Comparative analysis of five convolutional neural networks for landslide susceptibility assessment
BULLETIN OF ENGINEERING GEOLOGY AND THE ENVIRONMENT(2023)
摘要
To evaluate the performance of deep learning methods on the landslide susceptibility mapping, five different convolutional neural networks (CNN)—AlexNet, Inception-v3, Xception, ResNet-101, and DenseNet-201—were employed to predict the landslide susceptibility along a transmission line. Ten landslide influencing factors were extracted from three databases and considered in the input layers. The landslide (10,481 grids) and non-landslide (10,481 grids) data were randomly subdivided into 70% (14,673 grids) and 30% (6289 grids) to construct the training and validation samples, respectively. The appropriate architecture and training parameters were carefully selected after many attempts until the training and validation accuracy was above 90%. The receiver operating characteristic (ROC) curve, landslide density (LD), and landslide ratio (LR) were determined to estimate the five CNN networks’ prediction accuracy. All CNN networks had high area-under-the-curve (AUC) values when assessing landslide susceptibility, and most landslides occurred in the outputs with predicted high and very high landslide susceptibility ( LD > 65% and LR > 2.90). Generally, CNN networks had a higher accuracy than the two traditional methods due to the powerful capability of deep feature extraction. Additionally, the computational time cost in three steps was recorded to investigate the efficiency of five CNN networks, and all CNN networks accomplished the goals within an acceptable time using a commercially available computer (~ 24 h). Comparatively, AlexNet and Xception had better performance than other networks on the landslide susceptibility assessment.
更多查看译文
关键词
Landslide susceptibility,Deep learning,Convolutional neural network,Comparative analysis
AI 理解论文
溯源树
样例
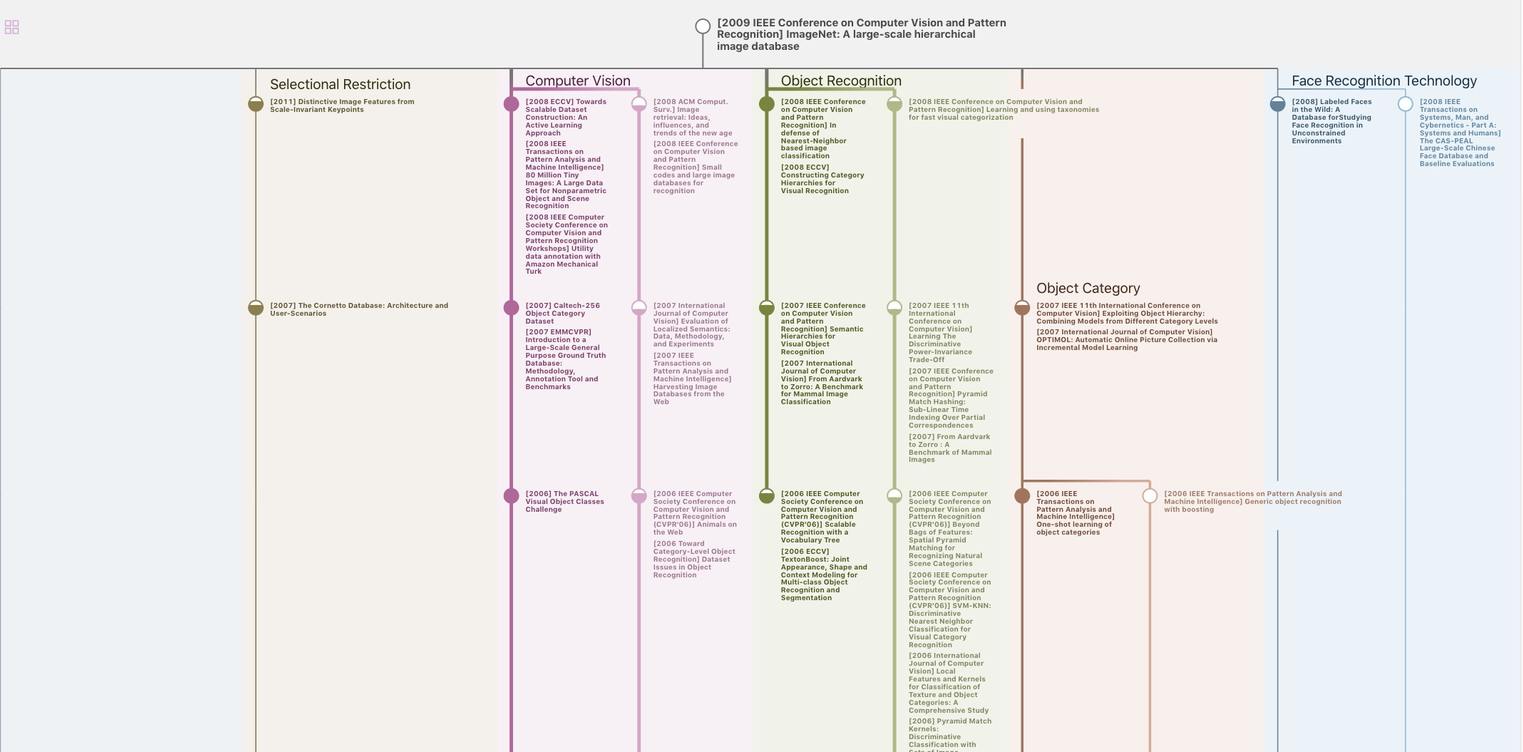
生成溯源树,研究论文发展脉络
Chat Paper
正在生成论文摘要