Noninvasive grading of glioma brain tumors using magnetic resonance imaging and deep learning methods
Journal of Cancer Research and Clinical Oncology(2023)
摘要
Purpose Convolutional Neural Networks (ConvNets) have quickly become popular machine learning techniques in recent years, particularly in the classification and segmentation of medical images . One of the most prevalent types of brain cancers is glioma, and early, accurate diagnosis is essential for both treatment and survival. In this study, MRI scans were examined utilizing deep learning techniques to examine glioma diagnosis studies. Methods In this systematic review, keywords were used to obtain English-language studies from the Arxiv, IEEE, Springer, ScienceDirect, and PubMed databases for the years 2010–2022. The material needed for review was then collected from the articles once they had been chosen based on the entry and exit criteria and in accordance with the research's goal. Results Finally, 77 different academic articles were chosen. According to a study of published articles, glioma brain tumors were discovered, categorized, and segmented utilizing a coordinated approach that included image collecting, pre-processing, model design and execution, and model output evaluation. The majority of investigations have used publicly accessible photo databases and already-trained algorithms. The bulk of studies have employed Dice's classification accuracy and similarity coefficient metrics to assess model performance. Conclusion The results of this study indicate that glioma segmentation has received more attention from researchers than glioma detection and classification. It is advised that more research be done in the areas of glioma detection and, particularly, grading in order to be included in systems that support medical diagnosis.
更多查看译文
关键词
glioma brain tumors,noninvasive grading,magnetic resonance imaging,deep learning
AI 理解论文
溯源树
样例
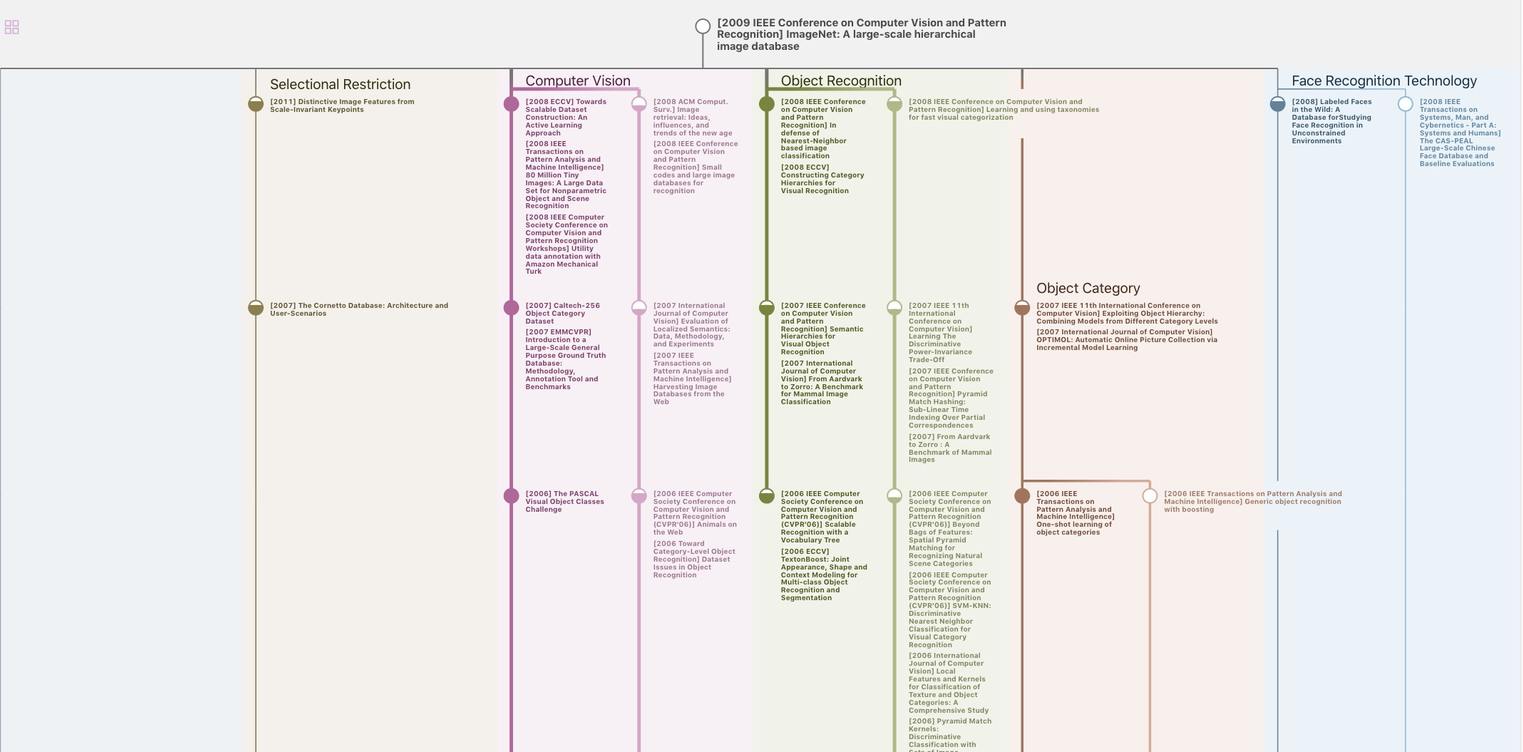
生成溯源树,研究论文发展脉络
Chat Paper
正在生成论文摘要