Deep Cascade-Learning Model via Recurrent Attention for Immunofixation Electrophoresis Image Analysis
IEEE TRANSACTIONS ON MEDICAL IMAGING(2023)
摘要
Immunofixation Electrophoresis (IFE) analysis has been an indispensable prerequisite for the diagnosis of M-protein, which is an important criterion to recognize diversified plasma cell diseases. Existing intelligent methods of IFE diagnosis commonly employ a single unified classifier to directly classify whether M-protein exists and which isotype of M-protein is. However, this unified classification is not optimal because the two tasks have different characteristics and require different feature extraction techniques. Classifying the M-protein existence depends on the presence or absence of dense bands in IFE data, while classifying the M-protein isotype depends on the location of dense bands. Consequently, a cascading two-classifier framework suitable to the two tasks respectively may achieve better performance. In this paper, we propose a novel deep cascade-learning model, which sequentially integrates a positive-negative classifier based on deep collocative learning and an isotype classifier based on recurrent attention model to address these two tasks respectively. Specifically, the attention mechanism can mimic the visual perception of clinicians, where only the most informative local regions are extracted through sequential partial observations. This not only avoids the interference of redundant regions but also saves computational power. Further, domain knowledge about SP lane and heavy-light-chain lanes is also introduced to assist our attention location. Extensive numerical experiments show that our deep cascade-learning outperforms state-of-the-art methods on recognized evaluation metrics and can effectively capture the co-location of dense bands in different lanes.
更多查看译文
关键词
Immunofixation electrophoresis,deep cascade-learning,recurrent attention mechanism
AI 理解论文
溯源树
样例
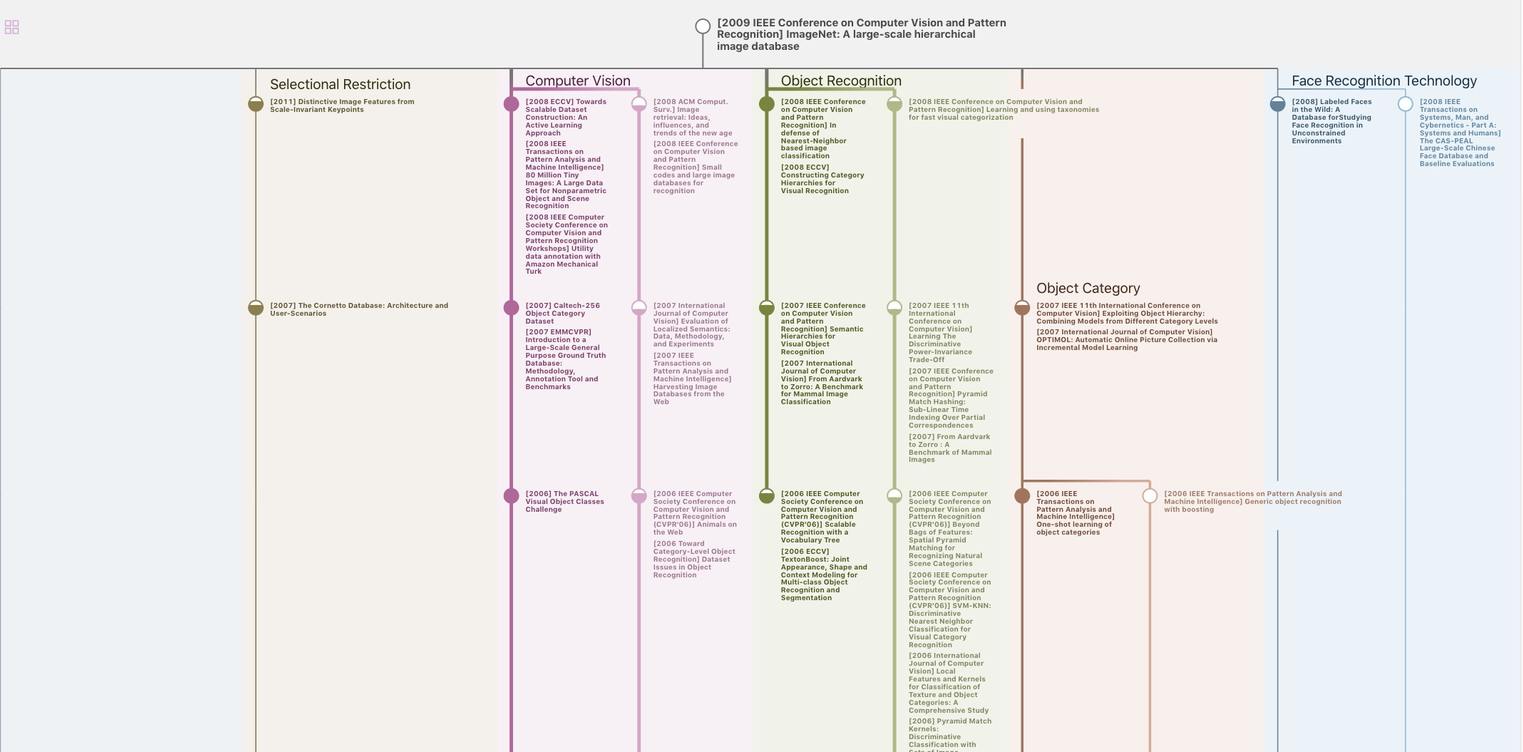
生成溯源树,研究论文发展脉络
Chat Paper
正在生成论文摘要