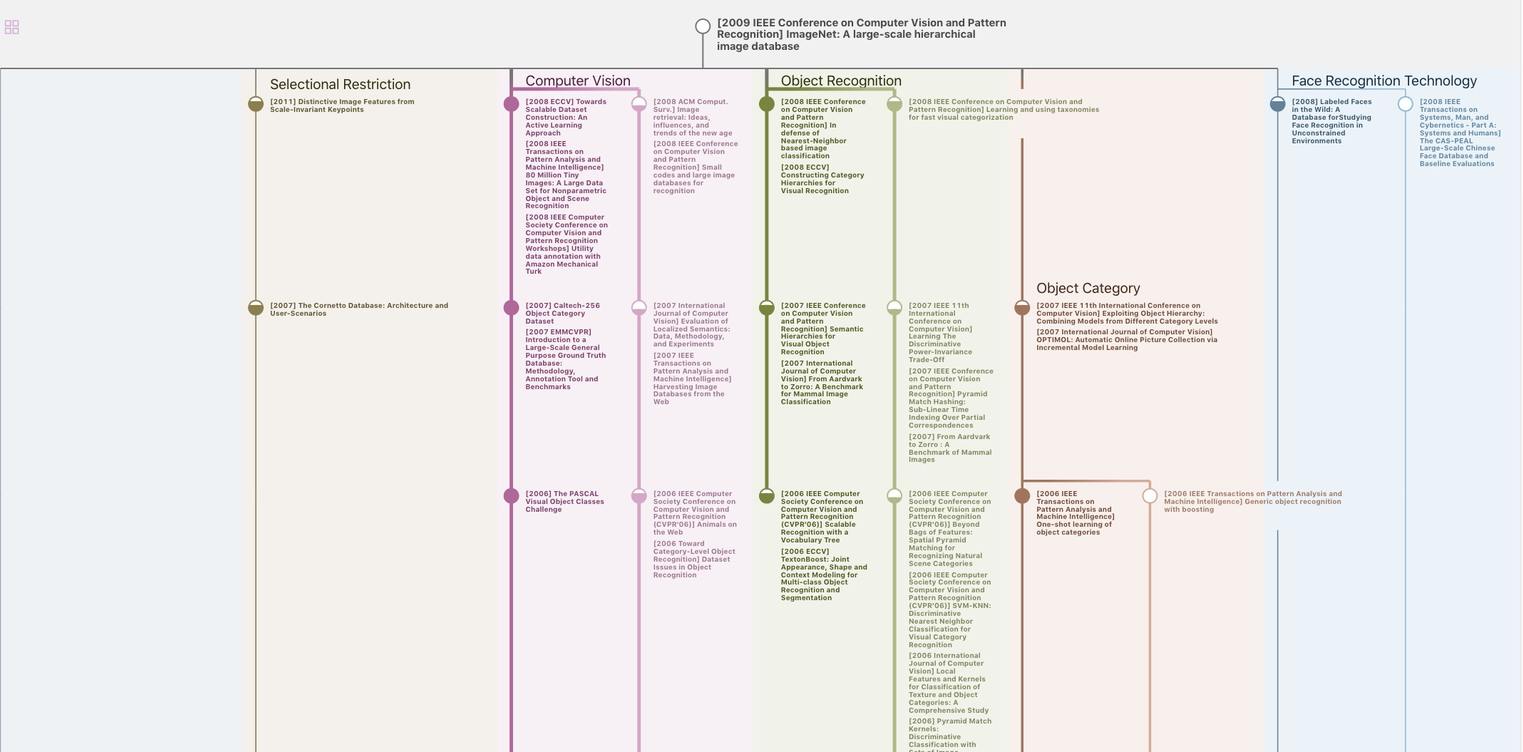
Generate MRT to find the research sequence of this paper
Chat Paper
Summary is being generated by the instructions you defined