Epistemic modeling uncertainty of rapid neural network ensembles for adaptive learning
FINITE ELEMENTS IN ANALYSIS AND DESIGN(2024)
摘要
Emulator embedded neural networks, which are closely related to physics informed neural networks, leverage multi-fidelity data sources for efficient design exploration of aerospace engineering systems. Multiple realizations of the neural network model are trained with different random initializations. The ensemble of model realizations is used to assess epistemic modeling uncertainty caused by a lack of training samples. This uncertainty estimation is crucial information for successful goal-oriented adaptive learning in an aerospace system design exploration. However, the costs of training the ensemble models often become prohibitive and pose a computational challenge, especially when the models are not trained in parallel during adaptive learning. In this work, a new type of emulator embedded neural network is presented using the rapid neural network paradigm. Unlike the conventional neural network training that optimizes the weights and biases of all the network layers by using gradient-based backpropagation, rapid neural network training adjusts only the last layer connection weights by applying a linear regression technique. It is found that the proposed emulator embedded neural network trains near-instantaneously, typically without loss of prediction accuracy. The proposed method is demonstrated on multiple analytical examples, as well as an aerospace flight parameter study of a generic hypersonic vehicle.
更多查看译文
关键词
Machine learning,Neural network,Multifidelity,Active learning,Aircraft design
AI 理解论文
溯源树
样例
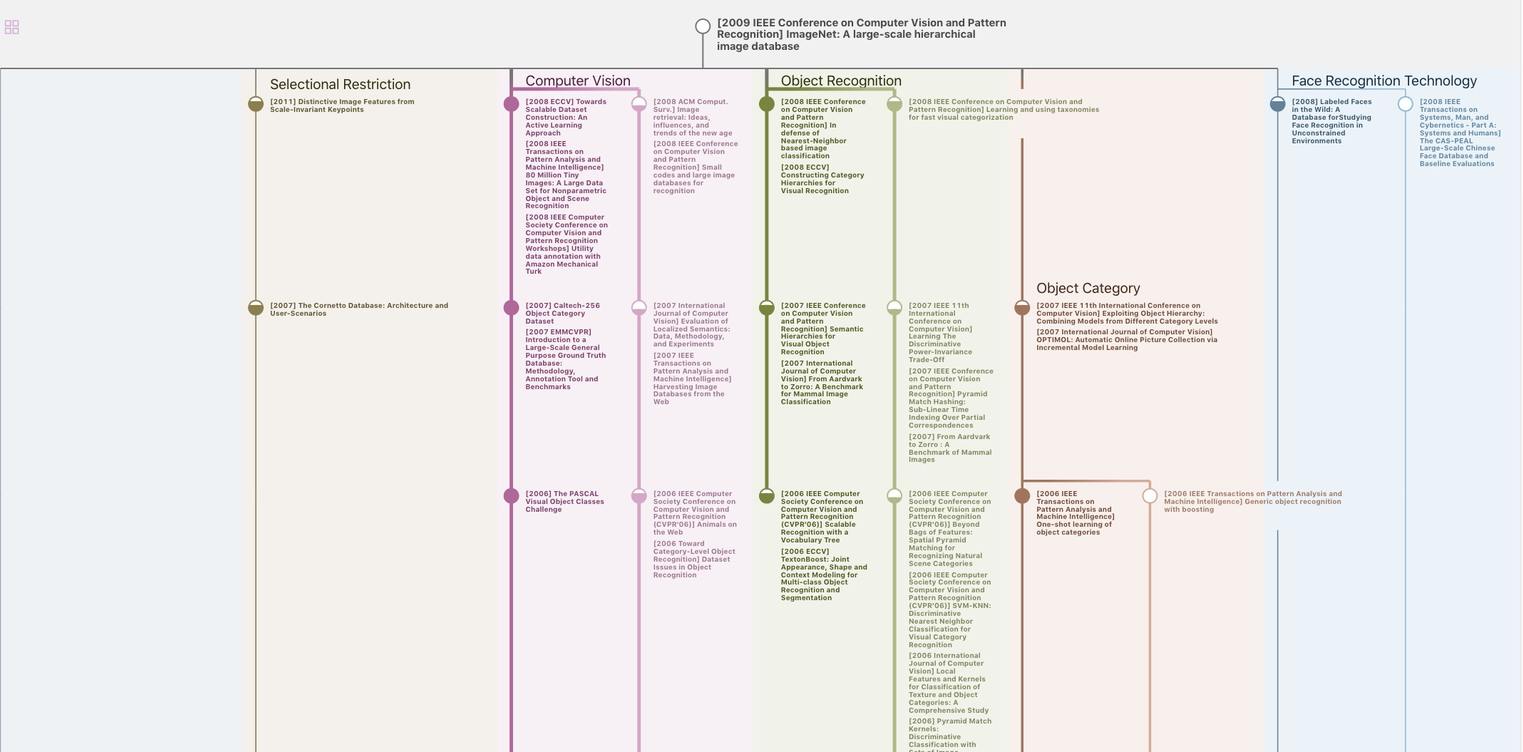
生成溯源树,研究论文发展脉络
Chat Paper
正在生成论文摘要