Structure-Preserving Transformers for Sequences of SPD Matrices
CoRR(2023)
摘要
In recent years, Transformer-based auto-attention mechanisms have been successfully applied to the analysis of a variety of context-reliant data types, from texts to images and beyond, including data from non-Euclidean geometries. In this paper, we present such a mechanism, designed to classify sequences of Symmetric Positive Definite matrices while preserving their Riemannian geometry throughout the analysis. We apply our method to automatic sleep staging on timeseries of EEG-derived covariance matrices from a standard dataset, obtaining high levels of stage-wise performance.
更多查看译文
关键词
spd matrices,structure-preserving
AI 理解论文
溯源树
样例
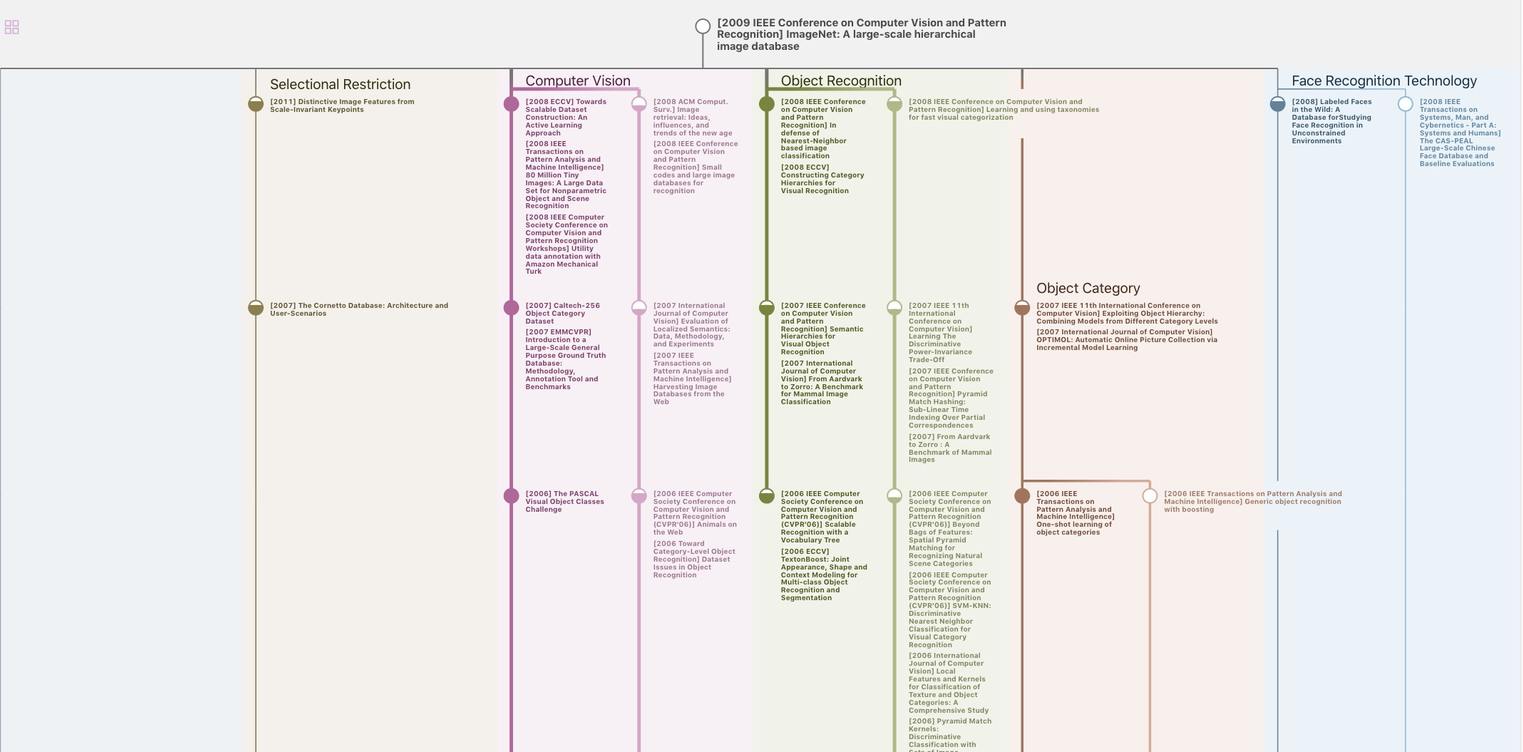
生成溯源树,研究论文发展脉络
Chat Paper
正在生成论文摘要