Improving generalization capability of deep learning-based nuclei instance segmentation by non-deterministic train time and deterministic test time stain normalization
COMPUTATIONAL AND STRUCTURAL BIOTECHNOLOGY JOURNAL(2024)
摘要
With the advent of digital pathology and microscopic systems that can scan and save whole slide histological images automatically, there is a growing trend to use computerized methods to analyze acquired images. Among different histopathological image analysis tasks, nuclei instance segmentation plays a fundamental role in a wide range of clinical and research applications. While many semi- and fully-automatic computerized methods have been proposed for nuclei instance segmentation, deep learning (DL)-based approaches have been shown to deliver the best performances. However, the performance of such approaches usually degrades when tested on unseen datasets. In this work, we propose a novel method to improve the generalization capability of a DL-based automatic segmentation approach. Besides utilizing one of the state-of-the-art DL-based models as a baseline, our method incorporates non-deterministic train time and deterministic test time stain normalization, and ensembling to boost the segmentation performance. We trained the model with one single training set and evaluated its segmentation performance on seven test datasets. Our results show that the proposed method provides up to 4.9%, 5.4%, and 5.9% better average performance in segmenting nuclei based on Dice score, aggregated Jaccard index, and panoptic quality score, respectively, compared to the baseline segmentation model.
更多查看译文
关键词
Digital pathology,Normalization,Nuclei segmentation,Machine learning,Deep learning,Medical image analysis
AI 理解论文
溯源树
样例
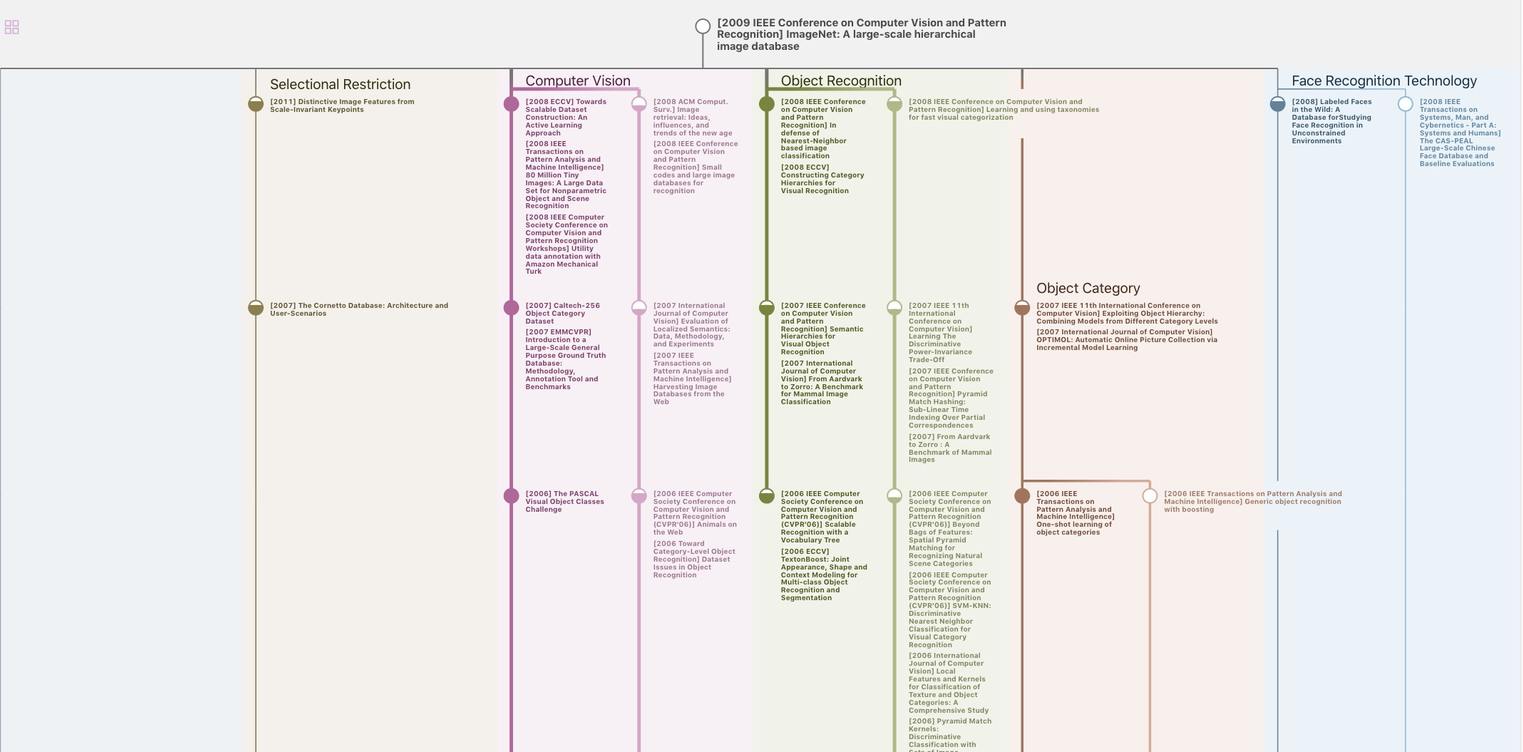
生成溯源树,研究论文发展脉络
Chat Paper
正在生成论文摘要