A point crowding-degree based evolutionary algorithm for many-objective optimization
Memetic Comput.(2023)
摘要
It is well known that many-objective optimization problems (MaOPs) are difficultly to be balanced diversity and convergence in the search process because diversity and convergence contradict each other. The method of evaluating the diversity of the solution set can directly affect the final performances of the algorithms. In this article, a point crowding-degree (PC) strategy is proposed to evaluate the diversity of solutions. The proposed PC strategy not only considers the distance between any two points as large as possible, but also considers the gap between each dimension component as large as possible. Moreover, The PC only ponders the influence of surrounding neighbor points on the diversity of a point. A selection strategy is designed to balance convergence and diversity. Based on all, a point crowding-degree based evolutionary algorithm (PCEA) for many-objective optimization problems is proposed. The PCEA is compared experimentally with several state-of-the-art algorithms on the 57 many-objective benchmark functions and the experimental results show that the proposed PCEA algorithm has strong competitiveness and better overall performance. In addition, the proposed PC strategy is integrated into other advanced MaOPs methods. The results show that it is beneficial to improve the performance of other MaOEAs algorithms.
更多查看译文
关键词
Many-objective optimization,Point crowding-degree,Diversity,Evolutionary algorithm
AI 理解论文
溯源树
样例
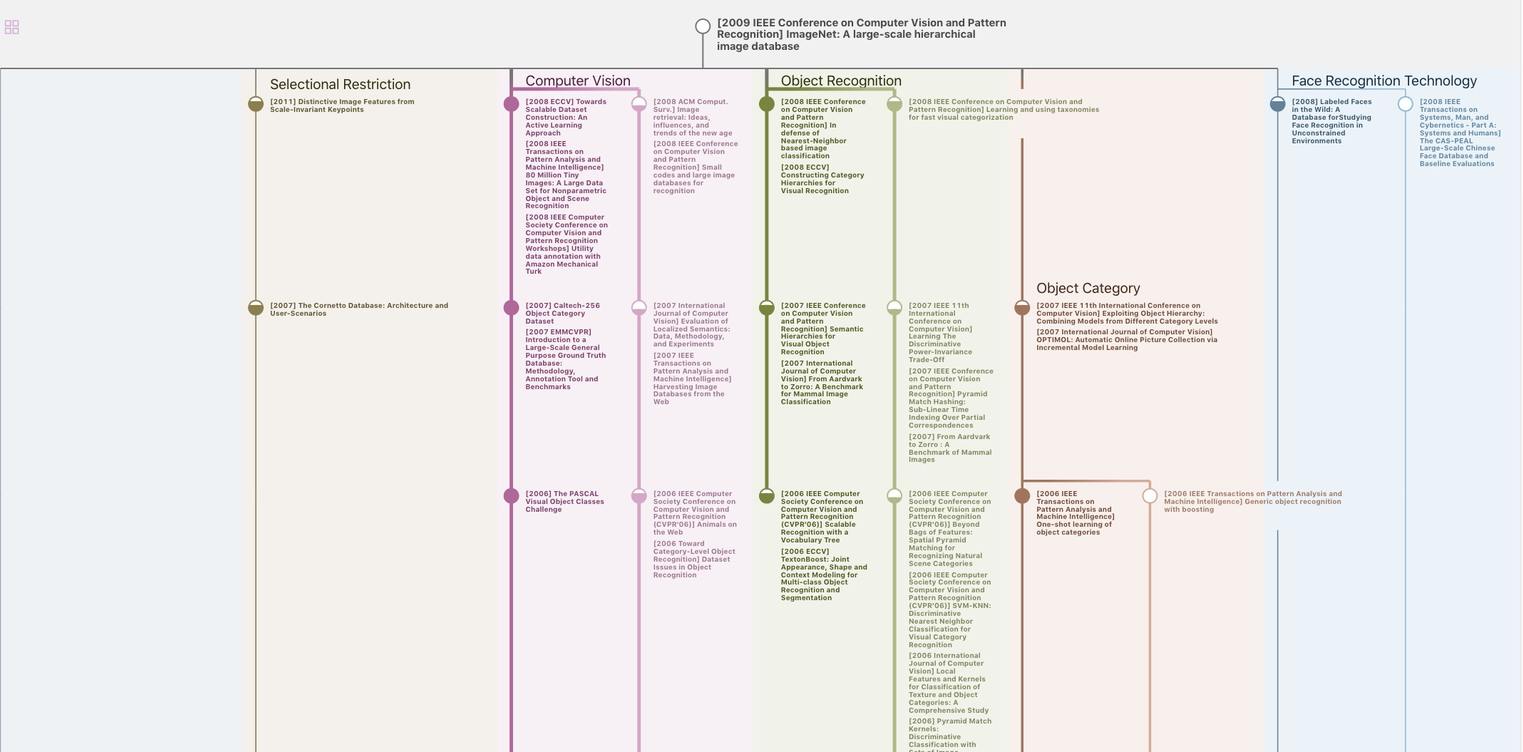
生成溯源树,研究论文发展脉络
Chat Paper
正在生成论文摘要