Stable In-hand Manipulation with Finger Specific Multi-agent Shadow Reward
CoRR(2023)
摘要
Deep Reinforcement Learning has shown its capability to solve the high degrees of freedom in control and the complex interaction with the object in the multi-finger dexterous in-hand manipulation tasks. Current DRL approaches prefer sparse rewards to dense rewards for the ease of training but lack behavior constraints during the manipulation process, leading to aggressive and unstable policies that are insufficient for safety-critical in-hand manipulation tasks. Dense rewards can regulate the policy to learn stable manipulation behaviors with continuous reward constraints but are hard to empirically define and slow to converge optimally. This work proposes the Finger-specific Multi-agent Shadow Reward (FMSR) method to determine the stable manipulation constraints in the form of dense reward based on the state-action occupancy measure, a general utility of DRL that is approximated during the learning process. Information Sharing (IS) across neighboring agents enables consensus training to accelerate the convergence. The methods are evaluated in two in-hand manipulation tasks on the Shadow Hand. The results show FMSR+IS converges faster in training, achieving a higher task success rate and better manipulation stability than conventional dense reward. The comparison indicates FMSR+IS achieves a comparable success rate even with the behavior constraint but much better manipulation stability than the policy trained with a sparse reward.
更多查看译文
关键词
manipulation,shadow
AI 理解论文
溯源树
样例
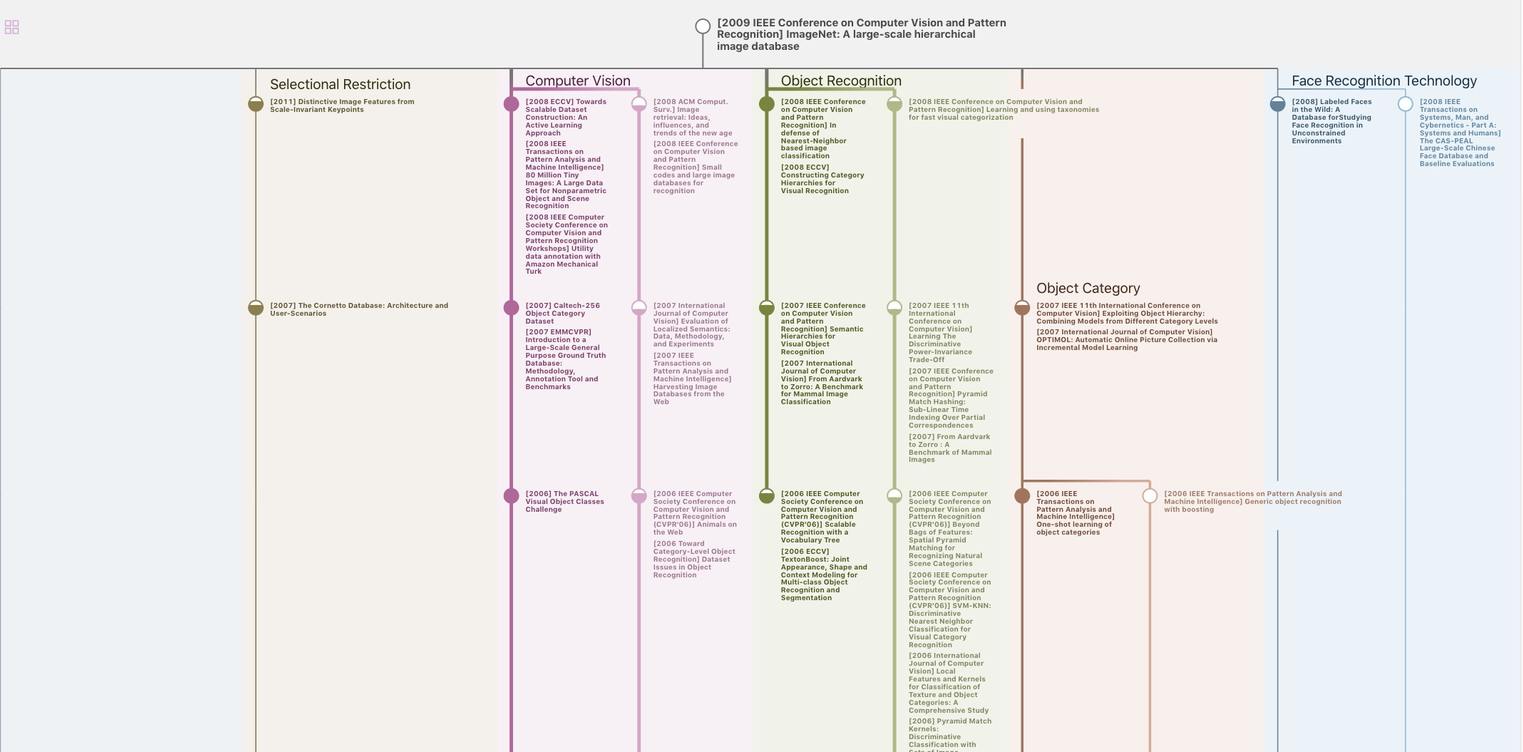
生成溯源树,研究论文发展脉络
Chat Paper
正在生成论文摘要