Hqretouch: learning professional face retouching via masked feature fusion and semantic-aware modulation
2023 IEEE INTERNATIONAL CONFERENCE ON IMAGE PROCESSING, ICIP(2023)
Abstract
Face retouching is a crucial technique for many consumer-level products. The goal of face retouching is to remove skin imperfections and preserve facial details simultaneously. However, it usually requires tedious manual work to achieve professional retouching effect. With the advent of Deep Neural Networks (DNNs), some methods were recently proposed to complete the task of face retouching automatically by using DNNs. They divide a portrait photo into local patches and train a DNN for face retouching. Although they can produce professional results automatically, there are still some limitations. Firstly, the network architecture fails to preserve sufficient facial details. Secondly, the facial semantic information is ignored when dividing a photo into some local patches. In this paper, we propose a novel method to solve these limitations. We first introduce the Masked Feature Fusion (MFF) module to a UNet, enabling the network to better preserve details in facial regions. Then, we exploit the semantic information by the Semantic-Aware Modulation (SAM) module, further boosting the retouching performance. Experiments on the recent public dataset Flickr-Faces-HQ-Retouched (FFHQR) demonstrate the effectiveness of our method. The code will be released.
MoreTranslated text
Key words
Professional Face Retouching,Masked Feature Fusion,Semantic-Aware Modulation
AI Read Science
Must-Reading Tree
Example
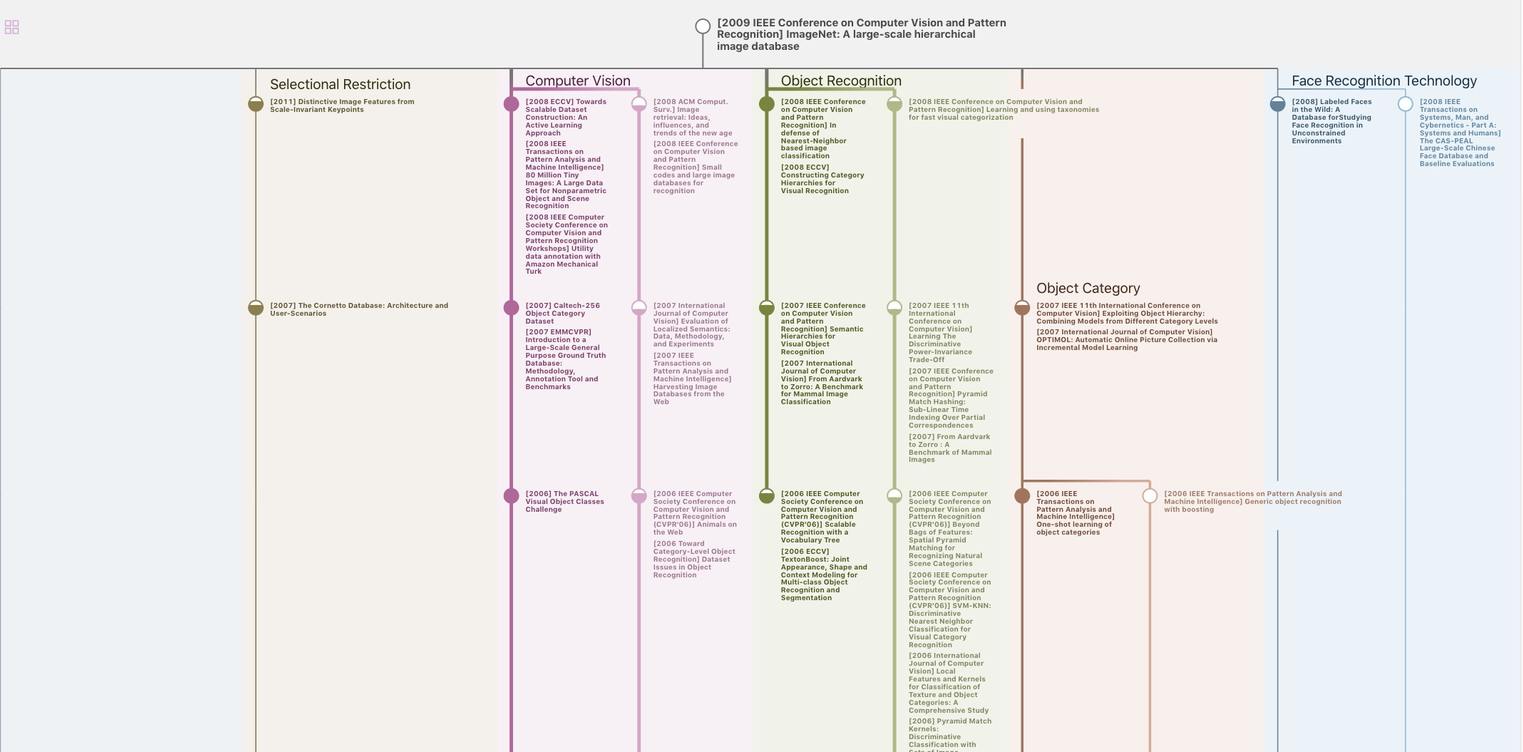
Generate MRT to find the research sequence of this paper
Chat Paper
Summary is being generated by the instructions you defined