Dual temporal transformers for fine-grained dangerous action recognition
2023 IEEE INTERNATIONAL CONFERENCE ON IMAGE PROCESSING, ICIP(2023)
Abstract
Recognizing dangerous actions is a critical task in computer vision, especially for surveillance applications. While existing deep learning methods have been successful in confined environments, they struggle with the anomalous and salient variations of human postures in dangerous actions. Additionally, finer-grained dangerous actions require more discriminative cues, adding to the complexity of the task. To address these challenges, we propose a novel solution that models the intrinsic and invariant properties of dangerous actions at multiple temporal semantic levels. Concretely, we propose a Dual Temporal Transformers (DTT) to capture temporal interactions between distinct key points in the human body aggregation from shallow to deep layers, increasing the perception field from local to global, simultaneously. By doing so, our method avoids overfitting to unrelated or minor clues in videos and achieves a generalized representation of abnormal actions. We evaluate our approach on indoor and outdoor environments and found that DTT outperforms existing methods in terms of efficiency and accuracy. Our code and dataset are pubic available on https://github.com/AveryJohnsonJJ/DTT.git.
MoreTranslated text
Key words
Fine-grained Dangerous Action Recognition,Temporal Transformer,Action Recognition
AI Read Science
Must-Reading Tree
Example
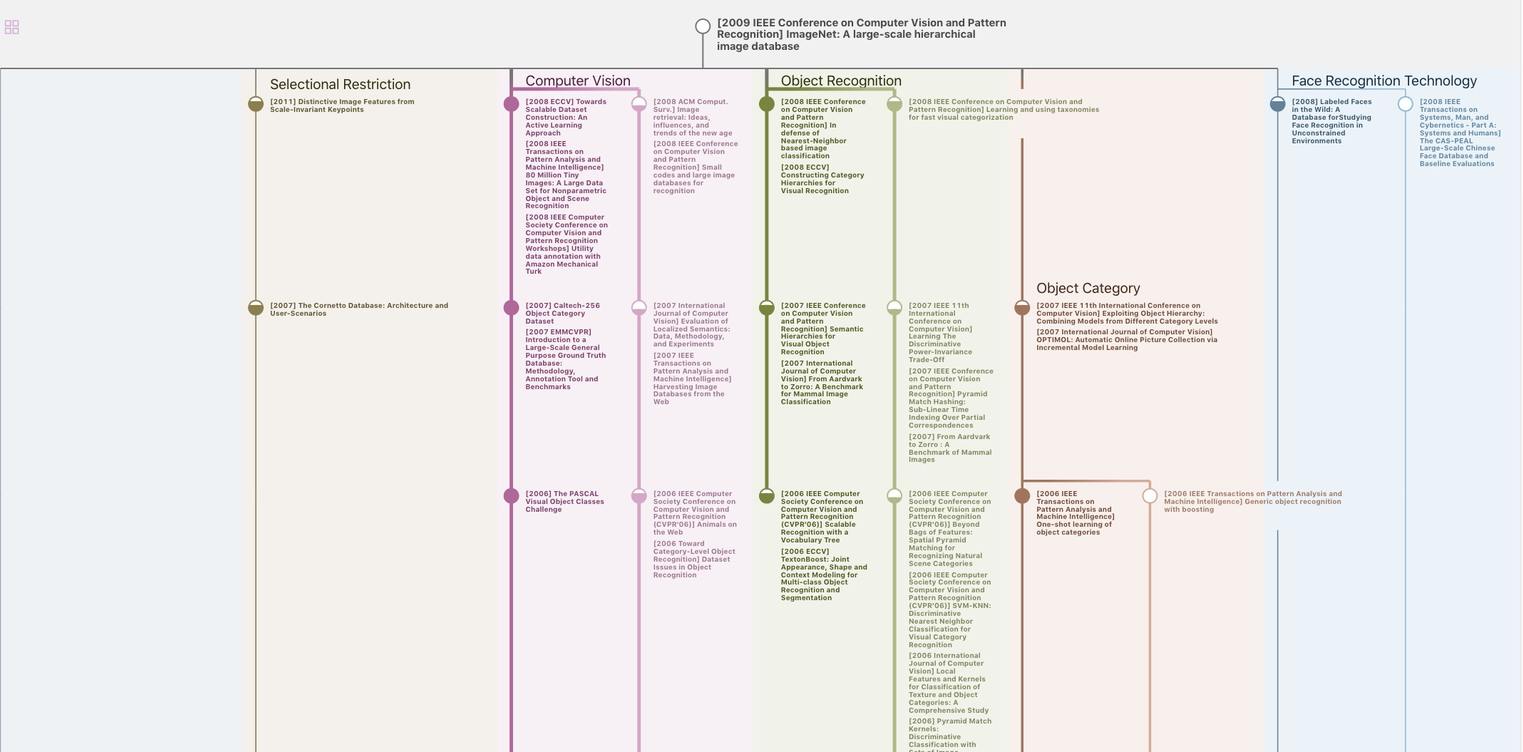
Generate MRT to find the research sequence of this paper
Chat Paper
Summary is being generated by the instructions you defined