Detecting Misinformation with LLM-Predicted Credibility Signals and Weak Supervision
CoRR(2023)
摘要
Credibility signals represent a wide range of heuristics that are typically used by journalists and fact-checkers to assess the veracity of online content. Automating the task of credibility signal extraction, however, is very challenging as it requires high-accuracy signal-specific extractors to be trained, while there are currently no sufficiently large datasets annotated with all credibility signals. This paper investigates whether large language models (LLMs) can be prompted effectively with a set of 18 credibility signals to produce weak labels for each signal. We then aggregate these potentially noisy labels using weak supervision in order to predict content veracity. We demonstrate that our approach, which combines zero-shot LLM credibility signal labeling and weak supervision, outperforms state-of-the-art classifiers on two misinformation datasets without using any ground-truth labels for training. We also analyse the contribution of the individual credibility signals towards predicting content veracity, which provides new valuable insights into their role in misinformation detection.
更多查看译文
关键词
credibility,misinformation,supervision,llm-predicted
AI 理解论文
溯源树
样例
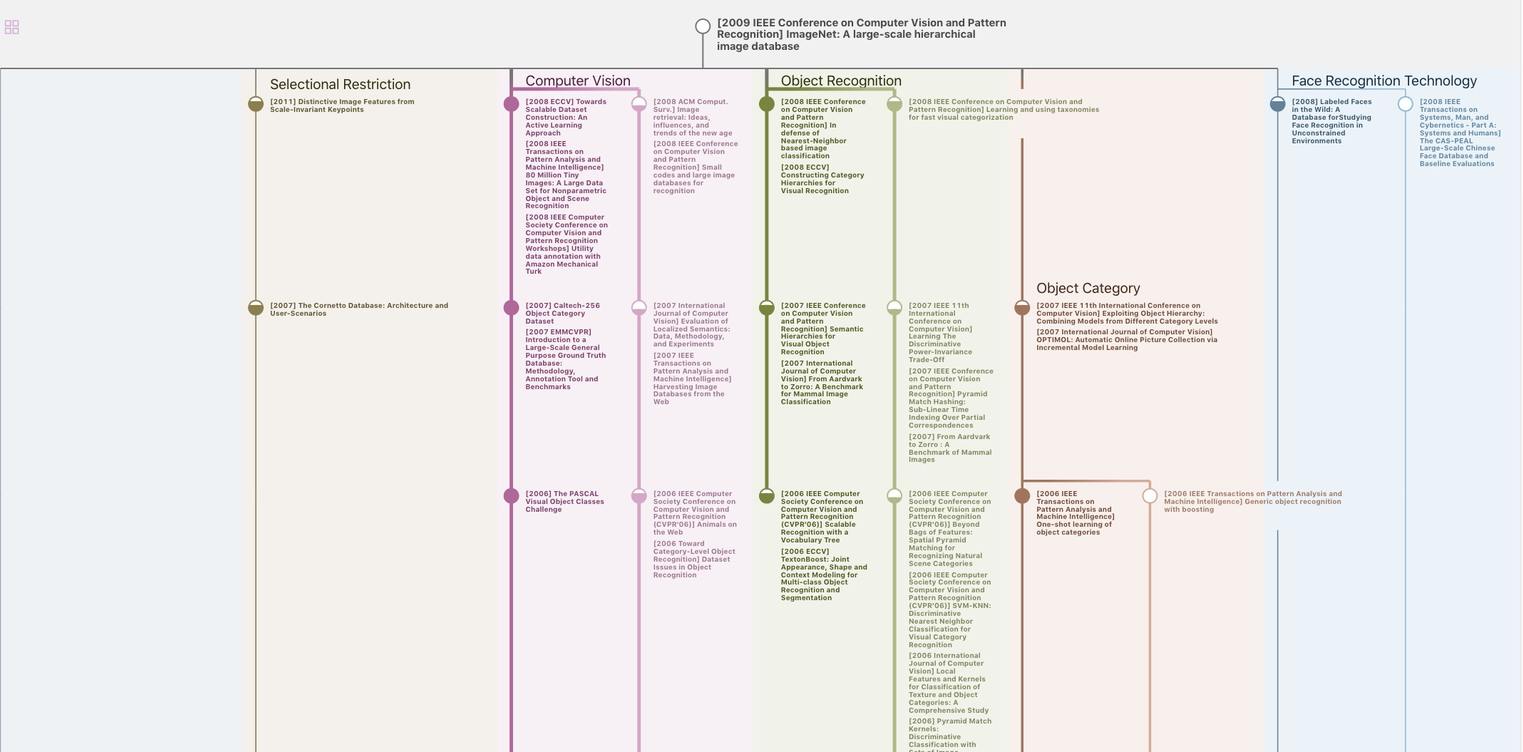
生成溯源树,研究论文发展脉络
Chat Paper
正在生成论文摘要