Neural network-based coronary dominance classification of RCA angiograms
CoRR(2023)
摘要
Background. Cardiac dominance classification is essential for SYNTAX score estimation, which is a tool used to determine the complexity of coronary artery disease and guide patient selection toward optimal revascularization strategy. Objectives. Cardiac dominance classification algorithm based on the analysis of right coronary artery (RCA) angiograms using neural network Method. We employed convolutional neural network ConvNext and Swin transformer for 2D image (frames) classification, along with a majority vote for cardio angiographic view classification. An auxiliary network was also used to detect irrelevant images which were then excluded from the data set. Our data set consisted of 828 angiographic studies, 192 of them being patients with left dominance. Results. 5-fold cross validation gave the following dominance classification metrics (p=95%): macro recall=93.1%, accuracy=93.5%, macro F1=89.2%. The most common case in which the model regularly failed was RCA occlusion, as it requires utilization of LCA information. Another cause for false prediction is a small diameter combined with poor quality cardio angiographic view. In such cases, cardiac dominance classification can be complex and may require discussion among specialists to reach an accurate conclusion. Conclusion. The use of machine learning approaches to classify cardiac dominance based on RCA alone has been shown to be successful with satisfactory accuracy. However, for higher accuracy, it is necessary to utilize LCA information in the case of an occluded RCA and detect cases where there is high uncertainty.
更多查看译文
关键词
coronary dominance classification,rca angiograms,network-based
AI 理解论文
溯源树
样例
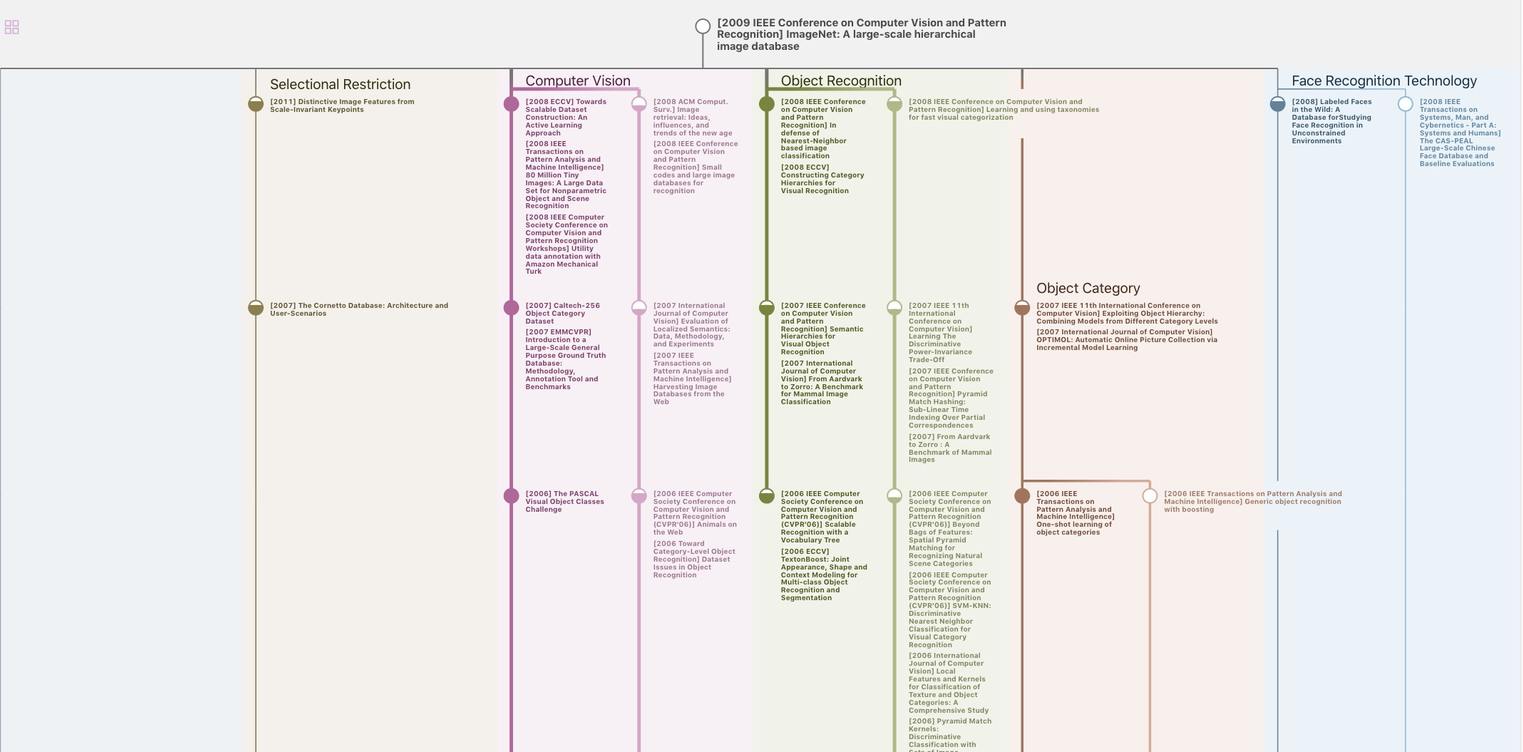
生成溯源树,研究论文发展脉络
Chat Paper
正在生成论文摘要