Gradient Dynamics in Linear Quadratic Network Games with Time-Varying Connectivity and Population Fluctuation
2023 62ND IEEE CONFERENCE ON DECISION AND CONTROL, CDC(2023)
摘要
In this paper, we consider a learning problem among non-cooperative agents interacting in a time-varying system. Specifically, we focus on repeated linear quadratic network games, in which the network of interactions changes with time and agents may not be present at each iteration. To get tractability, we assume that at each iteration, the network of interactions is sampled from an underlying random network model and agents participate at random with a given probability. Under these assumptions, we consider a gradient-based learning algorithm and establish almost sure convergence of the agents' strategies to the Nash equilibrium of the game played over the expected network. Additionally, we prove, in the large population regime, that the learned strategy is an epsilon-Nash equilibrium for each stage game with high probability. We validate our results over an online market application.
更多查看译文
AI 理解论文
溯源树
样例
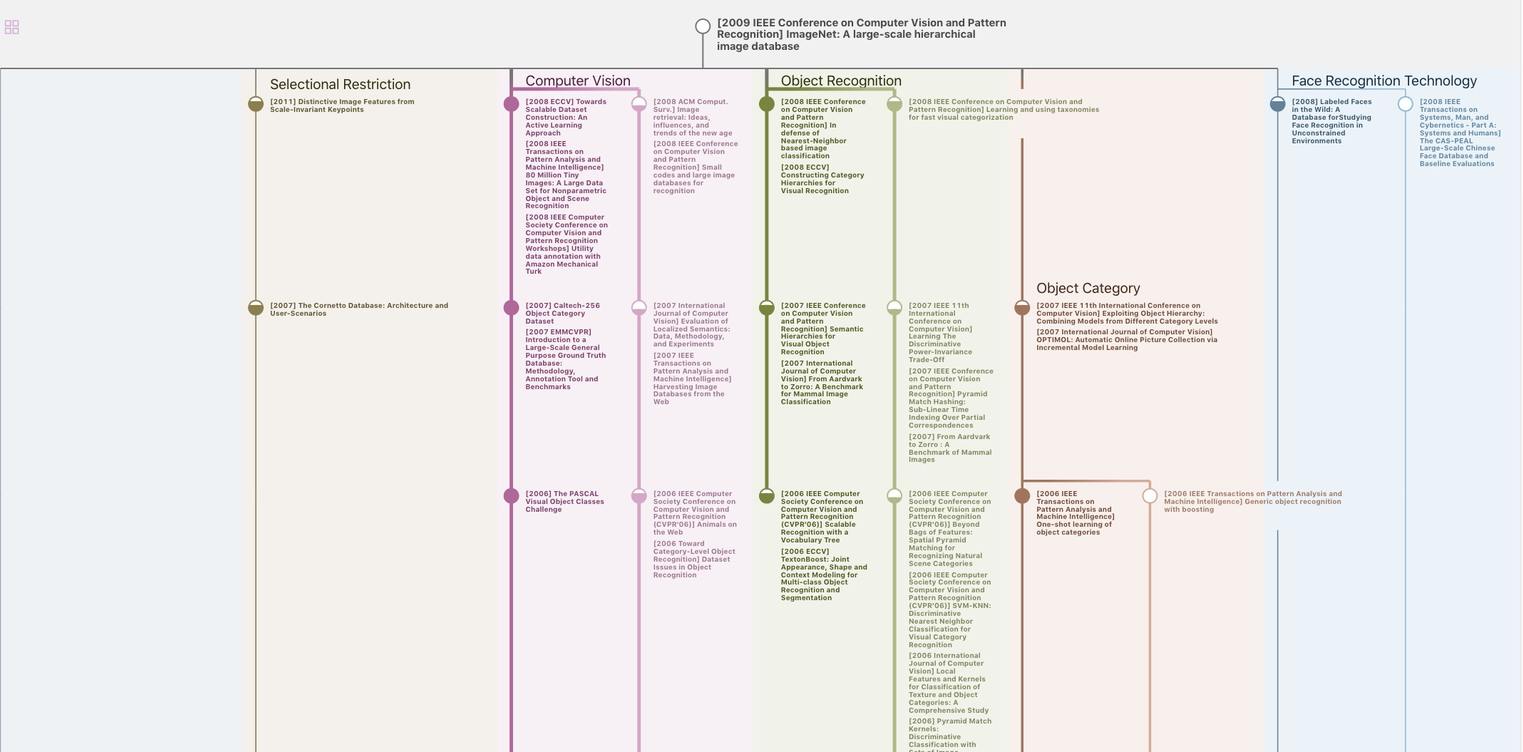
生成溯源树,研究论文发展脉络
Chat Paper
正在生成论文摘要