RecycleNet: Latent Feature Recycling Leads to Iterative Decision Refinement
Bildverarbeitung für die Medizin(2023)
摘要
Despite the remarkable success of deep learning systems over the last decade, a key difference still remains between neural network and human decision-making: As humans, we cannot only form a decision on the spot, but also ponder, revisiting an initial guess from different angles, distilling relevant information, arriving at a better decision. Here, we propose RecycleNet, a latent feature recycling method, instilling the pondering capability for neural networks to refine initial decisions over a number of recycling steps, where outputs are fed back into earlier network layers in an iterative fashion. This approach makes minimal assumptions about the neural network architecture and thus can be implemented in a wide variety of contexts. Using medical image segmentation as the evaluation environment, we show that latent feature recycling enables the network to iteratively refine initial predictions even beyond the iterations seen during training, converging towards an improved decision. We evaluate this across a variety of segmentation benchmarks and show consistent improvements even compared with top-performing segmentation methods. This allows trading increased computation time for improved performance, which can be beneficial, especially for safety-critical applications.
更多查看译文
关键词
Algorithms,Image recognition and understanding,Algorithms,Machine learning architectures,formulations,and algorithms,Applications,Biomedical / healthcare / medicine
AI 理解论文
溯源树
样例
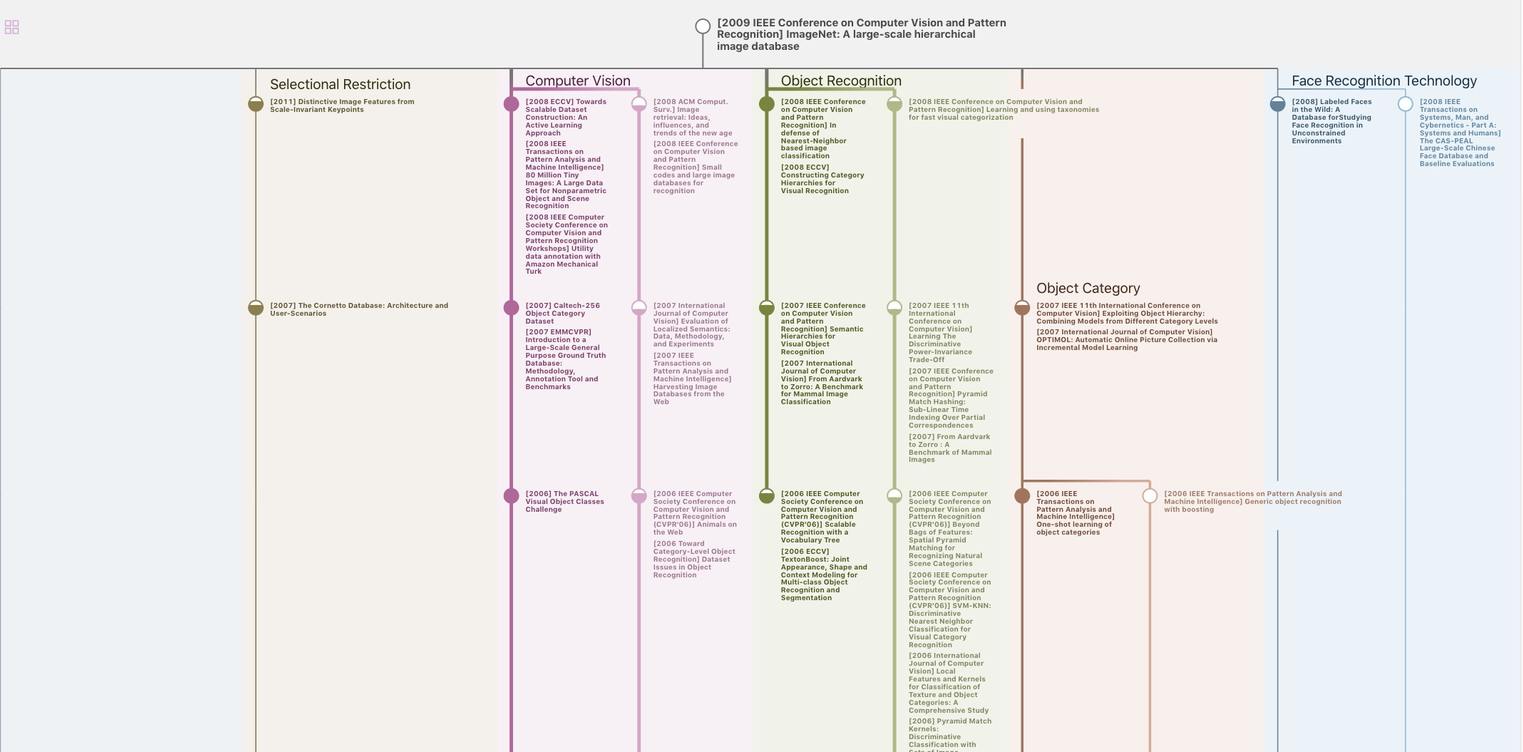
生成溯源树,研究论文发展脉络
Chat Paper
正在生成论文摘要