Online Active Continual Learning for Robotic Lifelong Object Recognition.
IEEE transactions on neural networks and learning systems(2023)
摘要
In real-world applications, robotic systems collect vast amounts of new data from ever-changing environments over time. They need to continually interact and learn new knowledge from the external world to adapt to the environment. Particularly, lifelong object recognition in an online and interactive manner is a crucial and fundamental capability for robotic systems. To meet this realistic demand, in this article, we propose an online active continual learning (OACL) framework for robotic lifelong object recognition, in the scenario of both classes and domains changing with dynamic environments. First, to reduce the labeling cost as much as possible while maximizing the performance, a new online active learning (OAL) strategy is designed by taking both the uncertainty and diversity of samples into account to protect the information volume and distribution of data. In addition, to prevent catastrophic forgetting and reduce memory costs, a novel online continual learning (OCL) algorithm is proposed based on the deep feature semantic augmentation and a new loss-based deep model and replay buffer update, which can mitigate the class imbalance between the old and new classes and alleviate confusion between two similar classes. Moreover, the mistake bound of the proposed method is analyzed in theory. OACL allows robots to select the most representative new samples to query labels and continually learn new objects and new variants of previously learned objects from a nonindependent and identically distributed (i.i.d.) data stream without catastrophic forgetting. Extensive experiments conducted on real lifelong robotic vision datasets demonstrate that our algorithm, even trained with fewer labeled samples and replay exemplars, can achieve state-of-the-art performance on OCL tasks.
更多查看译文
关键词
Catastrophic forgetting,lifelong object recognition,online active learning (OAL),online continual learning (OCL)
AI 理解论文
溯源树
样例
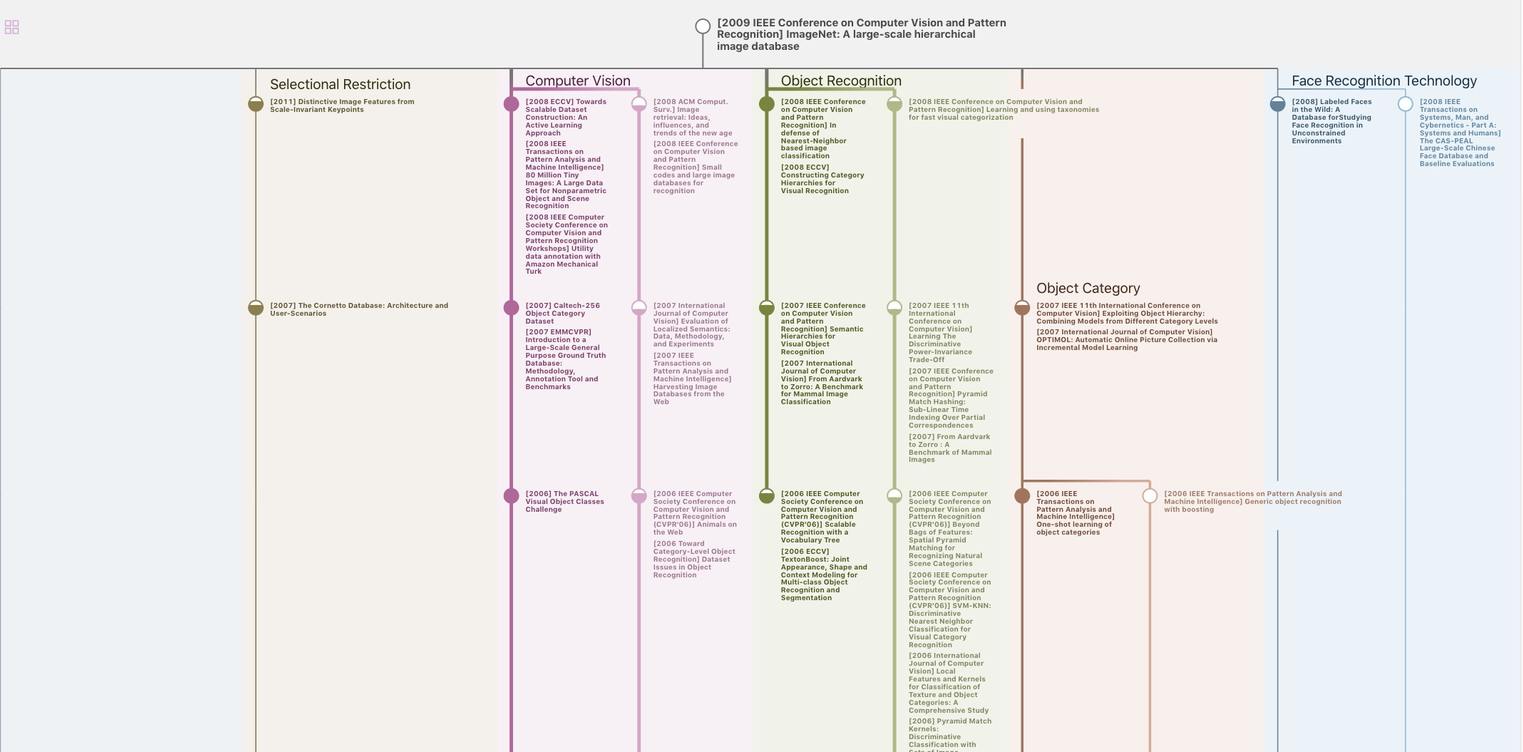
生成溯源树,研究论文发展脉络
Chat Paper
正在生成论文摘要