Efficient Community Search in Edge-Attributed Graphs
IEEE Transactions on Knowledge and Data Engineering(2023)
摘要
Given a graph, searching for a community containing a query vertex is a fundamental problem and has found many applications. Most existing community search models are based on non-attributed or vertex-attributed graphs. In many real-world graphs, however, the edges carry the richest information to describe the interactions between vertices; hence, it is important to take the information into account in community search. In this paper, we conduct a pioneer study on the community search on edge-attributed graphs. We proposed the Edge-Attributed Community Search (EACS) problem, which aims to extract a subgraph that contains the given query vertex while its edges have the maximum attribute similarity. We prove that the EACS problem is NP-hard and propose both exact and 2-approximation algorithms to address EACS. Our exact algorithms run up to 2320.34 times faster than the baseline solution. Our approximate algorithms further improve the efficiency by up to 2.93 times. We conducted extensive experiments to demonstrate the efficiency and effectiveness of our algorithms.
更多查看译文
关键词
graphs,community,search,edge-attributed
AI 理解论文
溯源树
样例
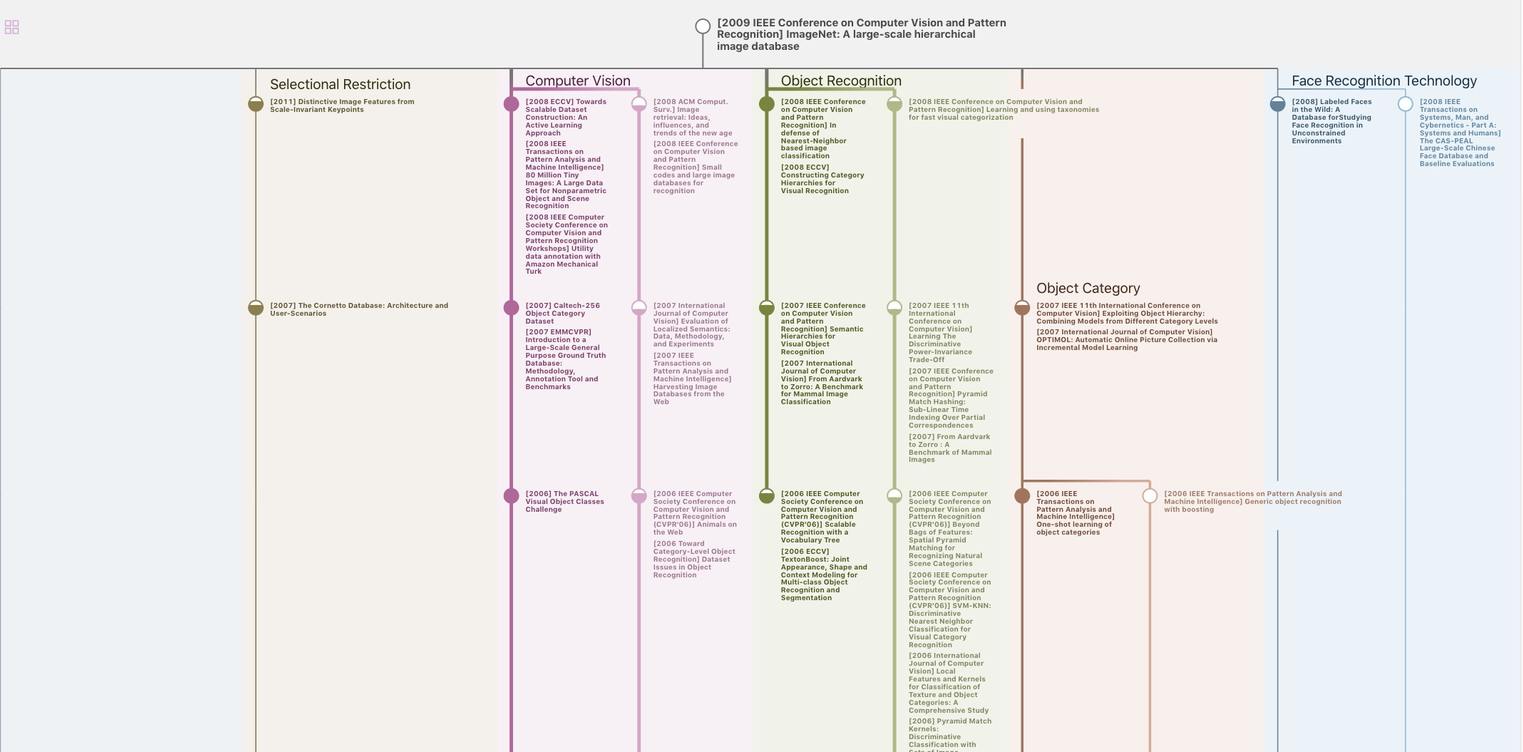
生成溯源树,研究论文发展脉络
Chat Paper
正在生成论文摘要