A Risk Prediction Model Based on Crash History Data for Railway Trams
CHINESE JOURNAL OF ELECTRONICS(2023)
Abstract
Risk prediction is an important task to ensuring the driving safety of railway trams. Although data-driven intelligent methods are proved to be effective for driving risk prediction, accuracy is still a top concern for the challenges of data quality which mainly represent as the unbalanced datasets. This study focuses on applying feature extraction and data augmentation methods to achieve effective risk prediction for railway trams, and proposes an approach based on a self-adaptive K- means clustering algorithm and the least squares deep convolution generative adversarial network (LS-DCGAN). The data preprocessing methods are proposed, which include the K- means algorithm to cluster the locations of trams and the extreme gradient boosting recursive feature elimination based feature selection algorithm to retain the key features. The LS-DCGAN model is designed for sparse sample expansion, aiming to address the sample category distribution imbalance problem. The experiments implemented with the public and real datasets show that the proposed approach can reach a high accuracy of 90.69%, which can greatly enhances the tram driving safety.
MoreTranslated text
Key words
Intelligent transportation,Railway tram,Driving risk prediction,Data augmentation
AI Read Science
Must-Reading Tree
Example
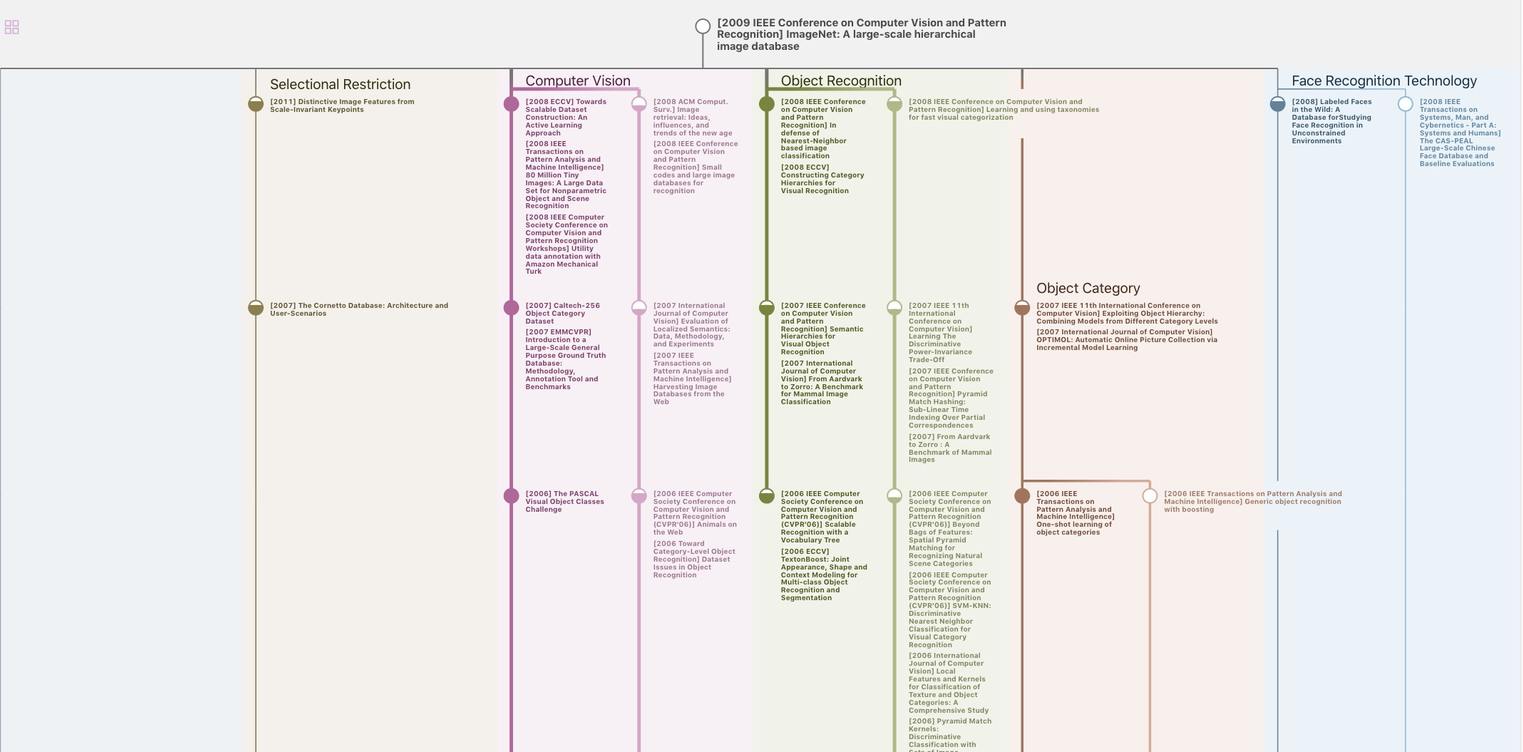
Generate MRT to find the research sequence of this paper
Chat Paper
Summary is being generated by the instructions you defined