Evolving Dynamic Bayesian Networks for CO(2 )Emissions Forecasting in Multi-Source Power Generation Systems
IEEE Latin America Transactions(2023)
Abstract
Global warming is a significant challenge. Among the contributors, CO(2 )emission is the foremost, and almost 40% of global emissions come from electricity generation. In this sense, an accurate prediction of CO(2 )emissions in a multi-source system combining traditional and renewable sources can be used to support the reduction of carbon emissions without affecting the energy demand-supply. Despite the several relevant research in this topic, because of higher uncertainty and variability caused mainly by the intermittent nature of renewable energy, CO(2 )emissions forecasting in multi-source power generation systems is a current challenge. This paper presents CO(2 )emissions forecasting for multi-source power generation systems using evolving discrete Dynamic Bayesian Networks. Our proposal uses an analytical threshold for selecting directed edges by the occurrence frequency as data arrives, allowing a constant adaptation to smoothly converges into a robust forecast model. It was tested using real data from multi-source power generation systems of Belgium, Germany, Portugal, and Spain. Its performance was compared with other forecasting methods. Comparing the results against a traditional DBN that not evolves the structure over time, our proposal was superior highlighting a contribution of performance improvement. The proposed method was better when compared against ANN and XgBoost, with the difference in performance statistically significant.
MoreTranslated text
Key words
CO2 emissions forecasting, Energy Management and Sustainability, Dynamic Bayesian Networks, Multi-Source power generation system
AI Read Science
Must-Reading Tree
Example
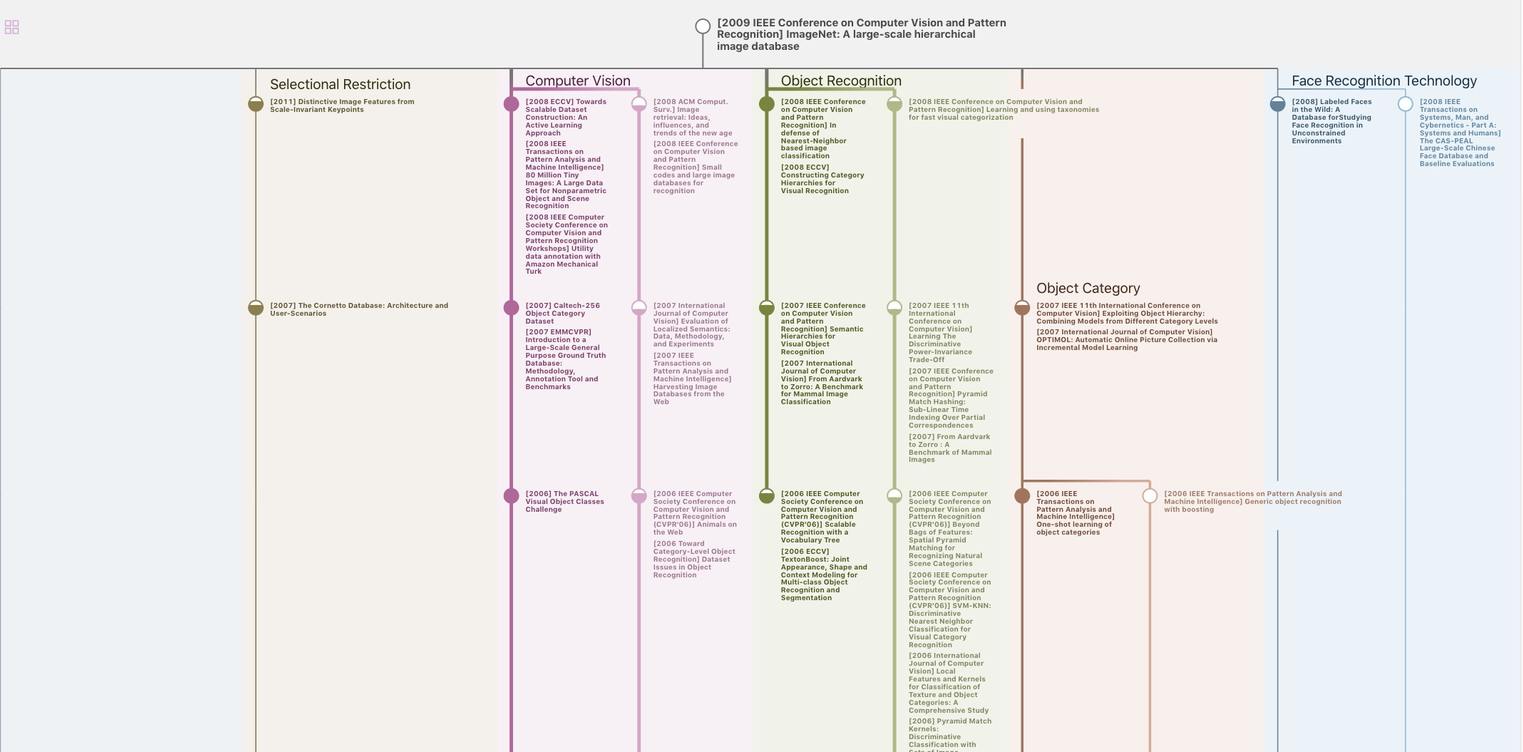
Generate MRT to find the research sequence of this paper
Chat Paper
Summary is being generated by the instructions you defined