Generalizable Neural Fields as Partially Observed Neural Processes
2023 IEEE/CVF International Conference on Computer Vision (ICCV)(2023)
Abstract
Neural fields, which represent signals as a function parameterized by a neural network, are a promising alternative to traditional discrete vector or grid-based representations. Compared to discrete representations, neural representations both scale well with increasing resolution, are continuous, and can be many-times differentiable. However, given a dataset of signals that we would like to represent, having to optimize a separate neural field for each signal is inefficient, and cannot capitalize on shared information or structures among signals. Existing generalization methods view this as a meta-learning problem and employ gradient-based meta-learning to learn an initialization which is then fine-tuned with test-time optimization, or learn hypernetworks to produce the weights of a neural field. We instead propose a new paradigm that views the large-scale training of neural representations as a part of a partially-observed neural process framework, and leverage neural process algorithms to solve this task. We demonstrate that this approach outperforms both state-of-the-art gradient-based meta-learning approaches and hypernetwork approaches.
MoreTranslated text
Key words
generalizable neural fields,observed neural processes
AI Read Science
Must-Reading Tree
Example
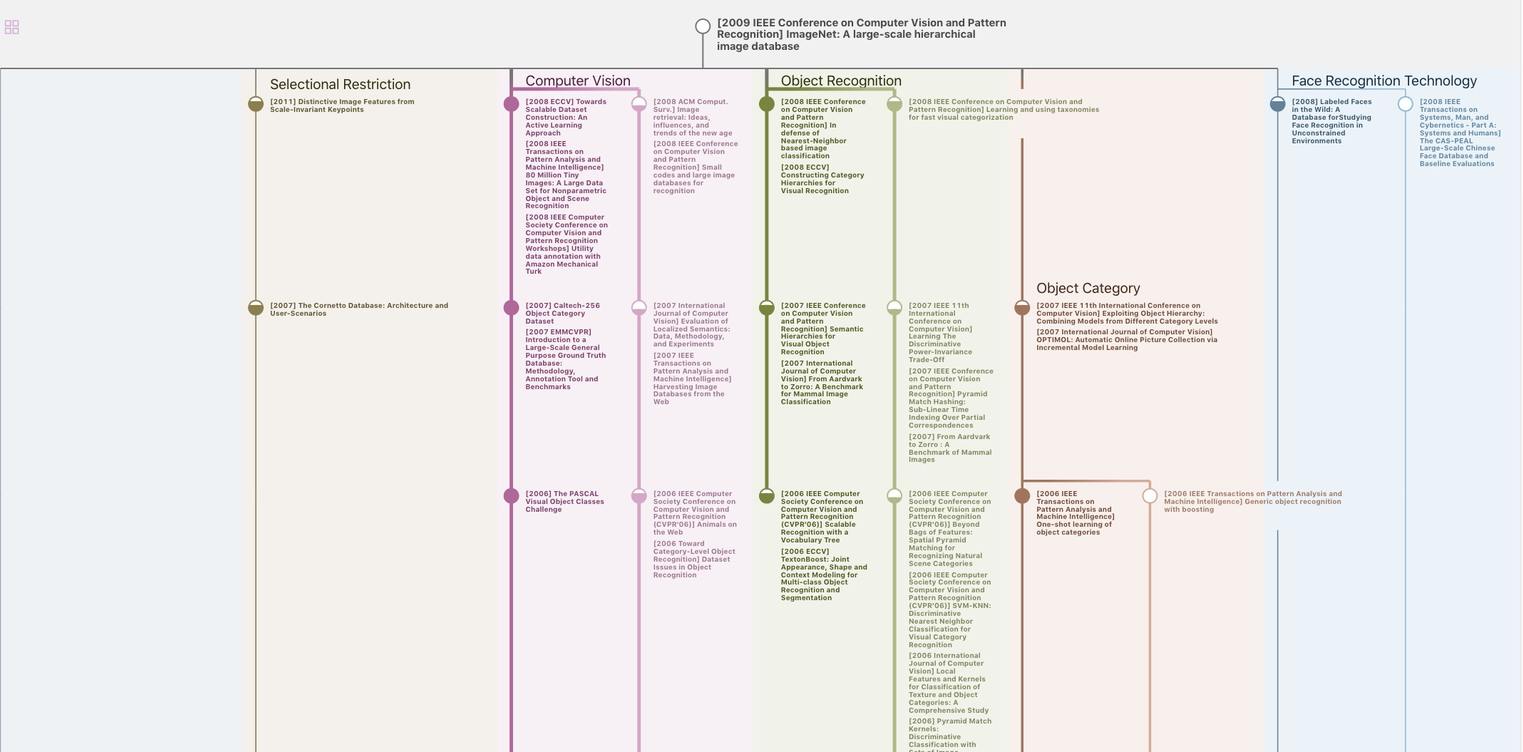
Generate MRT to find the research sequence of this paper
Chat Paper
Summary is being generated by the instructions you defined