Visual-based Fall Detection Characterization Using YOLOv3 Algorithm
2023 International Conference on Digital Applications, Transformation & Economy (ICDATE)(2023)
摘要
Falls are considered the leading cause of injuries and hospitalizations, especially in elderly. Rapid detection of falls and timely medical intervention can significantly reduce the risk of serious injuries and complications. This study aims to optimize the capability of the YOLOv3 algorithm to detect prone and supine fall events based on different lighting conditions such as 25 lux, 225 lux, and 360 lux. The proposed methodology utilized a custom dataset of 800 images containing both prone and supine positions; thus, the dataset was subsequently pre-processed and augmented. The model obtained an mAP of 95.4% with a precision of 85.8% and a recall of 94.2%. Given the three levels of background illuminations, the 25-lux illumination performed poorly with a 33.33% accuracy score and a 66.67% false detection rate. The brightest lighting condition at 360 lux functioned satisfactorily with a 73.33% accuracy score and a 26.67% false detection rate. Thus, it can be concluded that illumination does indeed affect the system’s detection ability, with higher illumination producing higher accuracy.
更多查看译文
关键词
Fall detection,YOLOv3,Prone,Supine,Background illumination
AI 理解论文
溯源树
样例
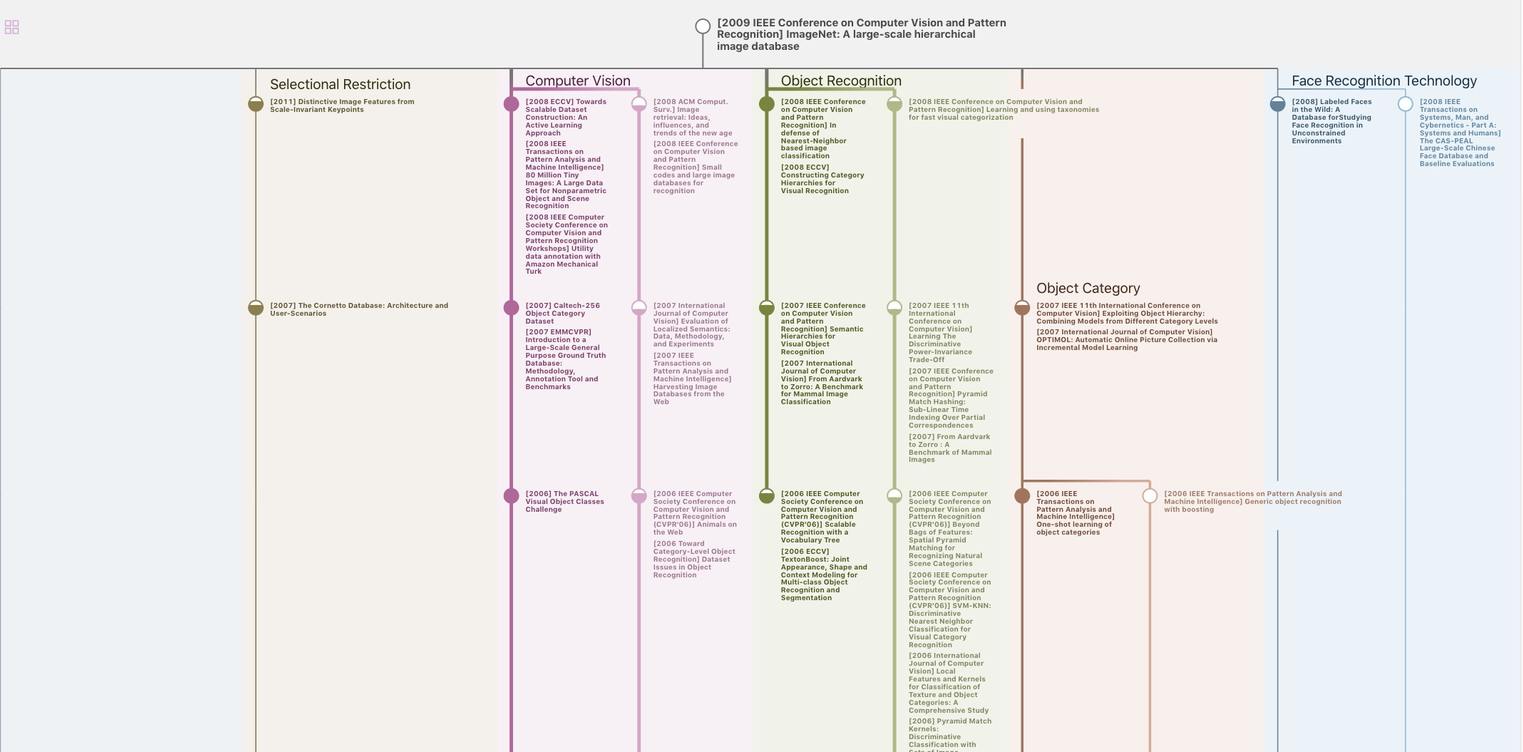
生成溯源树,研究论文发展脉络
Chat Paper
正在生成论文摘要