Multi-SP Network Slicing Parallel Relieving Edge Network Conflict
IEEE Transactions on Parallel and Distributed Systems(2023)
Abstract
Network slicing is rapidly prevailing in the edge network, which provides computing, network, and storage resources for various services. When the multiple service providers (SPs) respond to their tenants in parallel, individual decisions on the dynamic and shared edge network may lead to resource conflicts, which affects the delivery of network slicing services. Existing works ignore resource interaction and coordination in the multi-SP scenario, which is not in line with the actual situation. Indeed, the complexity of resource interaction caused by the coexistence of multiple SP policies increases the difficulty to solve the formulated optimization model. In this article, we focus on the multi-SP network slicing deployment in parallel. The coordination of network resources between SPs is designed as an effective multi-agent communication mechanism that is merged into multi-agent deep reinforcement learning (MADRL). To deal with dynamic edge networks, we design the neurons hotplugging learning which realizes scalability without a high cost of model retraining. Experiments on real and random networks demonstrate that the proposed multi-SP network slicing mechanism can successfully learn coordination policies and easily adapt to various network scales. It improves the accepted requests by 7.4%, reduces resource conflicts by 14.5%, and shortens the model convergence time by 83.3%.
MoreTranslated text
Key words
Network slicing,Resource management,Neurons,Scalability,Privacy,Network topology,Adaptation models,resource conflict,MADRL,multi-agent communication,neurons hotplugging learning
AI Read Science
Must-Reading Tree
Example
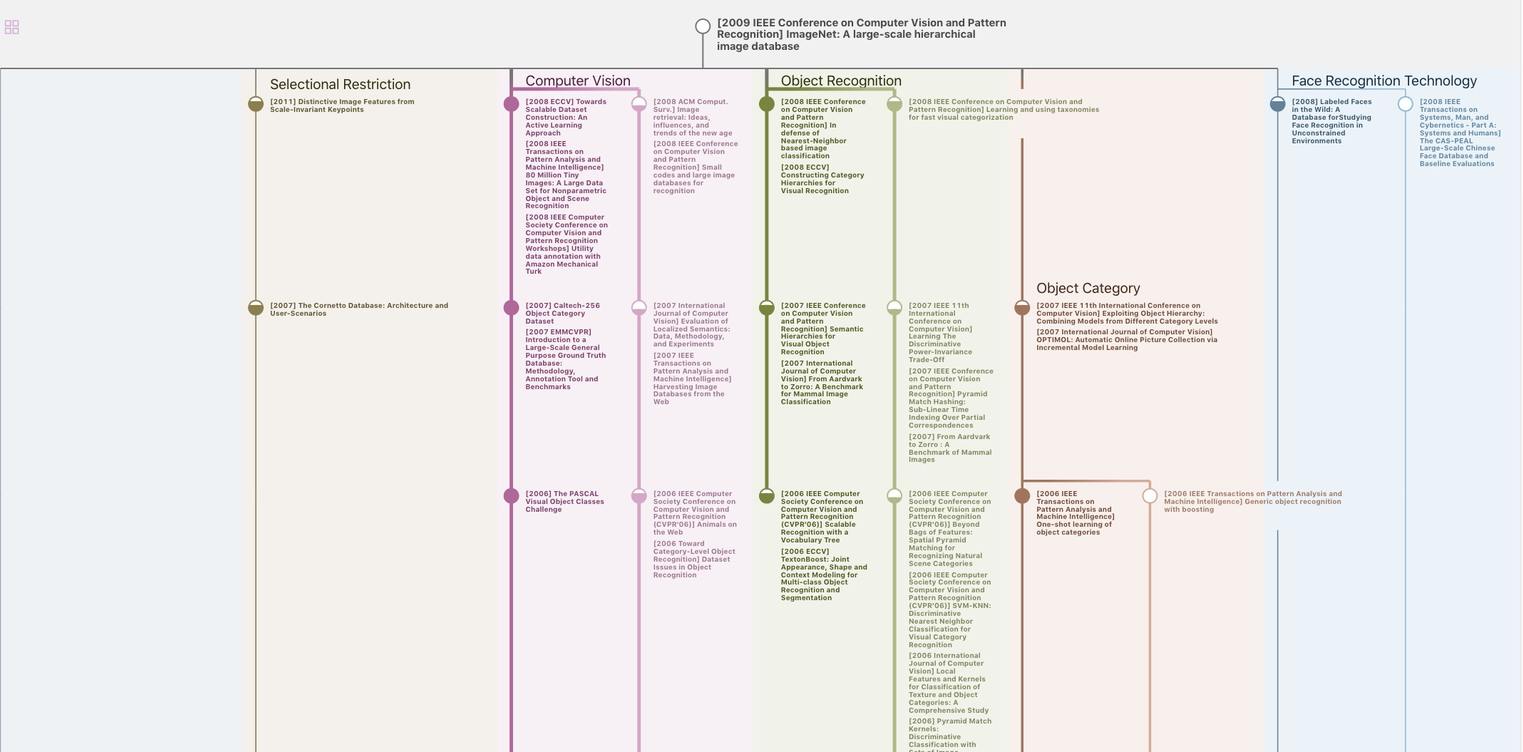
Generate MRT to find the research sequence of this paper
Chat Paper
Summary is being generated by the instructions you defined