Predicting interloper fraction with graph neural networks
JOURNAL OF COSMOLOGY AND ASTROPARTICLE PHYSICS(2023)
摘要
Upcoming emission-line spectroscopic surveys, such as Euclid and the Roman Space Telescope, will be affected by systematic effects due to the presence of interlopers: galaxies whose redshift and distance from us are miscalculated due to line confusion in their emission spectra. Particularly pernicious are interlopers involving the confusion between two lines with close emitted wavelengths, like H beta emitters confused as [O iii], since those are strongly spatially correlated with the target galaxies. They introduce a particular pattern in the 3D distribution of the observed galaxy catalog that can shift the position of the BAO peak in the galaxy correlation function and bias any cosmological analysis performed with that sample. Here we present a novel method to predict the fraction of interlopers in a galaxy catalog, using Graph Neural Networks (GNNs) to learn the posterior distribution of the interloper fraction while marginalizing over cosmology and galaxy bias. The method is developed using simulations with halos acting as a proxy for galaxies. The GNN can infer the mean and standard deviation of the posterior distribution of interloper fraction using small-scale information that is usually not considered in cosmological analyses. The injection of large-scale information into the graph as a global attribute improves the performance of the GNN when marginalizing over cosmology.
更多查看译文
关键词
Machine learning,redshift surveys
AI 理解论文
溯源树
样例
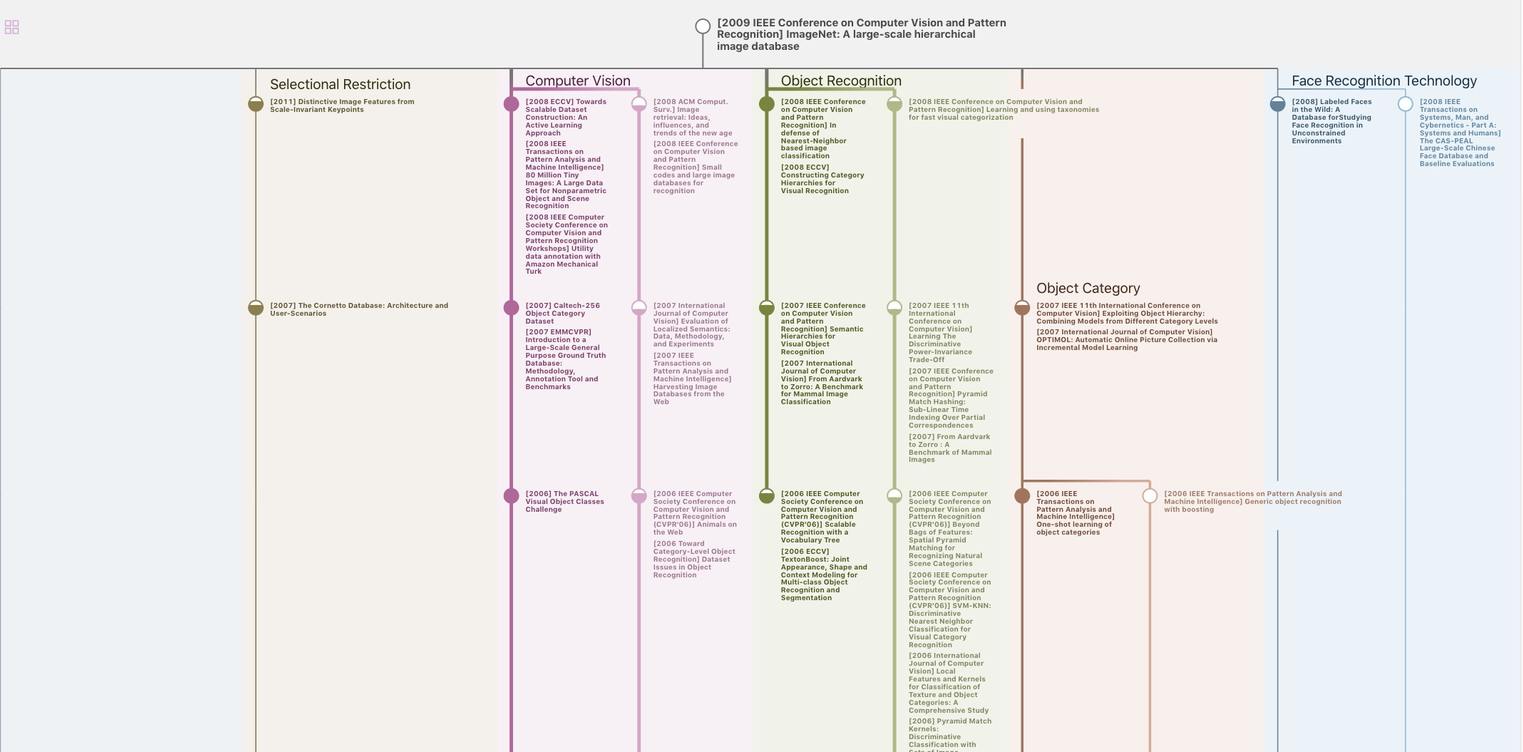
生成溯源树,研究论文发展脉络
Chat Paper
正在生成论文摘要