SSRL: A Safe and Smooth Reinforcement Learning Approach for Collision Avoidance in Navigation
2023 2nd Conference on Fully Actuated System Theory and Applications (CFASTA)(2023)
Abstract
This paper addresses the collision avoidance problem of autonomous robots using reinforcement learning (RL) techniques, with a focus on ensuring both safety and smoothness. To achieve stability guarantees, a data-based Lyapunov function is incorporated into the model-free RL framework for training policies. Furthermore, constraints on action increments and action distributions are introduced, which effectively mitigate the differences between adjacent actions and ensuring smoothness in the learned policies. Subsequently, a safe and smooth reinforcement learning algorithm is proposed for training navigation policies, and its superiority in terms of safety and smoothness are validated by using a ground mobile robot in a simulated environment.
MoreTranslated text
Key words
Collision Avoidance, Reinforcement Learning, Safety, Smoothness
AI Read Science
Must-Reading Tree
Example
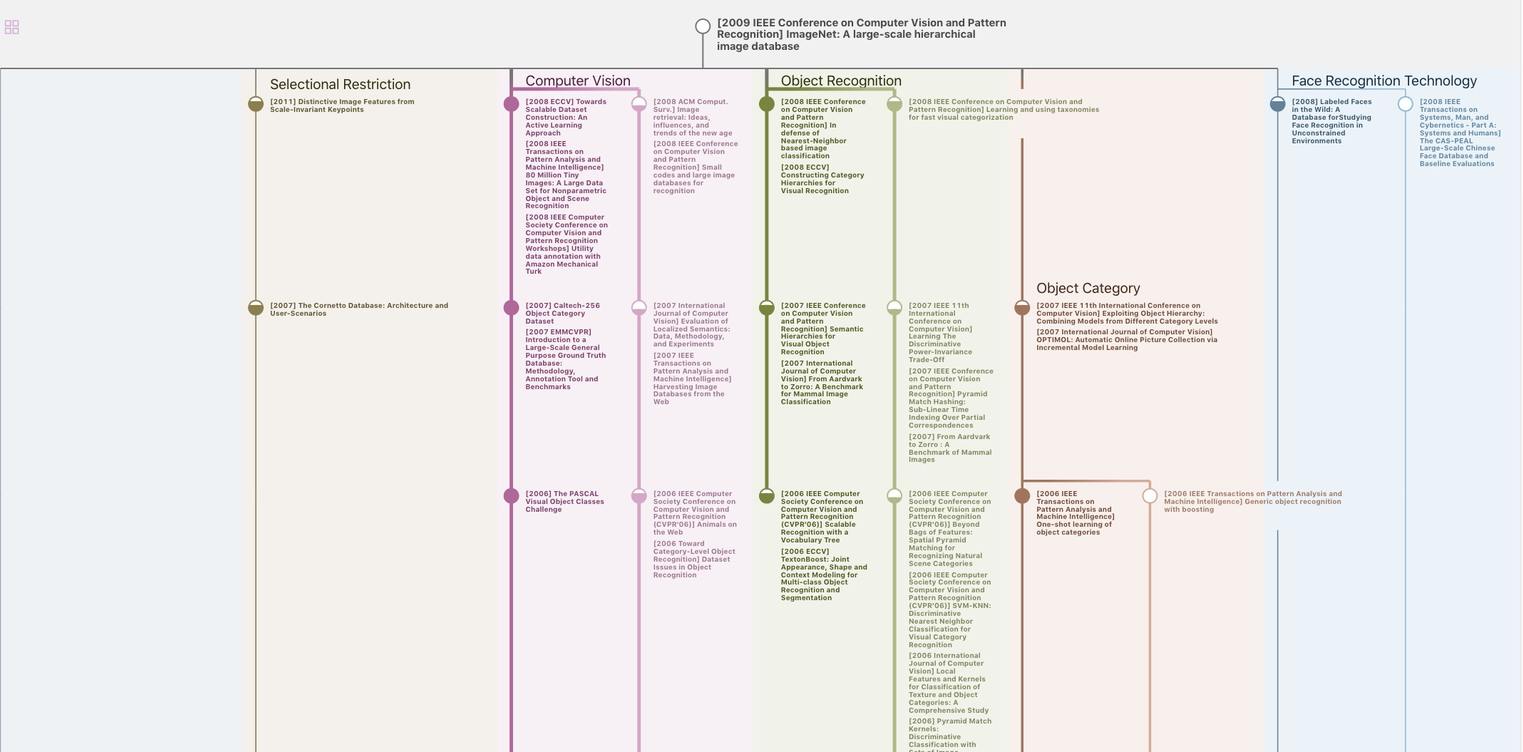
Generate MRT to find the research sequence of this paper
Chat Paper
Summary is being generated by the instructions you defined