Improving and Evaluating the Detection of Fragmentation in News Recommendations with the Clustering of News Story Chains
CoRR(2023)
摘要
News recommender systems play an increasingly influential role in shaping information access within democratic societies. However, tailoring recommendations to users' specific interests can result in the divergence of information streams. Fragmented access to information poses challenges to the integrity of the public sphere, thereby influencing democracy and public discourse. The Fragmentation metric quantifies the degree of fragmentation of information streams in news recommendations. Accurate measurement of this metric requires the application of Natural Language Processing (NLP) to identify distinct news events, stories, or timelines. This paper presents an extensive investigation of various approaches for quantifying Fragmentation in news recommendations. These approaches are evaluated both intrinsically, by measuring performance on news story clustering, and extrinsically, by assessing the Fragmentation scores of different simulated news recommender scenarios. Our findings demonstrate that agglomerative hierarchical clustering coupled with SentenceBERT text representation is substantially better at detecting Fragmentation than earlier implementations. Additionally, the analysis of simulated scenarios yields valuable insights and recommendations for stakeholders concerning the measurement and interpretation of Fragmentation.
更多查看译文
关键词
fragmentation,clustering,news
AI 理解论文
溯源树
样例
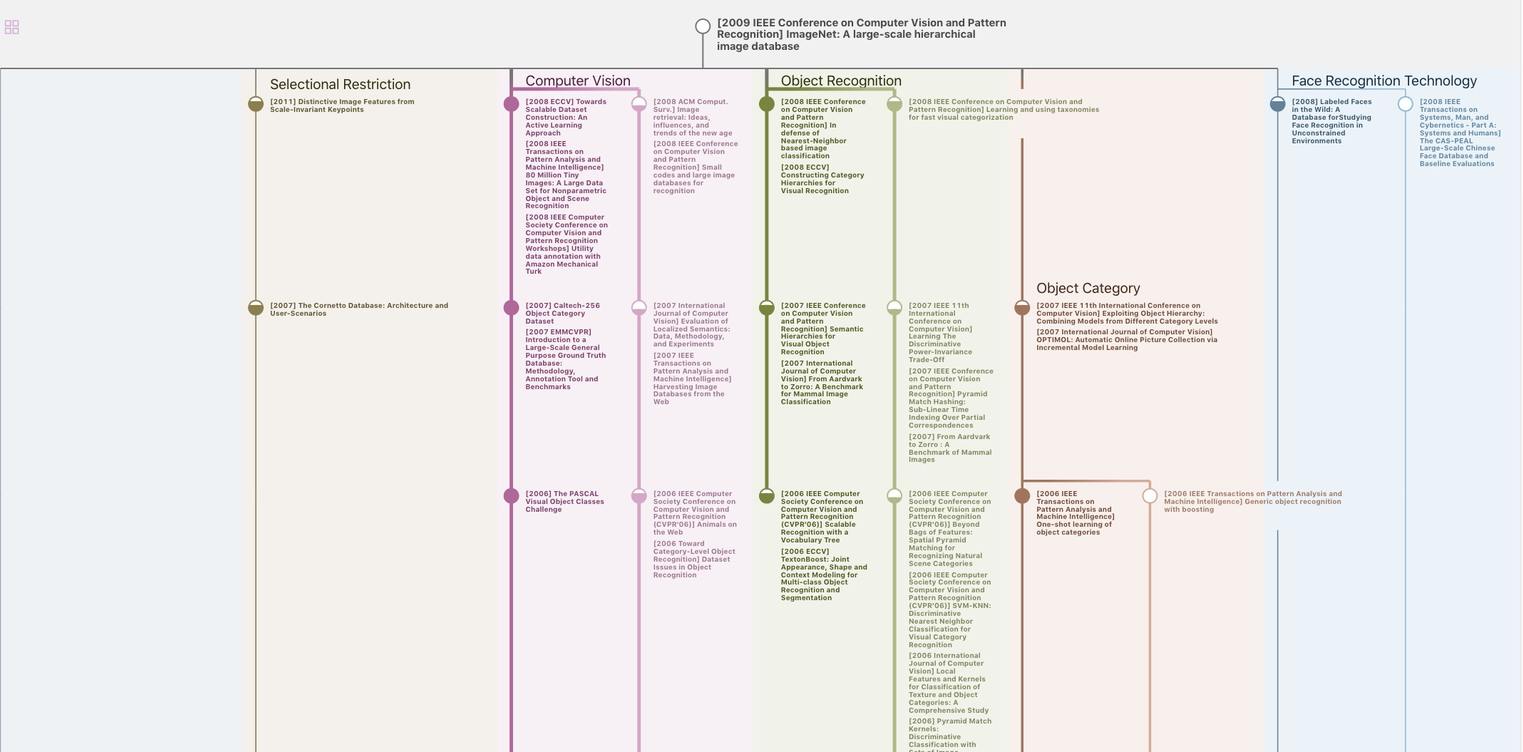
生成溯源树,研究论文发展脉络
Chat Paper
正在生成论文摘要