Automatic Regenerative Simulation Via Non-Reversible Simulated Tempering
JOURNAL OF THE AMERICAN STATISTICAL ASSOCIATION(2024)
关键词
Adaptation,Asymptotic variance,Distributed simulation,Markov chain Monte Carlo,Parallelization
AI 理解论文
溯源树
样例
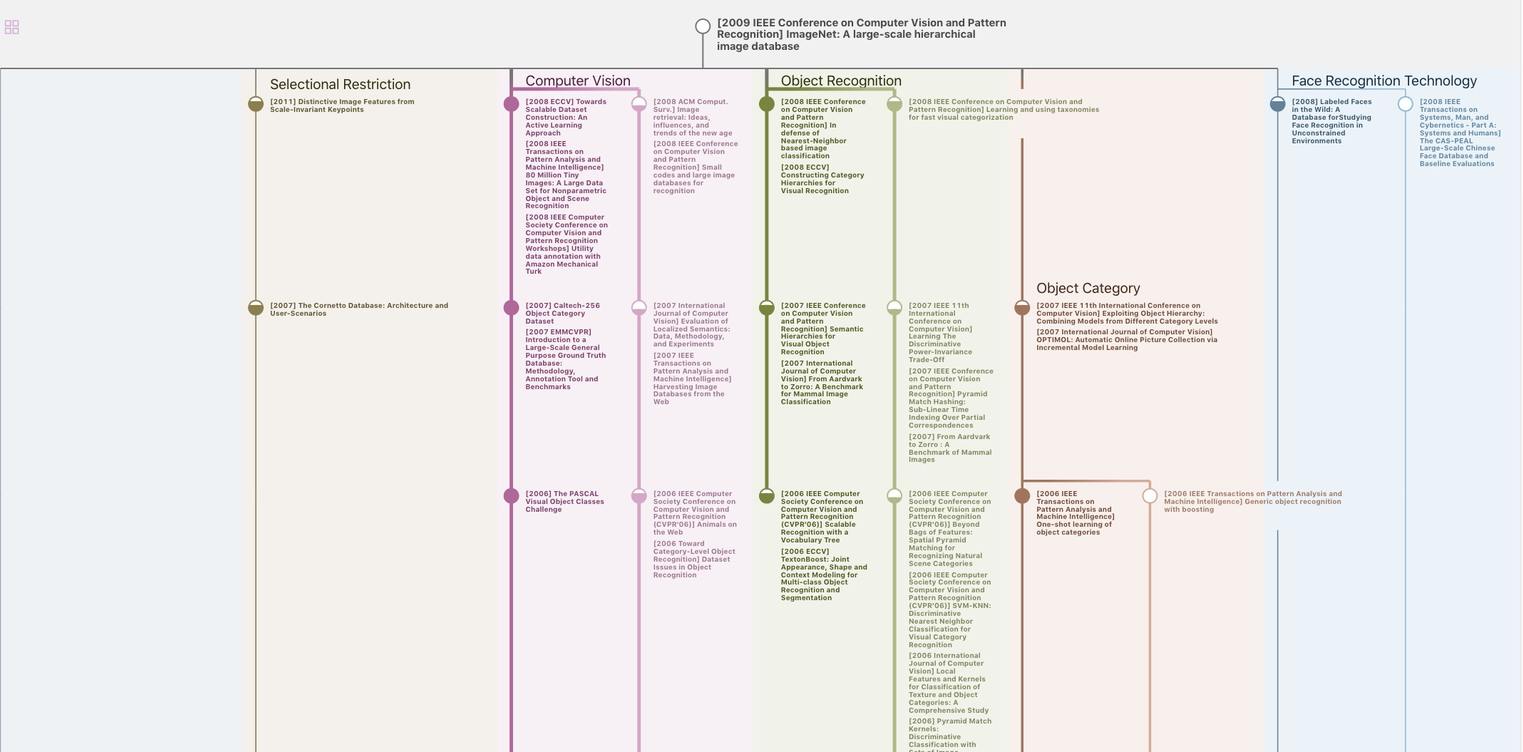
生成溯源树,研究论文发展脉络
Chat Paper
正在生成论文摘要