Local and global timeseries proxies using functional principal component analysis: application to history-matching and uncertainty quantification
Computational Geosciences(2023)
摘要
Accurate surrogate models are essential for the application of computational methods such as Markov chain Monte Carlo (McMC) using numerical reservoir simulation. Previous studies have often focused on building surrogates to represent the misfit (or likelihood) function. However, building an accurate constrained surrogate for the likelihood/misfit is difficult for higher dimensions unless an overly large number of samples is simulated first. Fortunately, functional data analysis can provide a set of ensemble-based statistical tools which can be utilized to emulate the full simulation output (timeseries) rather than the misfit function itself. Consequently, the misfit can be easily calculated by the simulated timeseries. In this study, functional principal component analysis (fPCA) is utilized to reduce the dimensionality of the timeseries. In other words, each simulation output (e.g., oil rate) is represented in terms of a few optimal functional principal component scores (fPCS), which can be readily inverted to reconstruct the original timeseries. fPCA is employed herein to develop a new and efficient Bayesian history-matching workflow in that it is used to iteratively update the fPCA-based local timeseries surrogates and search for the extrema of the likelihood function. In this proposed process, a few initial random samples are generated, and the corresponding timeseries are processed by fPCA. The resulting fPCSs for each simulation output are modelled individually using random variable proxies such as kriging or random forest to relate the uncertain variables to projected scores. These proxies are then used to calculate the likelihood and optimized to suggest the next best samples until a convergence criterion is met. This workflow is applied to a set of data obtained from a near-critical gas condensate well from a Canadian shale reservoir. The proposed history-matching workflow results in a nearly 8-times faster average convergence rate compared to other population-based algorithms studied. The history-matching samples are combined with additional adaptive samples to enhance the exploration aspect and predictability of the local surrogate models. This leads to an improvement of the local timeseries proxies for different outputs (from history-matching) and helps to generate global timeseries proxies that can be used for the entire parameter space. The results demonstrate that clustering the timeseries and applying fPCA to each cluster separately can significantly improve the accuracy of the global surrogates. Finally, the global surrogates are utilized to obtain accurate posterior distributions quickly through an McMC algorithm. This study introduces an adaptive sampling method for the first time that can be used to generate a highly accurate surrogate for timeseries and conduct the optimization efficiently.
更多查看译文
关键词
Functional principal component analysis,Markov chain Monte Carlo,Timeseries,Local surrogate,Global surrogate
AI 理解论文
溯源树
样例
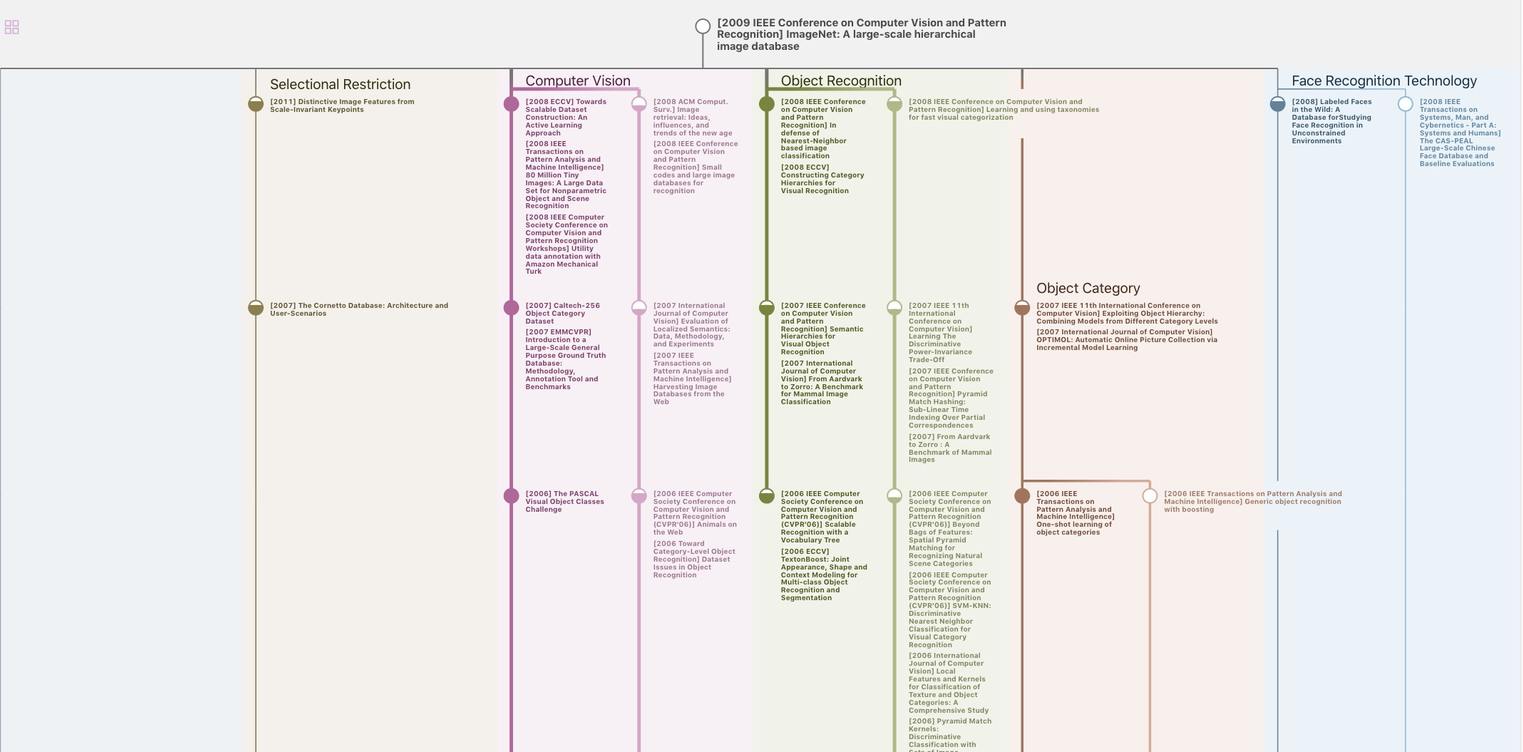
生成溯源树,研究论文发展脉络
Chat Paper
正在生成论文摘要