Neural network-aided optimisation of a radio-frequency atomic magnetometer
OPTICS EXPRESS(2023)
摘要
Efficient unsupervised optimisation of atomic magnetometers is a requirement in many applications, where direct intervention of an operator is not feasible. The efficient extraction of the optimal operating conditions from a small sample of experimental data requires a robust automated regression of the available data. Here we address this issue and propose the use of general regression neural networks as a tool for the optimisation of atomic magnetometers which does not require human supervision and is efficient, as it is ideally suited to operating with a small sample of data as input. As a case study, we specifically demonstrate the optimisation of an unshielded radio-frequency atomic magnetometer by using a general regression neural network which establishes a mapping between three input variables, the cell temperature, the pump beam power and the probe beam power, and one output variable, the AC sensitivity. The optimisation root results into an AC sensitivity of 44 fT/ Hz at 26 kHz.Published by Optica Publishing Group under the terms of the Creative Commons Attribution 4.0 License. Further distribution of this work must maintain attribution to the author(s) and the published article's title, journal citation, and DOI.
更多查看译文
关键词
optimisation,network-aided,radio-frequency
AI 理解论文
溯源树
样例
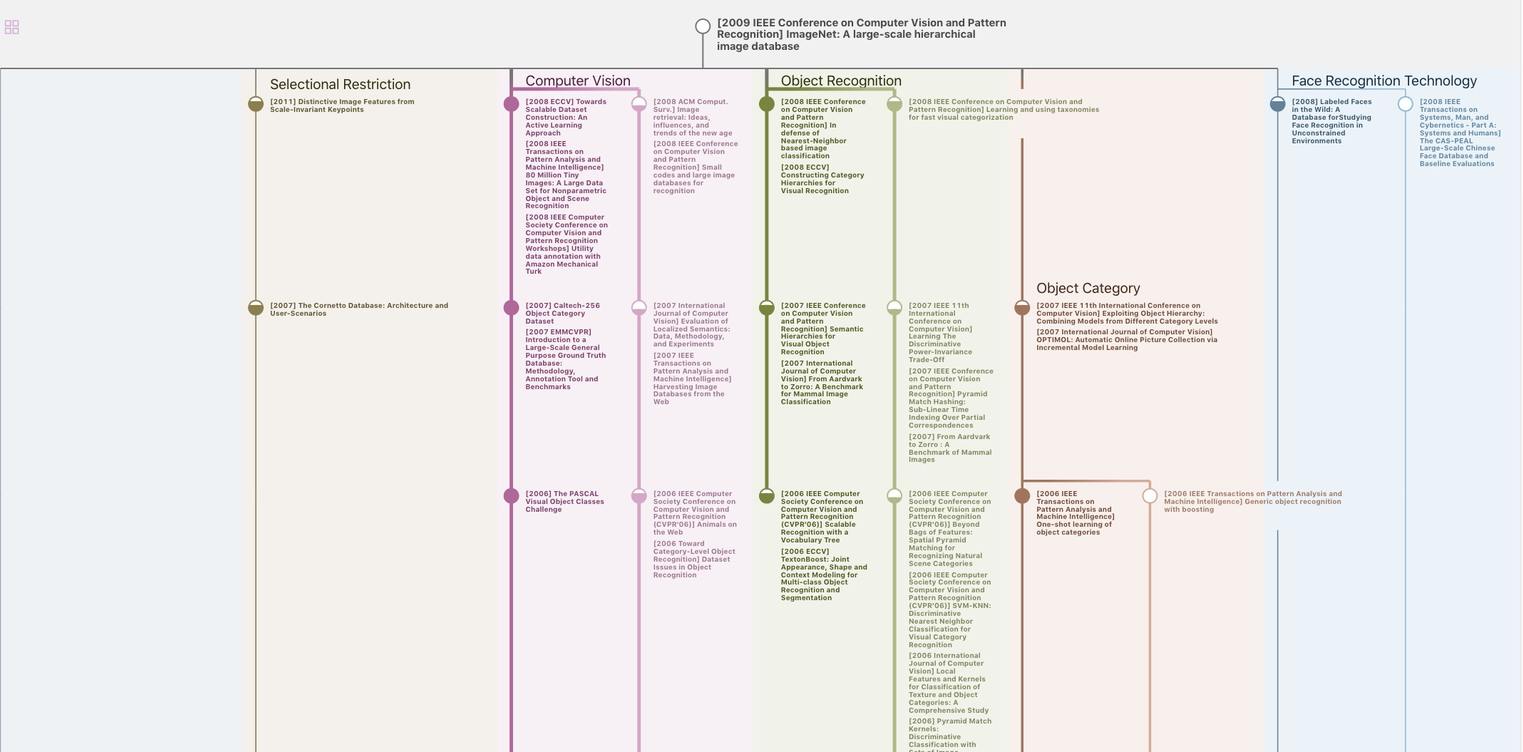
生成溯源树,研究论文发展脉络
Chat Paper
正在生成论文摘要