Machine Learning Techniques for Data-Driven Computer-Aided Diagnostic Method of Polycystic Ovary Syndrome (PCOS) resulting from Functional Ovarian Hyperandrogenism (FOH)
2023 International Conference on Computational Intelligence and Sustainable Engineering Solutions (CISES)(2023)
摘要
Due to changes in their way of life and the environment, humans have recently faced a wide variety of ailments. PCOS. Female infertility in India is primarily caused by PCOS, where it affects one in five (20%) of the population. The causes are high levels of androgens, a male hormone. Menstrual cycles become irregular when follicles, which are tiny fluid-filled sacs made by the ovaries, fail to release eggs on a regular basis. Doctors find it tough to manually examine disease symptoms, but machine learning techniques could be used to ensure that this category effectively identifies people with chronic conditions. Early detection and diagnosis of some diseases are more important since doing so can prevent them from progressing to their worst state. Methods: In this work, a diagnostic and timely prevention system will be developed that can diagnose PCOS using different machine learning techniques: support vector machines (SVM), logistic regression, and random forests. A correlation-based feature selection method is used to pick the features based on statistical values. The newly developed CAD system was tested using Prasoon Kottarathil's PCOS dataset, which was purchased from Kaggle. In conclusion, SVM obtains an accuracy of 70.55% for the PCOS dataset, 90.18% for logistic regression, and 92.024% for random forest. Gynecologists might seek a second opinion using the recognised CAD system to identify PCOS.
更多查看译文
关键词
Random Forest,Support Vector Machine,Logistic Regression,Correlation,Polycystic Ovary Syndrome
AI 理解论文
溯源树
样例
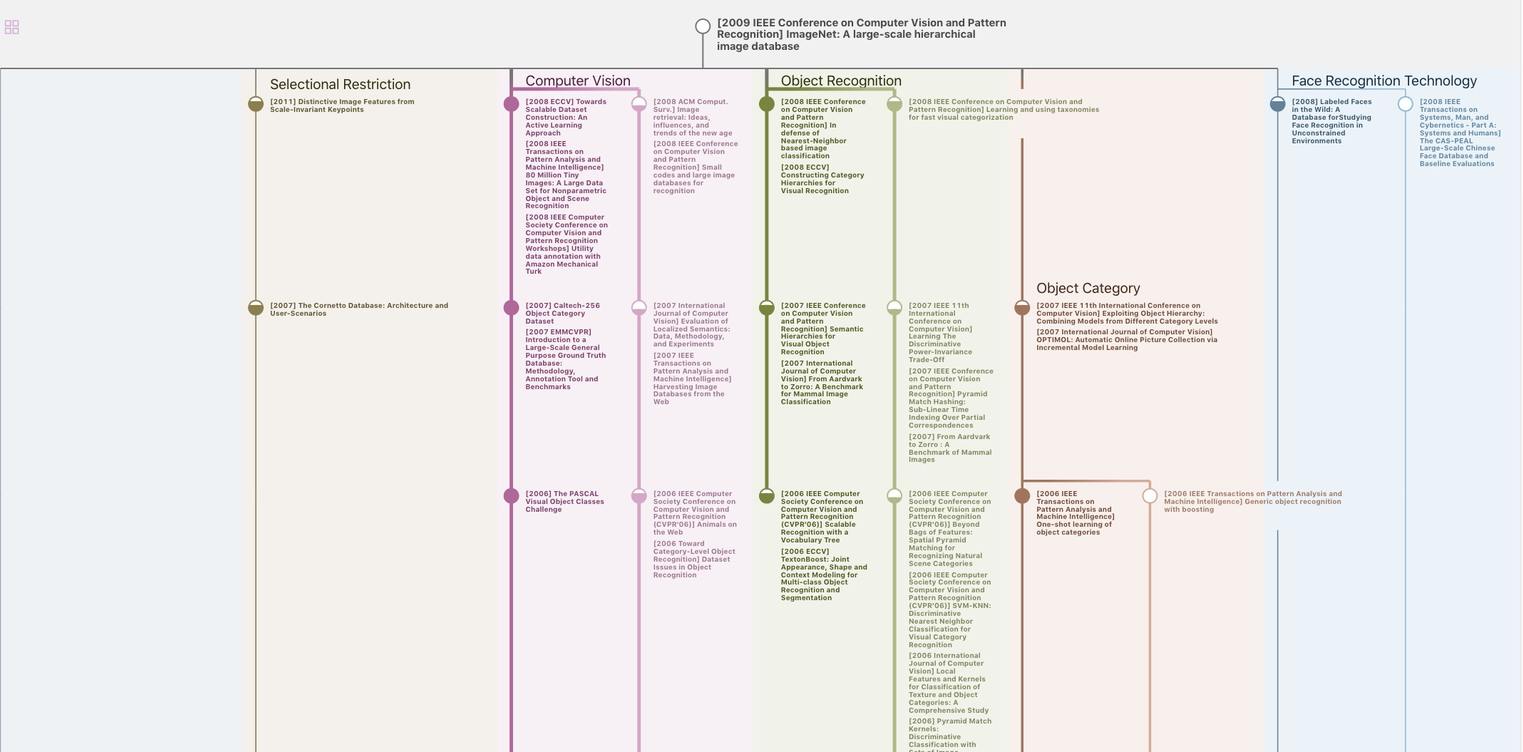
生成溯源树,研究论文发展脉络
Chat Paper
正在生成论文摘要