Collaborative region-boundary interaction network for medical image segmentation
Multimedia Tools and Applications(2024)
摘要
Existing medical image segmentation methods achieve impressive progress but remain challenged by high diversity in region scales or capricious boundaries. Meanwhile, they usually ignore another favorable factor, i.e., correlations between region and boundary, resulting in limitations to performance improvement. In this paper, we propose a novel framework, aiming at accurate segmentation by collaborating region detection and boundary localization subtasks as well as interacting relations between them. In particular, we first put forward a novel multi-level adaptive feature learning module, which can select discriminative information from backbone features by constructing holistic adaptive weights. Then, a collaborative multi-step refinement module is designed to excavate the reciprocal benefits between two subtasks via reasoning their high correlations cues. Moreover, we propose parallel iterative decoders, i.e., region/boundary iterative decoder, each of which consists of attention-based dual iteration paths to effectively aggregate multi-scale features. By cooperating with these three creative parts, our method sets the new state-of-the-art segmentation performance on five polyp benchmarks, where it achieves a mean Dice score of 92.9% on CVC-ClinicDB dataset. Furthermore, we extensively evaluate our method on both skin lesion segmentation from ISIC 2018 and nuclei segmentation from 2018 Data Science Bowl dataset, demonstrating its excellent generalization ability.
更多查看译文
关键词
Medical image segmentation,Multi-level adaptive feature learning,Collaborative interaction,Multi-step refinement,Iterative manner
AI 理解论文
溯源树
样例
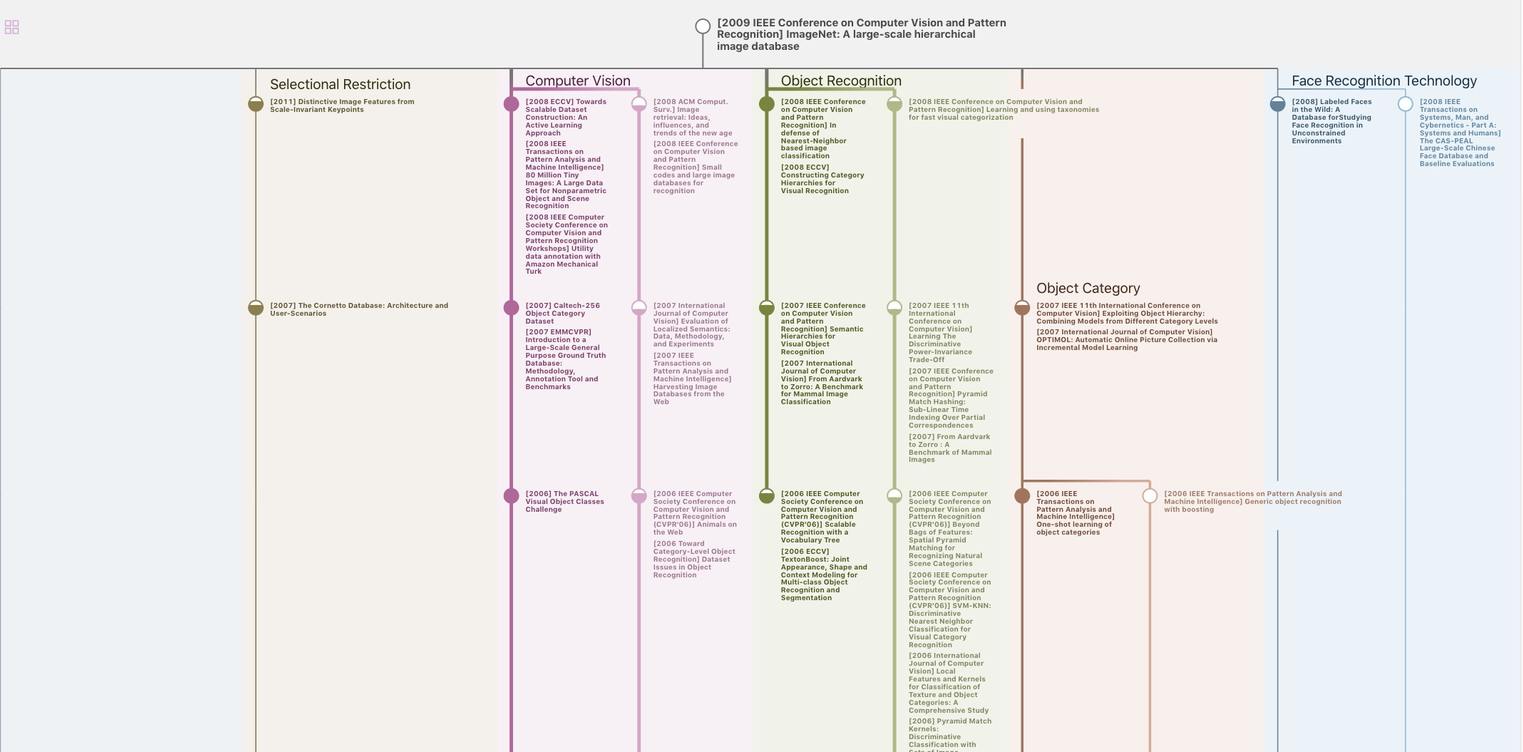
生成溯源树,研究论文发展脉络
Chat Paper
正在生成论文摘要