A Machine Learning Framework Reduces the Manual Workload for Systematic Reviews of the Diagnostic Performance of Prostate Magnetic Resonance Imaging
European Urology Open Science(2023)
Abstract
Prostate magnetic resonance imaging has become the imaging standard for prostate cancer in various clinical settings, with interpretation standardized according to the Prostate Imaging Reporting and Data System (PI-RADS). Each year, hundreds of scientific studies that report on the diagnostic performance of PI-RADS are published. To keep up with this ever-increasing evidence base, systematic reviews and meta-analyses are essential. As systematic reviews are highly resource-intensive, we investigated whether a machine learning framework can reduce the manual workload and speed up the screening process (title and abstract). We used search results from a living systematic review of the diagnostic performance of PI-RADS (1585 studies, of which 482 were potentially eligible after screening). A naïve Bayesian classifier was implemented in an active learning environment for classification of the titles and abstracts. Our outcome variable was the percentage of studies that can be excluded after 95% of relevant studies have been identified by the classifier (work saved over sampling: WSS@95%). In simulation runs of the entire screening process (controlling for classifier initiation and the frequency of classifier updating), we obtained a WSS@95% value of 28% (standard error of the mean ±0.1%). Applied prospectively, our classification framework would translate into a significant reduction in manual screening effort.
MoreTranslated text
Key words
Automation,Machine learning,Meta-analysis,Prostate magnetic resonance imaging,Systematic review,Prostate Imaging Reporting and Data System
AI Read Science
Must-Reading Tree
Example
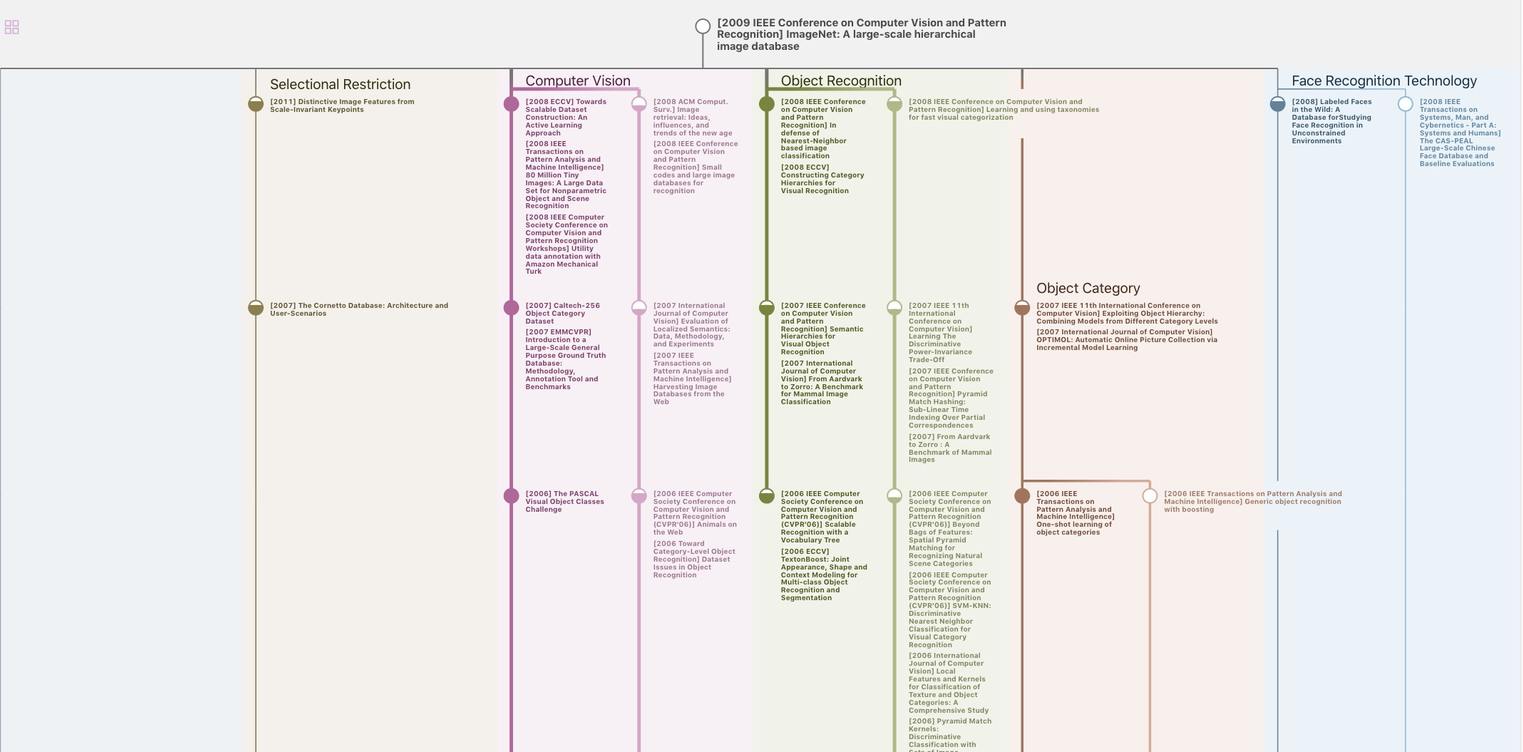
Generate MRT to find the research sequence of this paper
Chat Paper
Summary is being generated by the instructions you defined