Prediction of average score per taxon in Japan using mega data from the national census on river environments
Limnology(2024)
摘要
Water is essential in our daily lives, and conserving and monitoring its quality is crucial. Biological assessment is one of the methods for evaluating water quality. This study aimed at predicting the average score per taxon (ASPT), a biological assessment of the water quality index, for 109 Japanese rivers, mainly by machine learning using extreme gradient boosting and random forest. To this end, we extracted and utilized freshwater macroinvertebrate distribution data collected during 1991–2017 under the “National Census on River Environments” (NCRE) by the Ministry of Land, Infrastructure, and Transport. Five factors (average temperature, biochemical oxygen demand, elevation, slope, and human impact index) were addressed as environmental data affecting the macroinvertebrate distribution. Based on the biological data and five factors, predictive models were developed for 71 taxa used for ASPT. To confirm the accuracy of the prediction model, we compared the ASPT values that were predicted to the actual values. The actual values were within the range of the predicted values at elevations below 200 m, and the actual and predicted values diverged at elevations above 200 m, probably because 91% of the biological data used in the model were gathered at elevations lower than 200 m. Thus, the model is applicable at elevations below 200 m. This study is the first to show that it is possible to predict ASPT values for Japanese sites that have not been surveyed. These results suggest that accumulating biological data, such as NCRE data, will enable the preliminary screening of ecological predictions in freshwater areas.
更多查看译文
关键词
Aquatic ecology,Freshwater macroinvertebrate,Biological assessment,Extreme gradient boosting,Random forest
AI 理解论文
溯源树
样例
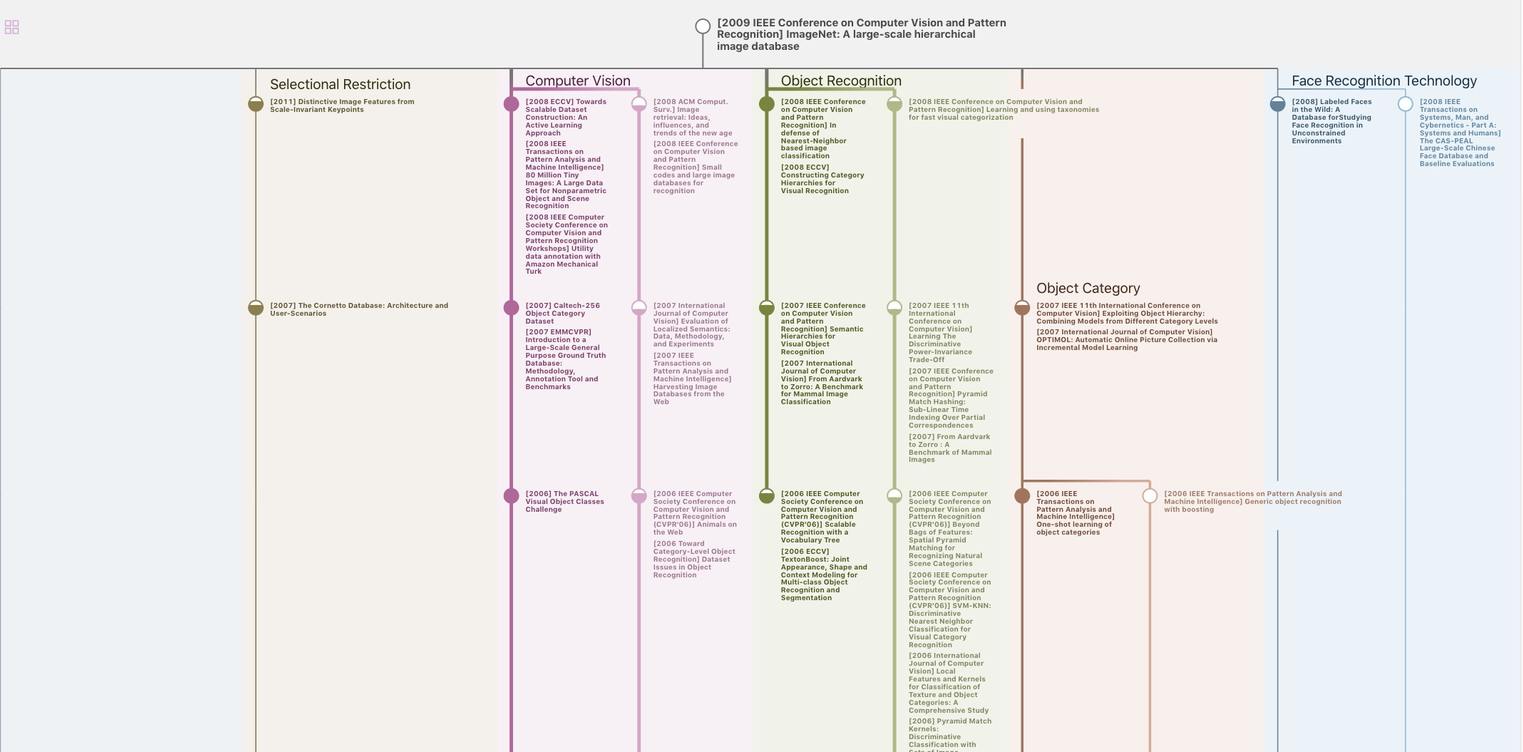
生成溯源树,研究论文发展脉络
Chat Paper
正在生成论文摘要