Electricity Demand Forecasting through Natural Language Processing with Long Short-Term Memory Networks
2023 IEEE PES Innovative Smart Grid Technologies Europe (ISGT EUROPE)(2023)
摘要
Electricity demand forecasting is a well established research field. Usually this task is performed considering historical loads, weather forecasts, calendar information and known major events. Recently attention has been given on the possible use of new sources of information from textual news in order to improve the performance of these predictions. This paper proposes a Long and Short-Term Memory (LSTM) network incorporating textual news features that successfully predicts the deterministic and probabilistic tasks of the UK national electricity demand. The study finds that public sentiment and word vector representations related to transport and geopolitics have time-continuity effects on electricity demand. The experimental results show that the LSTM with textual features improves by more than 3% compared to the pure LSTM benchmark and by close to 10% over the official benchmark. Furthermore, the proposed model effectively reduces forecasting uncertainty by narrowing the confidence interval and bringing the forecast distribution closer to the truth.
更多查看译文
关键词
Electricity demand forecasting,LSTM network,natural language processing,smart grids,probabilistic forecasting
AI 理解论文
溯源树
样例
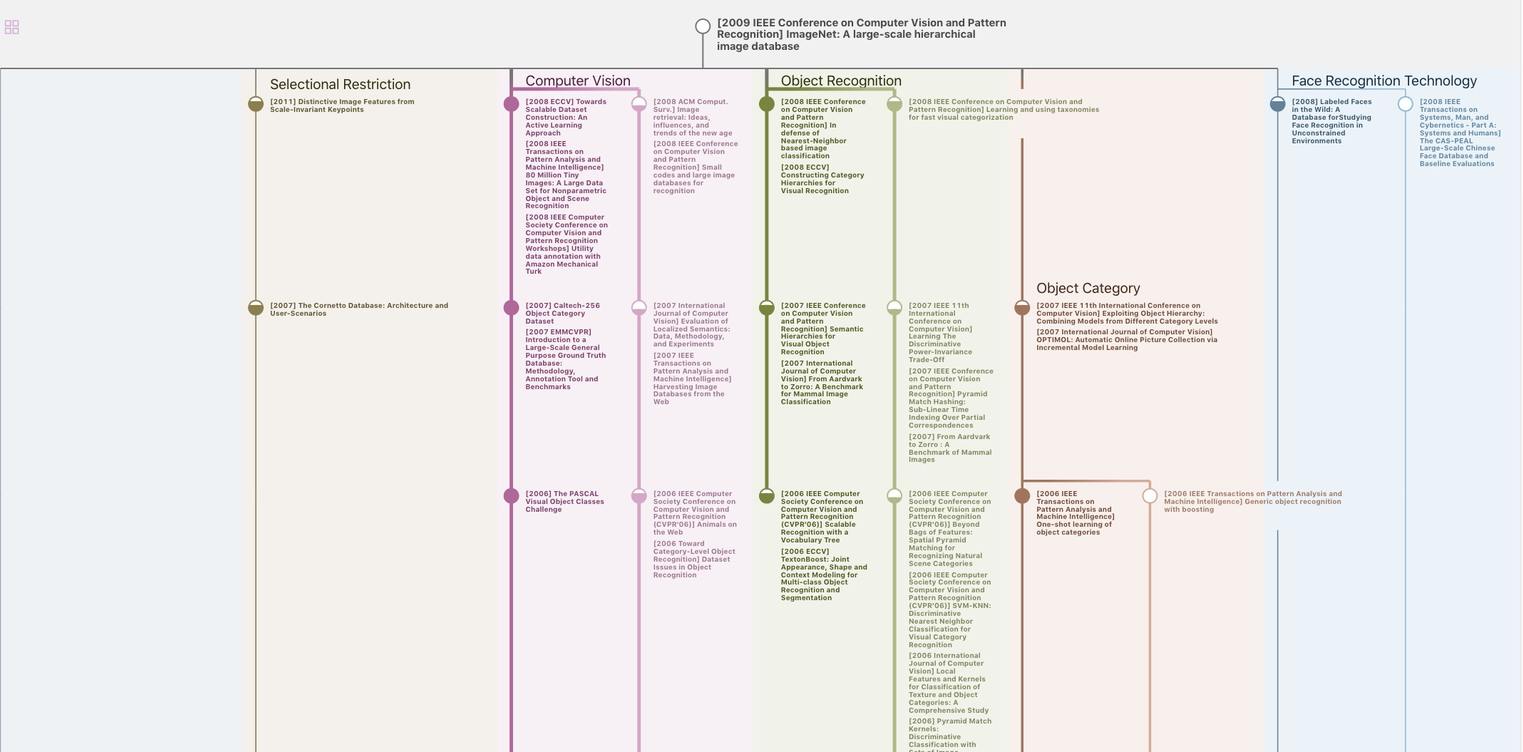
生成溯源树,研究论文发展脉络
Chat Paper
正在生成论文摘要