Context-aware driver risk prediction with telematics data
Accident; analysis and prevention(2023)
摘要
Driving risk prediction is crucial for safety and risk mitigation. While traditional methods rely on demographic information for insurance pricing, they may not fully capture actual driving behavior. To address this, telematics data has gained popularity. This study focuses on using telematics data and contextual information (e.g., road type, daylight) to represent a driver’s style through tensor representations. Drivers with similar behaviors are identified by clustering their representations, forming risk cohorts. Past at-fault traffic accidents and citations serve as partial risk labels. The relative magnitude of average records (per driver) for each cohort indicates their risk label, such as low or high risk, which can be transferred to drivers in a cohort. A classifier is then constructed using augmented risk labels and driving style representations to predict driving risk for new drivers. Real-world data from major US cities validates the effectiveness of this framework. The approach is practical for large-scale scenarios as the data can be obtained at scale. Its focus on driver-based risk prediction makes it applicable to industries like auto-insurance. Beyond personalized premiums, the framework empowers drivers to assess their driving behavior in various contexts, facilitating skill improvement over time.
更多查看译文
关键词
Telematics data,Contextual information,Label refinement,Contextualization,Risk classification
AI 理解论文
溯源树
样例
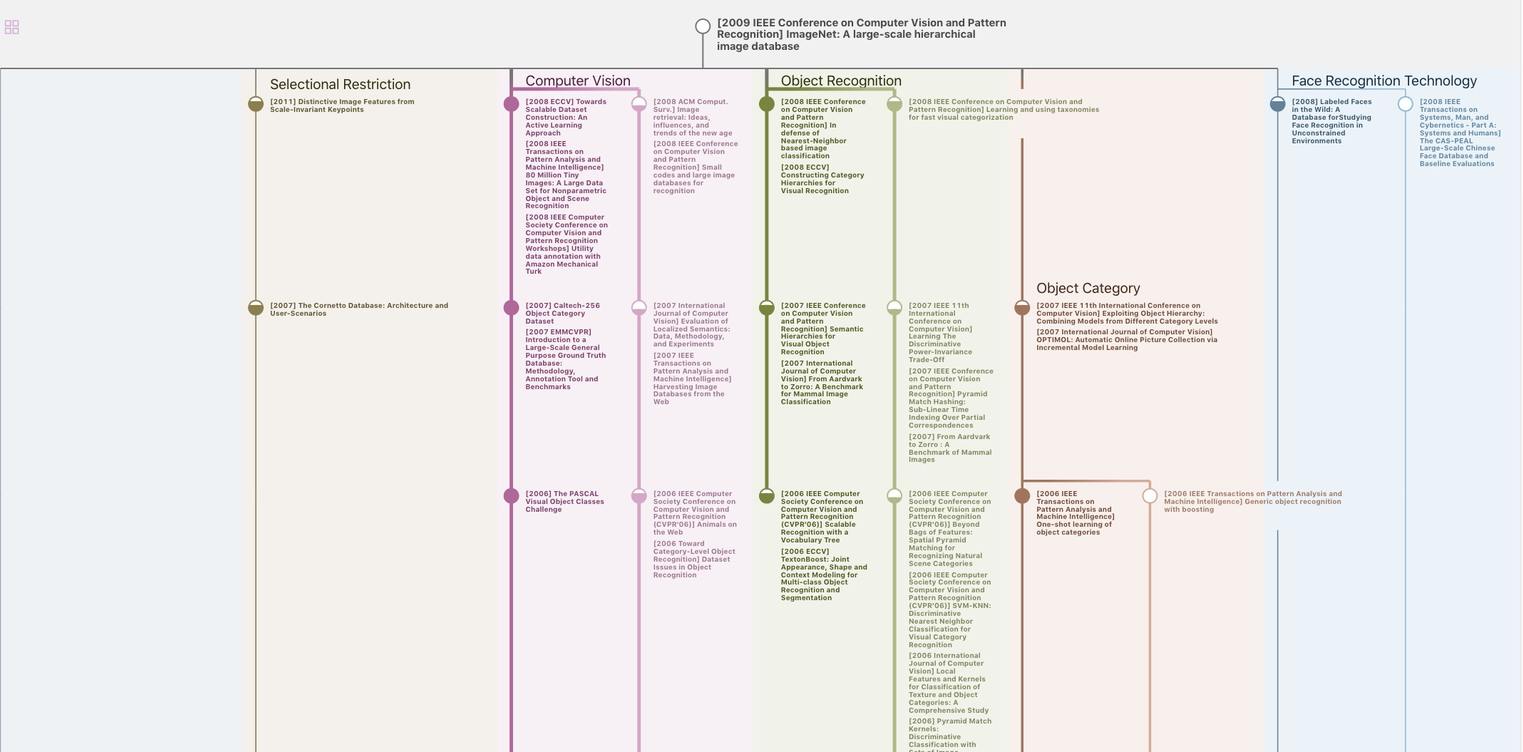
生成溯源树,研究论文发展脉络
Chat Paper
正在生成论文摘要